A New Method for False Alarm Suppression in Heterogeneous Change Detection
Remote Sensing(2023)
摘要
Heterogeneous change detection has a wide range of applications in many fields. However, to date, many existing problems of heterogeneous change detection, such as false alarm suppression, have not been specifically addressed. In this article, we discuss the problem of false alarm suppression and propose a new method based on the combination of a convolutional neural network (CNN) and graph convolutional network (GCN). This approach employs a two-channel CNN to learn the feature maps of multitemporal images and then calculates difference maps of different scales, which means that both low-level and high-level features contribute equally to the change detection. The GCN, with a newly built convolution kernel (called the partially absorbing random walk convolution kernel), classifies these difference maps to obtain the inter-feature relationships between true targets and false ones, which can be represented by an adjacent matrix. We use pseudo-label samples to train the whole network, which means our method is unsupervised. Our method is verified on two typical data sets. The experimental results indicate the superiority of our method compared to some state-of-the-art approaches, which proves the efficacy of our method in false alarm suppression.
更多查看译文
关键词
heterogeneous change detection,CNN,feature difference,GCN
AI 理解论文
溯源树
样例
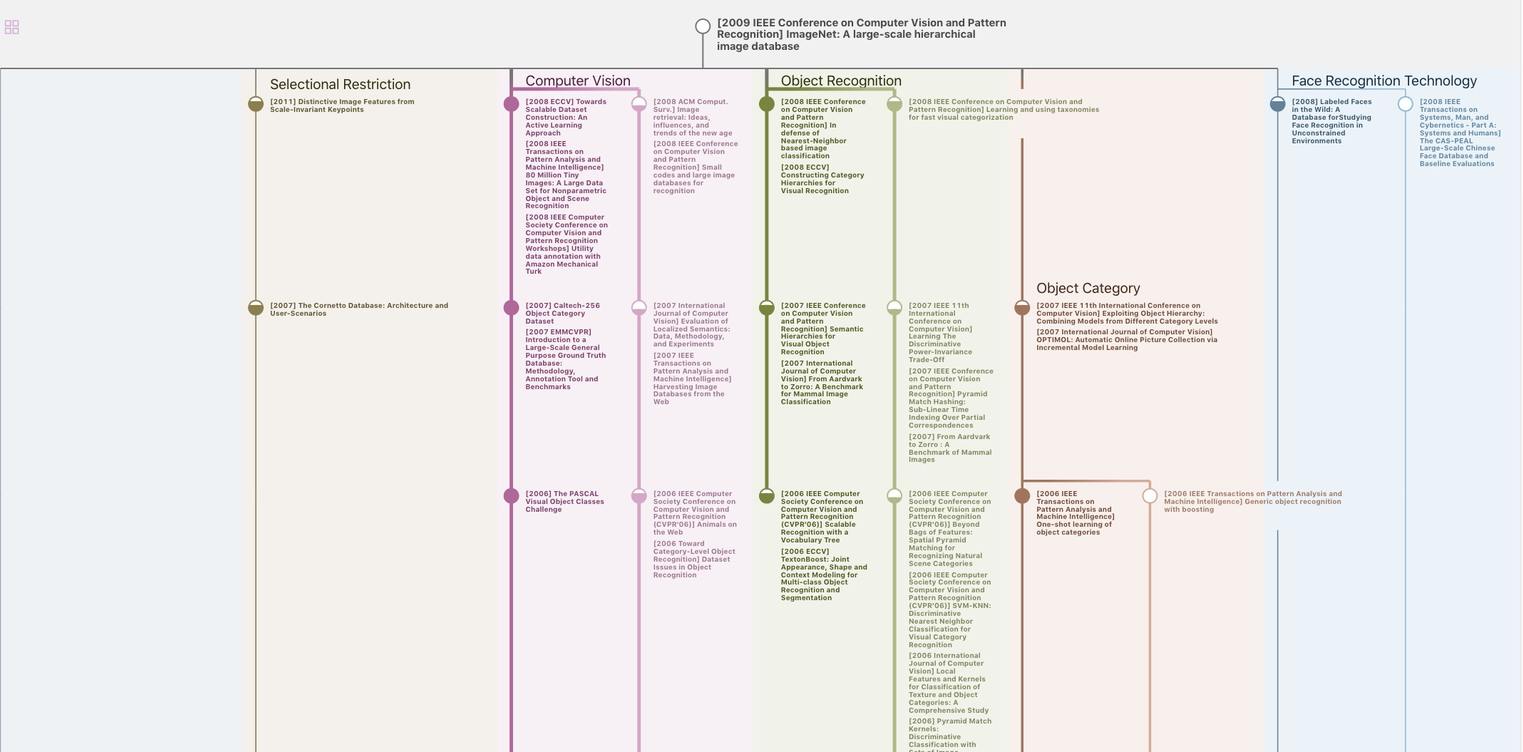
生成溯源树,研究论文发展脉络
Chat Paper
正在生成论文摘要