Three-Dimensional Human Pose Estimation from Sparse IMUs through Temporal Encoder and Regression Decoder
Sensors(2023)
摘要
Three-dimensional (3D) pose estimation has been widely used in many three-dimensional human motion analysis applications, where inertia-based path estimation is gradually being adopted. Systems based on commercial inertial measurement units (IMUs) usually rely on dense and complex wearable sensors and time-consuming calibration, causing intrusions to the subject and hindering free body movement. The sparse IMUs-based method has drawn research attention recently. Existing sparse IMUs-based three-dimensional pose estimation methods use neural networks to obtain human poses from temporal feature information. However, these methods still suffer from issues, such as body shaking, body tilt, and movement ambiguity. This paper presents an approach to improve three-dimensional human pose estimation by fusing temporal and spatial features. Based on a multistage encoder–decoder network, a temporal convolutional encoder and human kinematics regression decoder were designed. The final three-dimensional pose was predicted from the temporal feature information and human kinematic feature information. Extensive experiments were conducted on two benchmark datasets for three-dimensional human pose estimation. Compared to state-of-the-art methods, the mean per joint position error was decreased by 13.6% and 19.4% on the total capture and DIP-IMU datasets, respectively. The quantitative comparison demonstrates that the proposed temporal information and human kinematic topology can improve pose accuracy.
更多查看译文
关键词
three-dimensional human pose,sparse IMUs,encoder–decoder,temporal convolutional encoder,human kinematics hierarchy,regression decoder
AI 理解论文
溯源树
样例
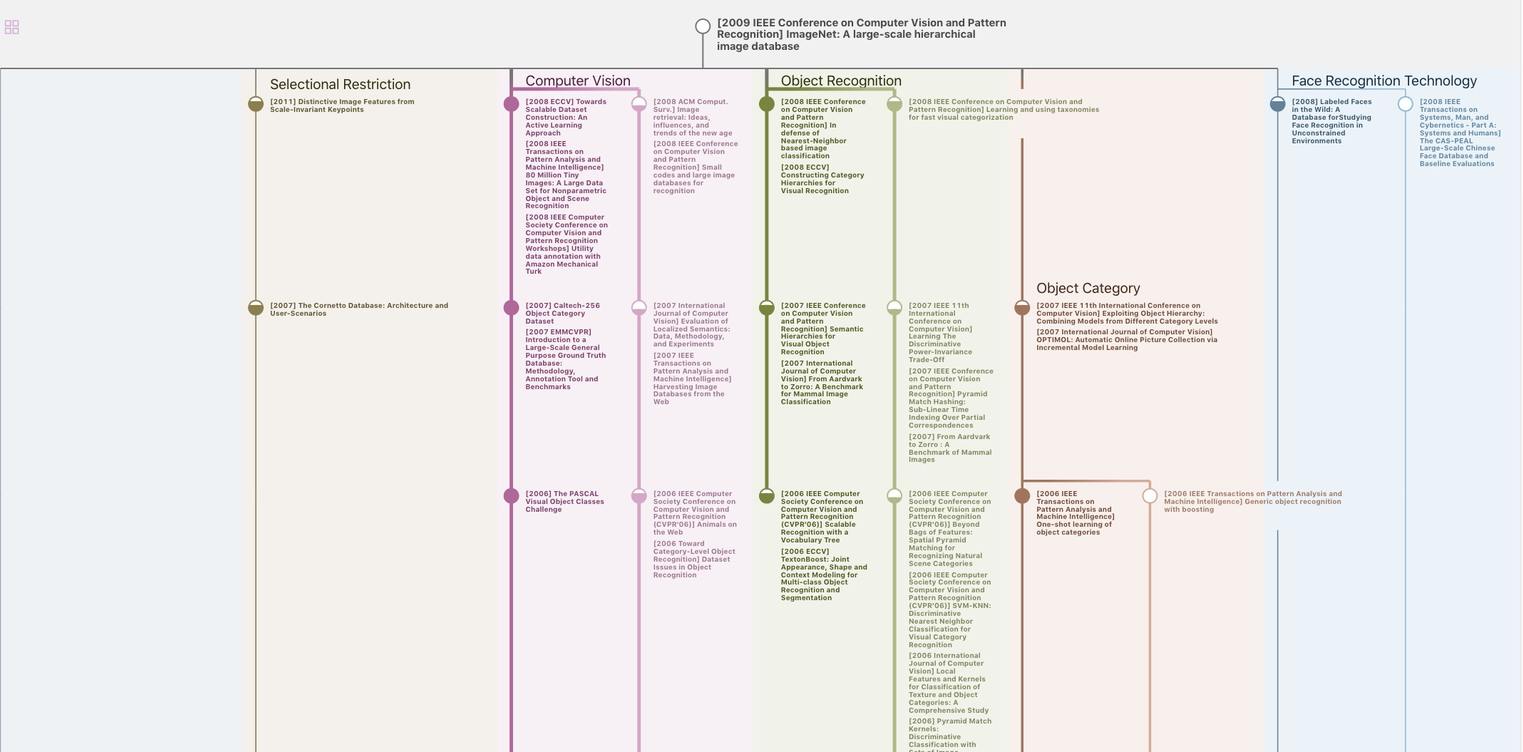
生成溯源树,研究论文发展脉络
Chat Paper
正在生成论文摘要