Algorithmic Graph Theory, Reinforcement Learning and Game Theory in MD Simulations: From 3D Structures to Topological 2D-Molecular Graphs (2D-MolGraphs) and Vice Versa
Molecules(2023)
摘要
This paper reviews graph-theory-based methods that were recently developed in our group for post-processing molecular dynamics trajectories. We show that the use of algorithmic graph theory not only provides a direct and fast methodology to identify conformers sampled over time but also allows to follow the interconversions between the conformers through graphs of transitions in time. Examples of gas phase molecules and inhomogeneous aqueous solid interfaces are presented to demonstrate the power of topological 2D graphs and their versatility for post-processing molecular dynamics trajectories. An even more complex challenge is to predict 3D structures from topological 2D graphs. Our first attempts to tackle such a challenge are presented with the development of game theory and reinforcement learning methods for predicting the 3D structure of a gas-phase peptide.
更多查看译文
关键词
algorithmic graph theory,molecular dynamics,identification of conformers,machine learning,game theory,pathways,conformational conversion,prediction of 3D structures
AI 理解论文
溯源树
样例
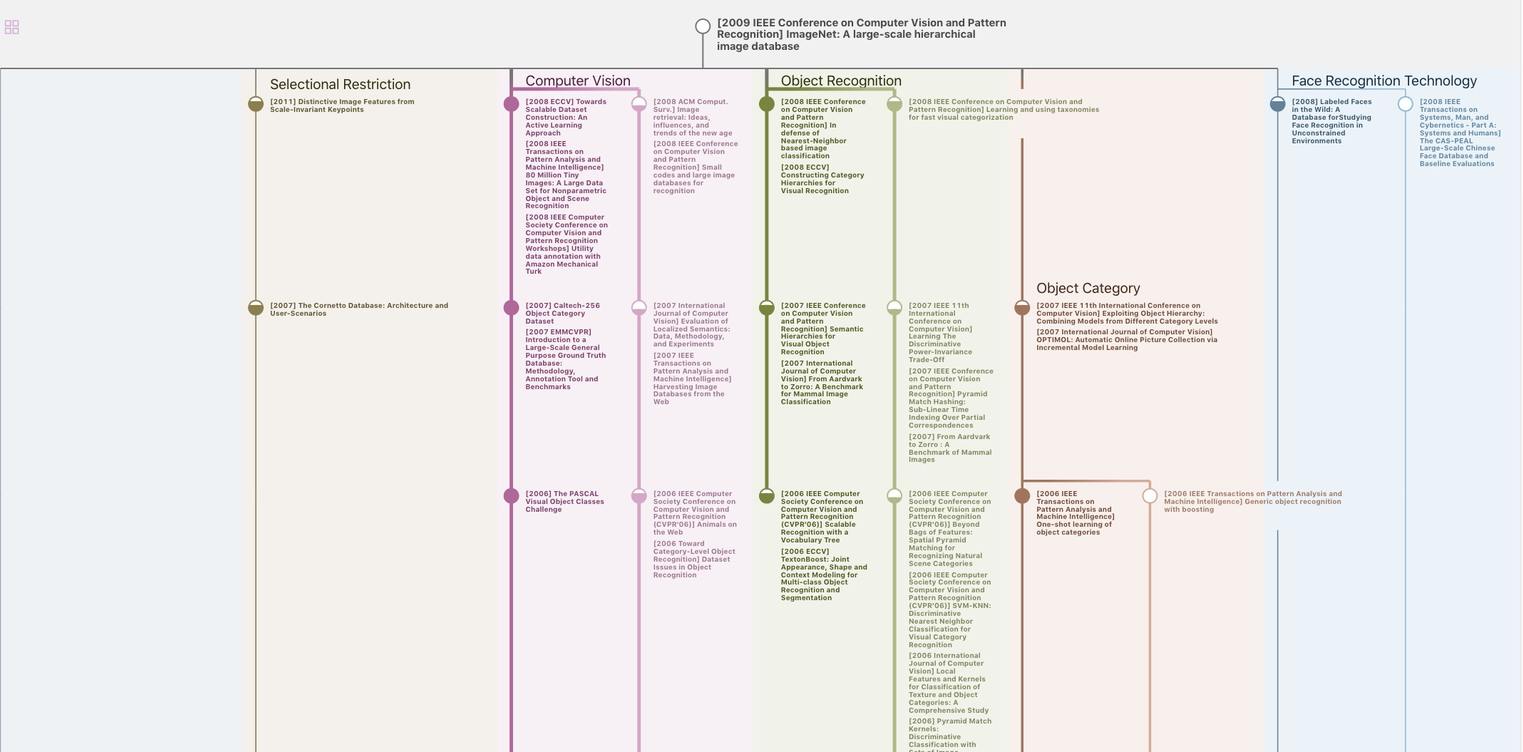
生成溯源树,研究论文发展脉络
Chat Paper
正在生成论文摘要