HY1C/D-CZI Noctiluca scintillans Bloom Recognition Network Based on Hybrid Convolution and Self-Attention
Remote Sensing(2023)
摘要
Accurate Noctiluca scintillans bloom (NSB) recognition from space is of great significance for marine ecological monitoring and underwater target detection. However, most existing NSB recognition models require expert visual interpretation or manual adjustment of model thresholds, which limits model application in operational NSB monitoring. To address these problems, we developed a Noctiluca scintillans Bloom Recognition Network (NSBRNet) incorporating an Inception Conv Block (ICB) and a Swin Attention Block (SAB) based on the latest deep learning technology, where ICB uses convolution to extract channel and local detail features, and SAB uses self-attention to extract global spatial features. The model was applied to Coastal Zone Imager (CZI) data onboard Chinese ocean color satellites (HY1C/D). The results show that NSBRNet can automatically identify NSB using CZI data. Compared with other common semantic segmentation models, NSBRNet showed better performance with a precision of 92.22%, recall of 88.20%, F1-score of 90.10%, and IOU of 82.18%.
更多查看译文
关键词
<i>Noctiluca scintillans</i> blooms,remote sensing,deep learning,HY-1C/D,CZI
AI 理解论文
溯源树
样例
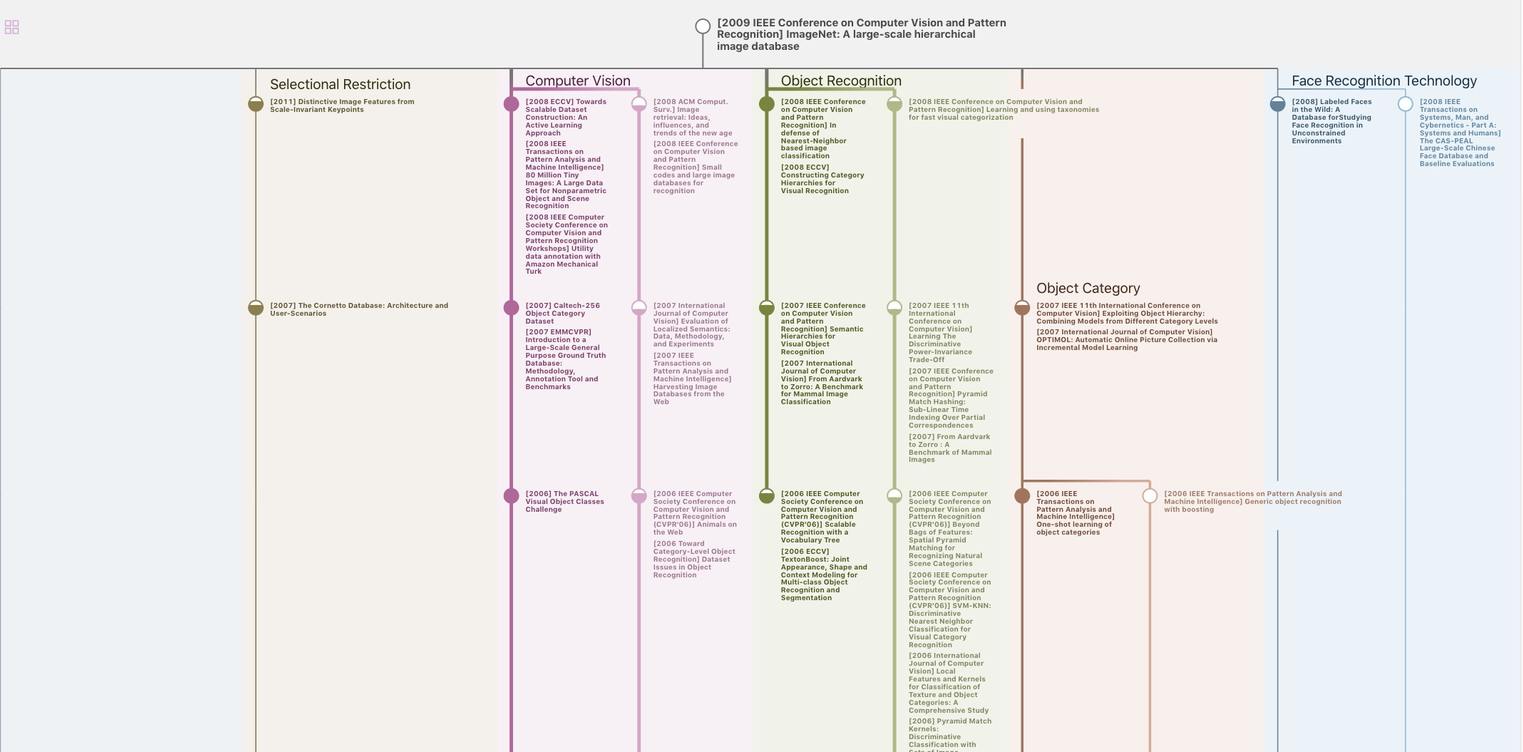
生成溯源树,研究论文发展脉络
Chat Paper
正在生成论文摘要