Masked Graph Convolutional Network for Small Sample Classification of Hyperspectral Images
Remote Sensing(2023)
摘要
The deep learning method has achieved great success in hyperspectral image classification, but the lack of labeled training samples still restricts the development and application of deep learning methods. In order to deal with the problem of small samples in hyperspectral image classification, a novel small sample classification method based on rotation-invariant uniform local binary pattern (RULBP) features and a graph-based masked autoencoder is proposed in this paper. Firstly, the RULBP features of hyperspectral images are extracted, and then the k-nearest neighbor method is utilized to construct the graph. Furthermore, self-supervised learning is conducted on the constructed graph so that the model can learn to extract features more suitable for small sample classification. Since the self-supervised training mainly adopts the masked autoencoder method, only unlabeled samples are needed to complete the training. After training, only a small number of samples are used to fine-tune the graph convolutional network, so as to complete the classification of all nodes in the graph. A large number of classification experiments on three commonly used hyperspectral image datasets show that the proposed method could achieve higher classification accuracy with fewer labeled samples.
更多查看译文
关键词
hyperspectral image classification,graph convolutional network,masked autoencoder,rotation-invariant uniform local binary pattern
AI 理解论文
溯源树
样例
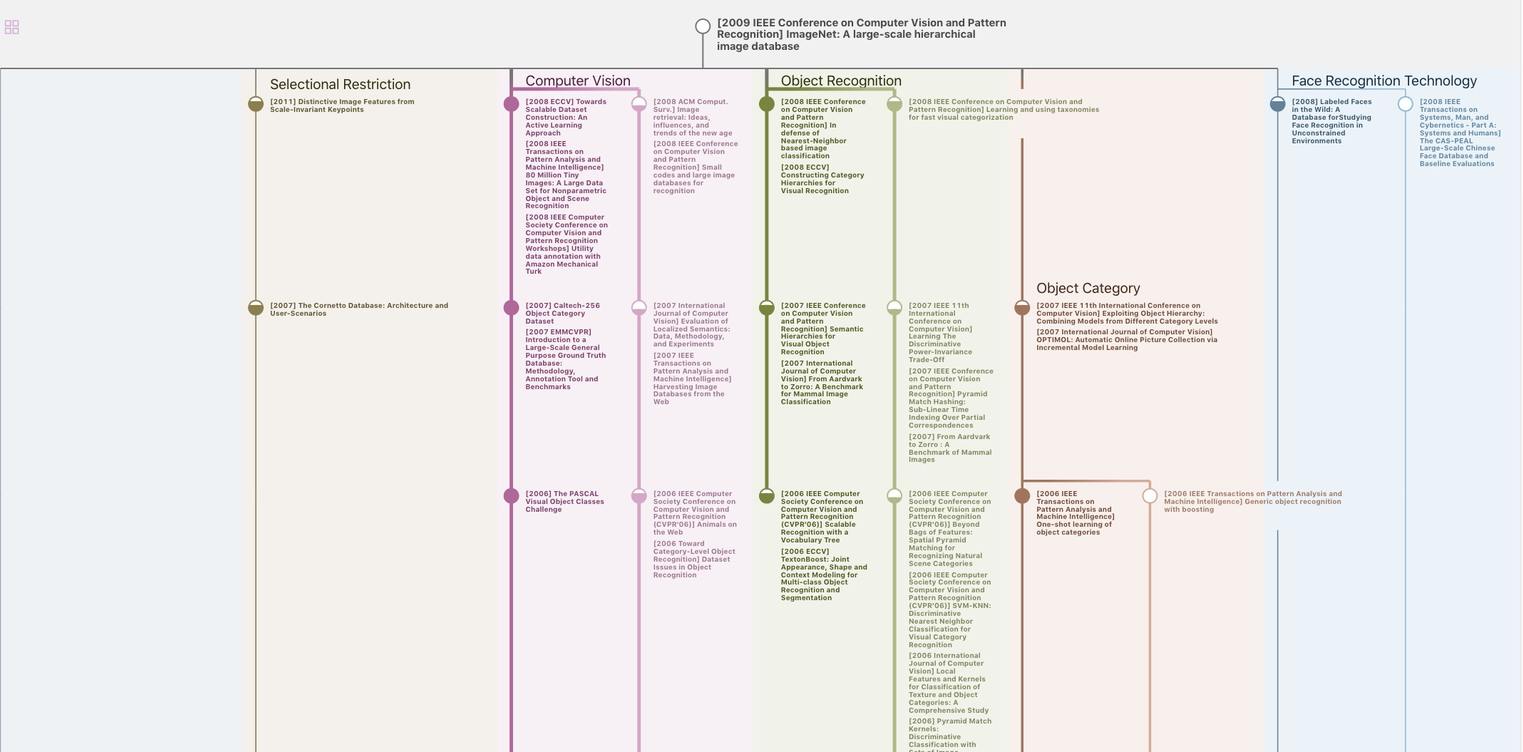
生成溯源树,研究论文发展脉络
Chat Paper
正在生成论文摘要