Deep Metric Learning for Scalable Gait-Based Person Re-Identification Using Force Platform Data
Sensors(2023)
摘要
Walking gait data acquired with force platforms may be used for person re-identification (re-ID) in various authentication, surveillance, and forensics applications. Current force platform-based re-ID systems classify a fixed set of identities (IDs), which presents a problem when IDs are added or removed from the database. We formulated force platform-based re-ID as a deep metric learning (DML) task, whereby a deep neural network learns a feature representation that can be compared between inputs using a distance metric. The force platform dataset used in this study is one of the largest and the most comprehensive of its kind, containing 193 IDs with significant variations in clothing, footwear, walking speed, and time between trials. Several DML model architectures were evaluated in a challenging setting where none of the IDs were seen during training (i.e., zero-shot re-ID) and there was only one prior sample per ID to compare with each query sample. The best architecture was 85% accurate in this setting, though an analysis of changes in walking speed and footwear between measurement instances revealed that accuracy was 28% higher on same-speed, same-footwear comparisons, compared to cross-speed, cross-footwear comparisons. These results demonstrate the potential of DML algorithms for zero-shot re-ID using force platform data, and highlight challenging cases.
更多查看译文
关键词
gait recognition,biometric,ground reaction force,deep learning,zero-shot re-ID,center of pressure
AI 理解论文
溯源树
样例
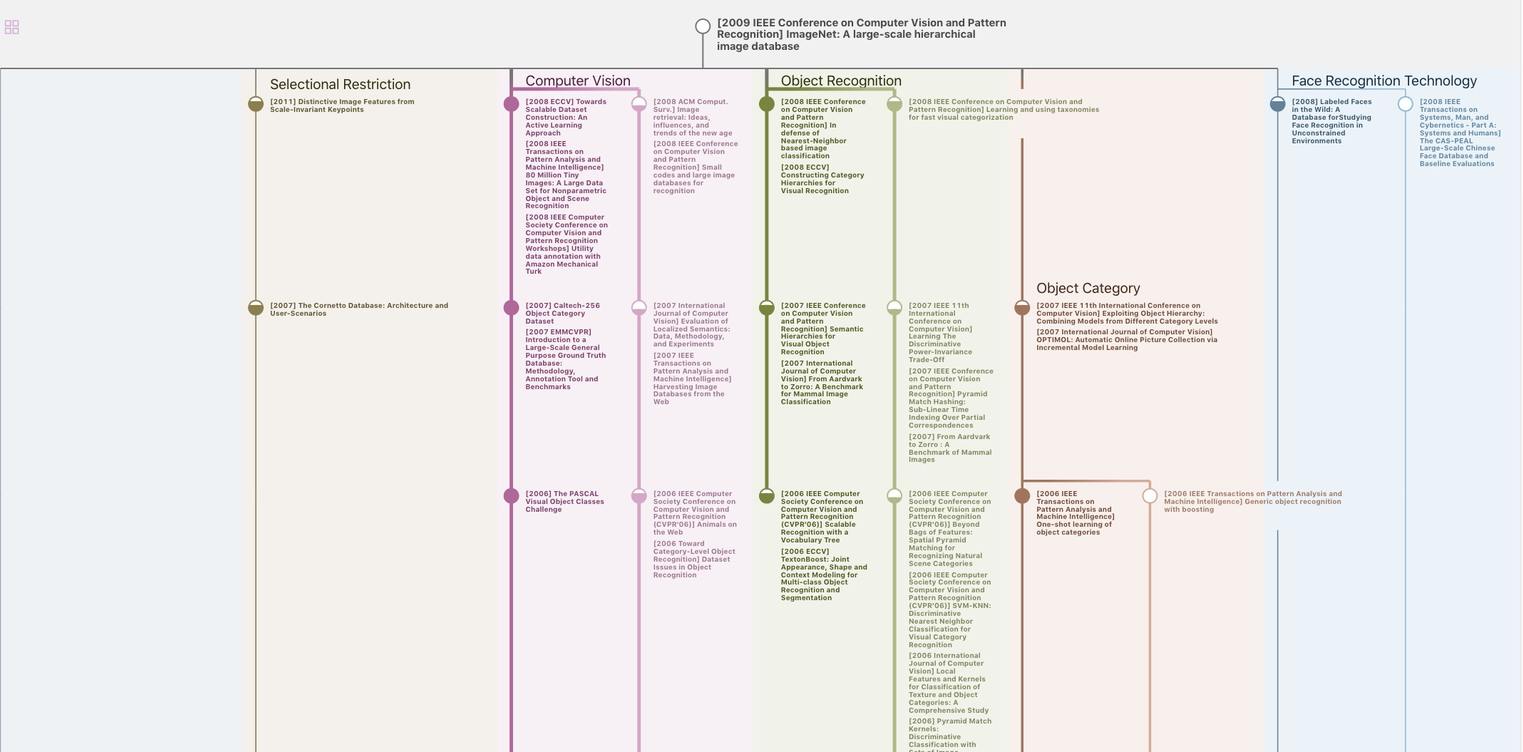
生成溯源树,研究论文发展脉络
Chat Paper
正在生成论文摘要