Strip Surface Defect Detection Algorithm Based on YOLOv5.
MATERIALS(2023)
摘要
In order to improve the detection accuracy of the surface defect detection of industrial hot rolled strip steel, the advanced technology of deep learning is applied to the surface defect detection of strip steel. In this paper, we propose a framework for strip surface defect detection based on a convolutional neural network (CNN). In particular, we propose a novel multi-scale feature fusion module (ATPF) for integrating multi-scale features and adaptively assigning weights to each feature. This module can extract semantic information at different scales more fully. At the same time, based on this module, we build a deep learning network, CG-Net, that is suitable for strip surface defect detection. The test results showed that it achieved an average accuracy of 75.9 percent (mAP50) in 6.5 giga floating-point operation (GFLOPs) and 105 frames per second (FPS). The detection accuracy improved by 6.3% over the baseline YOLOv5s. Compared with YOLOv5s, the reference quantity and calculation amount were reduced by 67% and 59.5%, respectively. At the same time, we also verify that our model exhibits good generalization performance on the NEU-CLS dataset.
更多查看译文
关键词
deep learning,hot rolled strip steel,YOLOv5,attention mechanism,surface defect detection
AI 理解论文
溯源树
样例
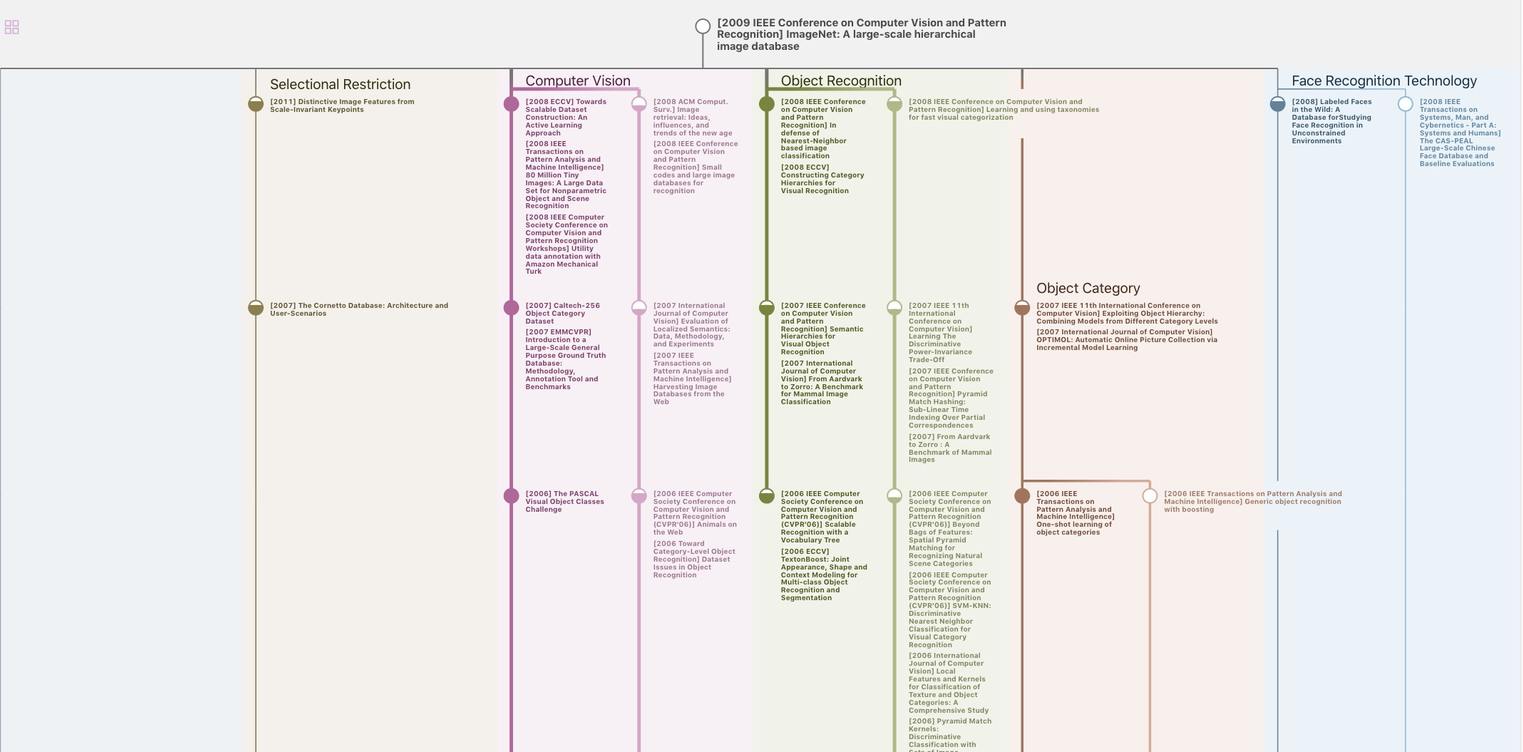
生成溯源树,研究论文发展脉络
Chat Paper
正在生成论文摘要