Benchmarking Self-Supervised Contrastive Learning Methods for Image-Based Plant Phenotyping.
Plant phenomics (Washington, D.C.)(2023)
摘要
The rise of self-supervised learning (SSL) methods in recent years presents an opportunity to leverage unlabeled and domain-specific datasets generated by image-based plant phenotyping platforms to accelerate plant breeding programs. Despite the surge of research on SSL, there has been a scarcity of research exploring the applications of SSL to image-based plant phenotyping tasks, particularly detection and counting tasks. We address this gap by benchmarking the performance of 2 SSL methods-momentum contrast (MoCo) v2 and dense contrastive learning (DenseCL)-against the conventional supervised learning method when transferring learned representations to 4 downstream (target) image-based plant phenotyping tasks: wheat head detection, plant instance detection, wheat spikelet counting, and leaf counting. We studied the effects of the domain of the pretraining (source) dataset on the downstream performance and the influence of redundancy in the pretraining dataset on the quality of learned representations. We also analyzed the similarity of the internal representations learned via the different pretraining methods. We find that supervised pretraining generally outperforms self-supervised pretraining and show that MoCo v2 and DenseCL learn different high-level representations compared to the supervised method. We also find that using a diverse source dataset in the same domain as or a similar domain to the target dataset maximizes performance in the downstream task. Finally, our results show that SSL methods may be more sensitive to redundancy in the pretraining dataset than the supervised pretraining method. We hope that this benchmark/evaluation study will guide practitioners in developing better SSL methods for image-based plant phenotyping.
更多查看译文
关键词
plant,contrastive learning methods,self-supervised,image-based
AI 理解论文
溯源树
样例
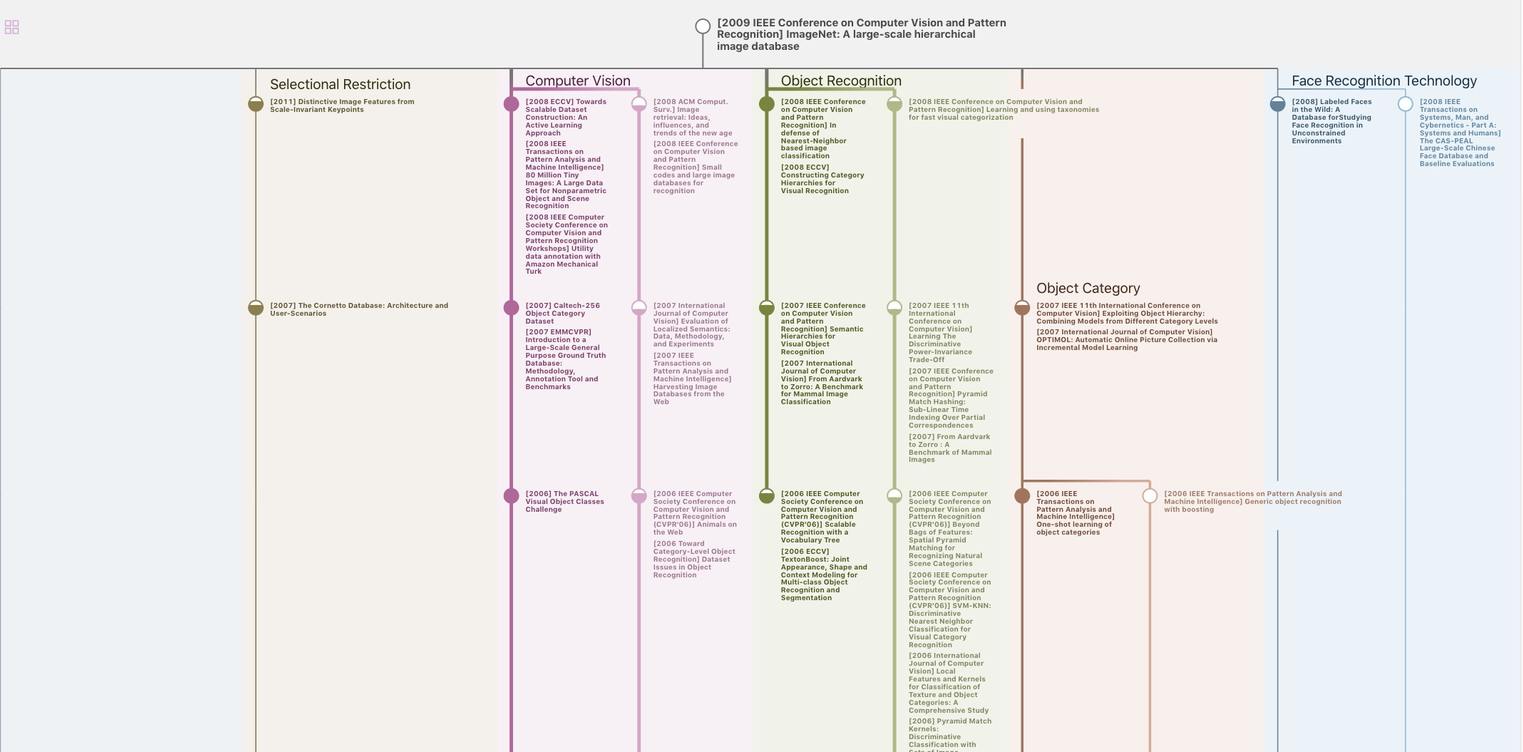
生成溯源树,研究论文发展脉络
Chat Paper
正在生成论文摘要