Machine Learning Based Representative Spatio-Temporal Event Documents Classification
Applied Sciences(2023)
摘要
As the scale of online news and social media expands, attempts to analyze the latest social issues and consumer trends are increasing. Research on detecting spatio-temporal event sentences in text data is being actively conducted. However, a document contains important spatio-temporal events necessary for event analysis, as well as non-critical events for event analysis. It is important to increase the accuracy of event analysis by extracting only the key events necessary for event analysis from among a large number of events. In this study, we define important 'representative spatio-temporal event documents' for the core subject of documents and propose a BiLSTM-based document classification model to classify representative spatio-temporal event documents. We build 10,000 gold-standard training datasets to train the proposed BiLSTM model. The experimental results show that our BiLSTM model improves the F1 score by 2.6% and the accuracy by 4.5% compared to the baseline CNN model.
更多查看译文
关键词
BiLSTM,representative spatio-temporal event,document classification
AI 理解论文
溯源树
样例
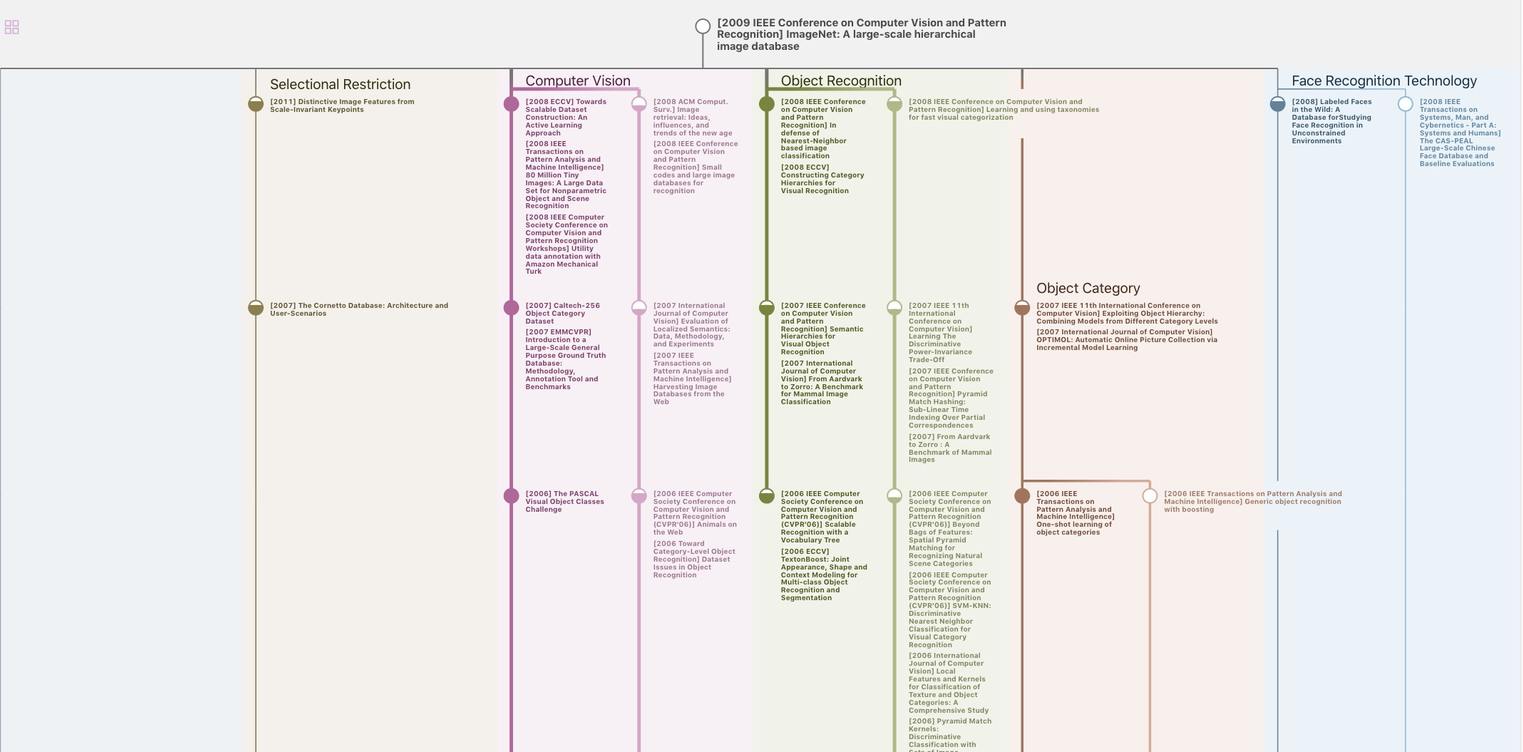
生成溯源树,研究论文发展脉络
Chat Paper
正在生成论文摘要