Error Consistency for Machine Learning Evaluation and Validation with Application to Biomedical Diagnostics
Diagnostics(2023)
摘要
Supervised machine learning classification is the most common example of artificial intelligence (AI) in industry and in academic research. These technologies predict whether a series of measurements belong to one of multiple groups of examples on which the machine was previously trained. Prior to real-world deployment, all implementations need to be carefully evaluated with hold-out validation, where the algorithm is tested on different samples than it was provided for training, in order to ensure the generalizability and reliability of AI models. However, established methods for performing hold-out validation do not assess the consistency of the mistakes that the AI model makes during hold-out validation. Here, we show that in addition to standard methods, an enhanced technique for performing hold-out validation—that also assesses the consistency of the sample-wise mistakes made by the learning algorithm—can assist in the evaluation and design of reliable and predictable AI models. The technique can be applied to the validation of any supervised learning classification application, and we demonstrate the use of the technique on a variety of example biomedical diagnostic applications, which help illustrate the importance of producing reliable AI models. The validation software created is made publicly available, assisting anyone developing AI models for any supervised classification application in the creation of more reliable and predictable technologies.
更多查看译文
关键词
classification,error consistency,supervised machine learning,validation
AI 理解论文
溯源树
样例
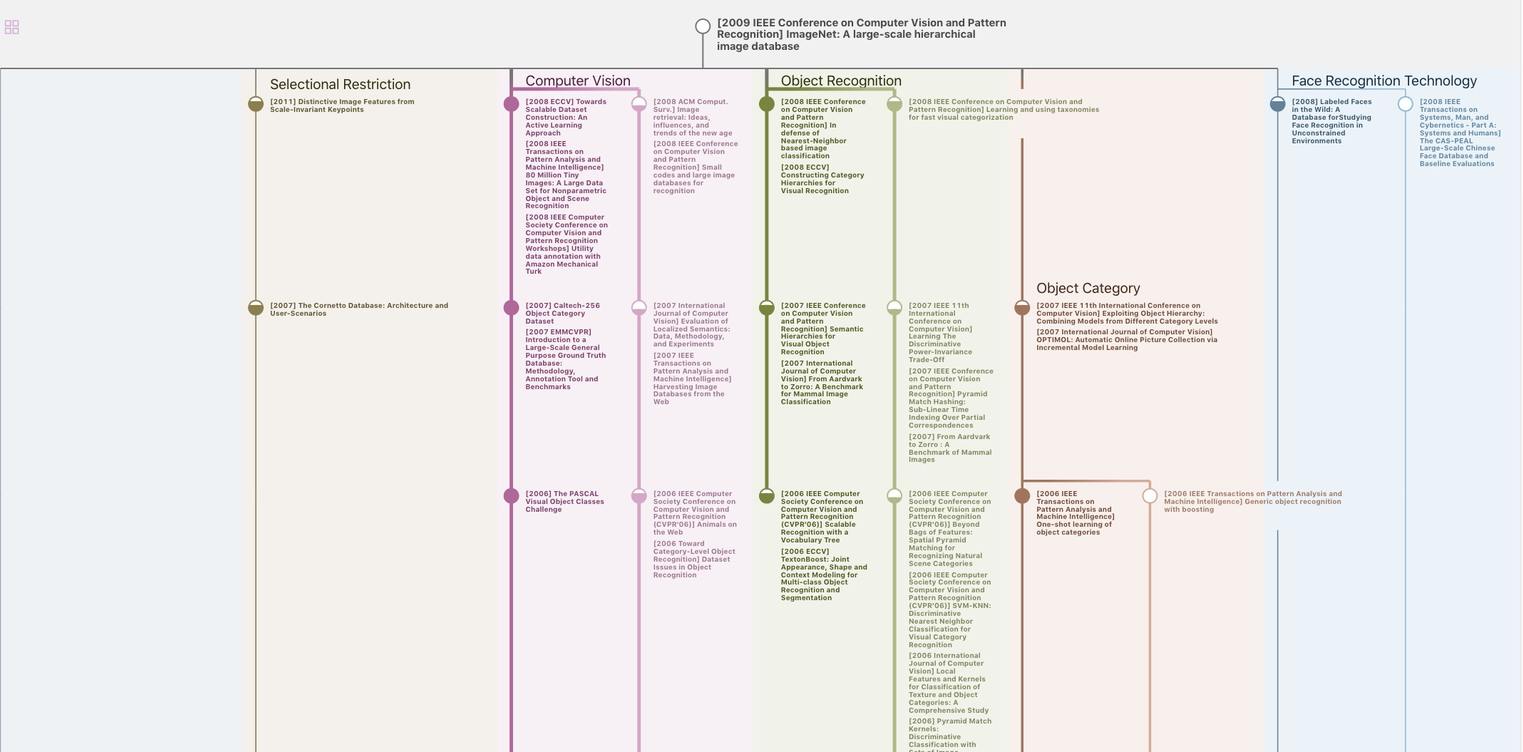
生成溯源树,研究论文发展脉络
Chat Paper
正在生成论文摘要