An Integrated Deep-Learning-Based Approach for Energy Consumption Prediction of Machining Systems
Sustainability(2023)
摘要
Large and extensive manufacturing systems consume a large proportion of manufacturing energy. A key component of energy efficiency management is the accurate prediction of energy efficiency. However, the nonlinear and vibration characteristics of machining systems’ energy consumption (EC) pose a challenge to the accurate prediction of system EC. To address this challenge, an energy consumption prediction method for machining systems is presented, which is based on an improved particle swarm optimization (IPSO) algorithm to optimize long short-term memory (LSTM) neural networks. The proposed method optimizes the LSTM hyperparameters by improving the particle swarm algorithm with dynamic inertia weights (DIWPSO-LSTM), which enhances the prediction accuracy and efficiency of the model. In the experimental results, we compared several improved optimization algorithms, and the proposed method has a performance improvement of more than 30% in mean absolute error (MAE)and mean error(ME).
更多查看译文
关键词
energy consumption prediction,particle swarm optimization algorithm of dynamic inertia weights (DIWPSO),long short-term memory network (LSTM),machining systems,deep learning
AI 理解论文
溯源树
样例
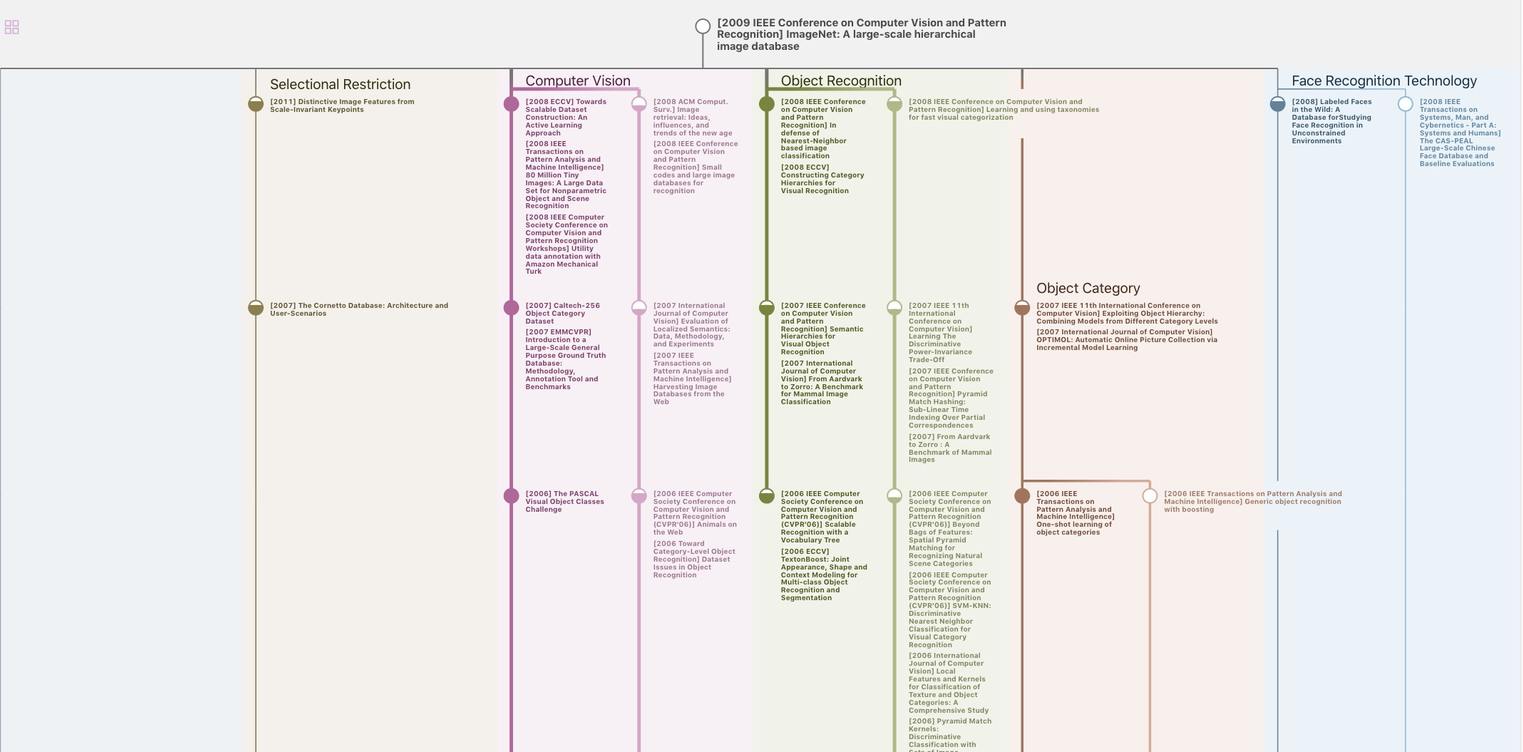
生成溯源树,研究论文发展脉络
Chat Paper
正在生成论文摘要