Multi-scale Geometry-aware Transformer for 3D Point Cloud Classification
CoRR(2023)
摘要
Self-attention modules have demonstrated remarkable capabilities in capturing long-range relationships and improving the performance of point cloud tasks. However, point cloud objects are typically characterized by complex, disordered, and non-Euclidean spatial structures with multiple scales, and their behavior is often dynamic and unpredictable. The current self-attention modules mostly rely on dot product multiplication and dimension alignment among query-key-value features, which cannot adequately capture the multi-scale non-Euclidean structures of point cloud objects. To address these problems, this paper proposes a self-attention plug-in module with its variants, Multi-scale Geometry-aware Transformer (MGT). MGT processes point cloud data with multi-scale local and global geometric information in the following three aspects. At first, the MGT divides point cloud data into patches with multiple scales. Secondly, a local feature extractor based on sphere mapping is proposed to explore the geometry inner each patch and generate a fixed-length representation for each patch. Thirdly, the fixed-length representations are fed into a novel geodesic-based self-attention to capture the global non-Euclidean geometry between patches. Finally, all the modules are integrated into the framework of MGT with an end-to-end training scheme. Experimental results demonstrate that the MGT vastly increases the capability of capturing multi-scale geometry using the self-attention mechanism and achieves strong competitive performance on mainstream point cloud benchmarks.
更多查看译文
关键词
3d,multi-scale,geometry-aware
AI 理解论文
溯源树
样例
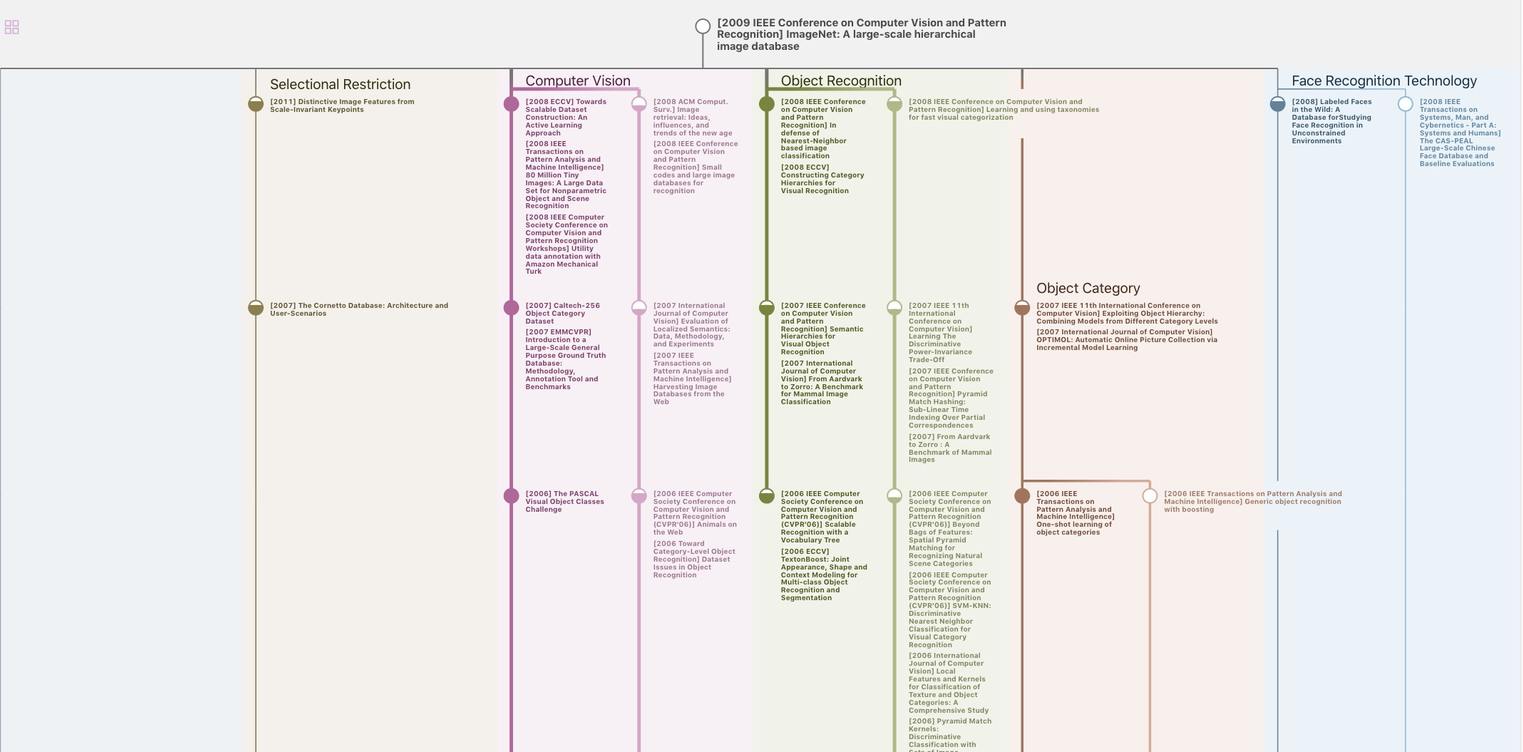
生成溯源树,研究论文发展脉络
Chat Paper
正在生成论文摘要