BTDM: A Bi-Directional Translating Decoding Model-Based Relational Triple Extraction
Applied Sciences(2023)
摘要
The goal of relational triple extraction is to extract knowledge-rich relational triples from unstructured text. Although the previous methods obtain considerable performance, there are still some problems, such as error propagation, the overlapping triple problem, and suboptimal subject–object alignment. To address the shortcomings above, in this paper, we decompose this task into three subtasks from a fresh perspective: entity extraction, subject–object alignment and relation judgement, as well as propose a novel bi-directional translating decoding model (BTDM). Specifically, a bidirectional translating decoding structure is designed to perform entity extraction and subject–object alignment, which decodes entity pairs from both forward and backward extraction. The bidirectional structure effectively mitigates the error propagation problem and aligns the subject–object pairs. The translating decoding approach handles the overlapping triple problem. Finally, a (entity pair, relation) bipartite graph is designed to achieve practical relationship judgement. Experiments show that our model outperforms previous methods and achieves state-of-the-art performance on NYT and WebNLG. We achieved F1-scores of 92.7% and 93.8% on the two datasets. Meanwhile, in various complementary experiments on complex scenarios, our model demonstrates consistent performance gain in various complex scenarios.
更多查看译文
关键词
entity and relation extraction,information extraction,nature language processing,error propagation,overlapping triples
AI 理解论文
溯源树
样例
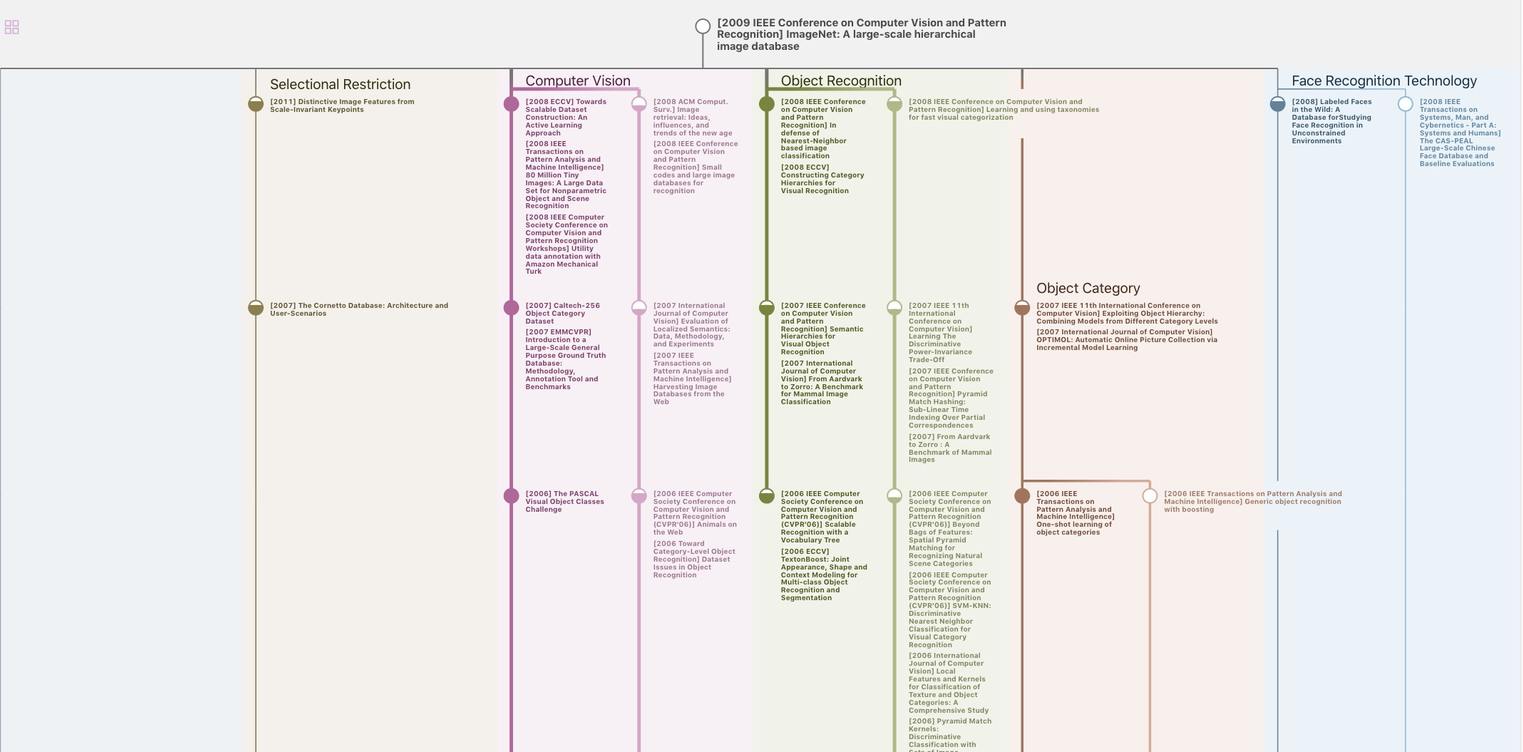
生成溯源树,研究论文发展脉络
Chat Paper
正在生成论文摘要