Modified RNN for Solving Comprehensive Sylvester Equation with TDOA Application
IEEE TRANSACTIONS ON NEURAL NETWORKS AND LEARNING SYSTEMS(2023)
摘要
The augmented Sylvester equation, as a comprehensive equation, is of great significance and its special cases (e.g., Lyapunov equation, Sylvester equation, Stein equation) are frequently encountered in various fields. It is worth pointing out that the current research on simultaneously eliminating the lagging error and handling noises in the nonstationary complex-valued field is rather rare. Therefore, this article focuses on solving a nonstationary complex-valued augmented Sylvester equation (NCASE) in real time and proposes two modified recurrent neural network (RNN) models. The first proposed modified RNN model possesses gradient search and velocity compensation, termed as RNN-GV model. The superiority of the proposed RNN-GV model to traditional algorithms including the complex-valued gradient-based RNN (GRNN) model lies in completely eliminating the lagging error when employed in the nonstationary problem. The second model named complex-valued integration enhanced RNN-GV with the nonlinear acceleration (IERNN-GVN) model is proposed to adapt to a noisy environment and accelerate the convergence process. Besides, the convergence and robustness of these two proposed models are proved via theoretical analysis. Simulative results on an illustrative example and an application to the moving source localization coincide with the theoretical analysis and illustrate the excellent performance of the proposed models.
更多查看译文
关键词
Gradient search,integration enhancement,nonlinear acceleration,nonstationary complex-valued augmented Sylvester equation (NCASE),recurrent neural network (RNN),velocity compensation
AI 理解论文
溯源树
样例
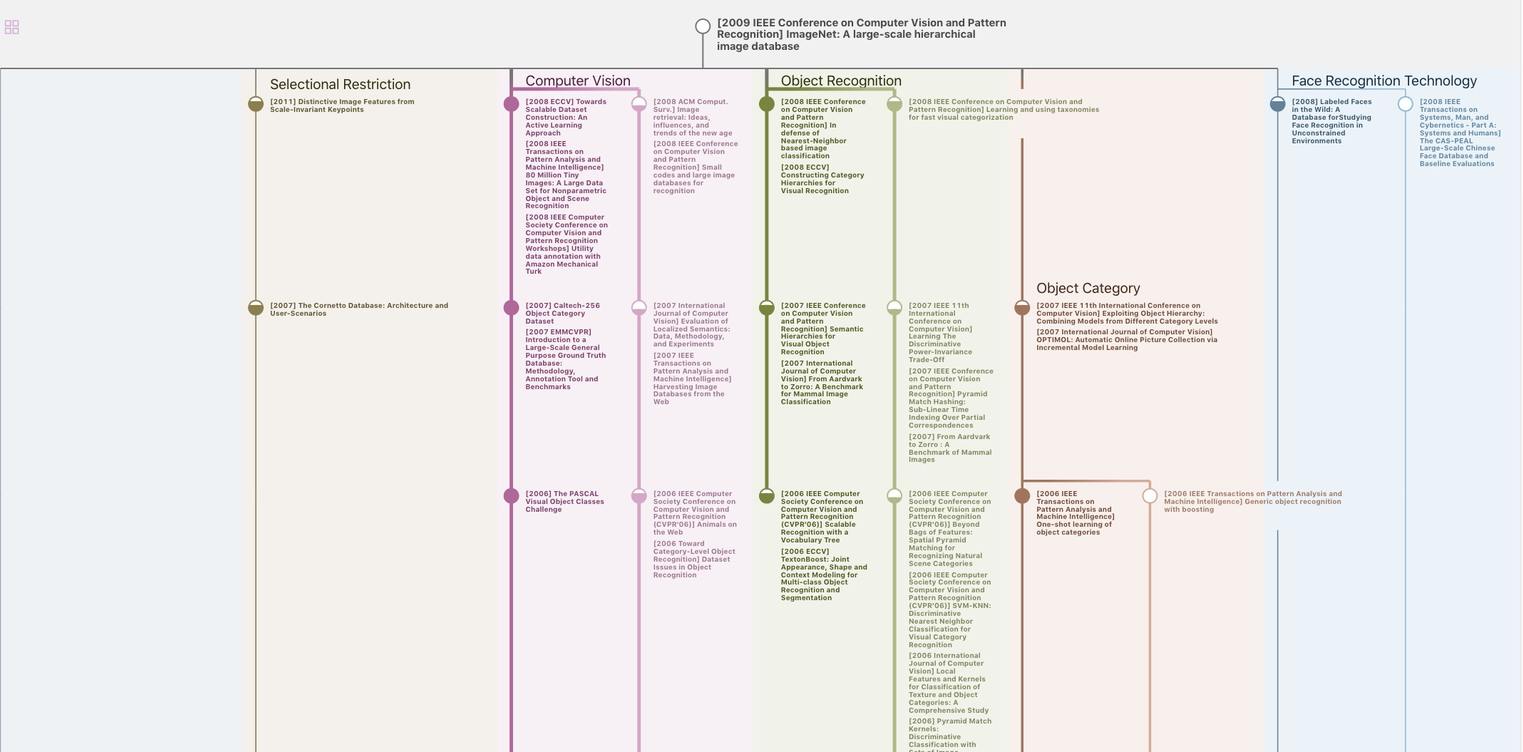
生成溯源树,研究论文发展脉络
Chat Paper
正在生成论文摘要