Communicating Uncertainty in Machine Learning Explanations: A Visualization Analytics Approach for Predictive Process Monitoring
CoRR(2023)
摘要
As data-driven intelligent systems advance, the need for reliable and transparent decision-making mechanisms has become increasingly important. Therefore, it is essential to integrate uncertainty quantification and model explainability approaches to foster trustworthy business and operational process analytics. This study explores how model uncertainty can be effectively communicated in global and local post-hoc explanation approaches, such as Partial Dependence Plots (PDP) and Individual Conditional Expectation (ICE) plots. In addition, this study examines appropriate visualization analytics approaches to facilitate such methodological integration. By combining these two research directions, decision-makers can not only justify the plausibility of explanation-driven actionable insights but also validate their reliability. Finally, the study includes expert interviews to assess the suitability of the proposed approach and designed interface for a real-world predictive process monitoring problem in the manufacturing domain.
更多查看译文
关键词
machine learning explanations,visualization analytics approach,monitoring,machine learning
AI 理解论文
溯源树
样例
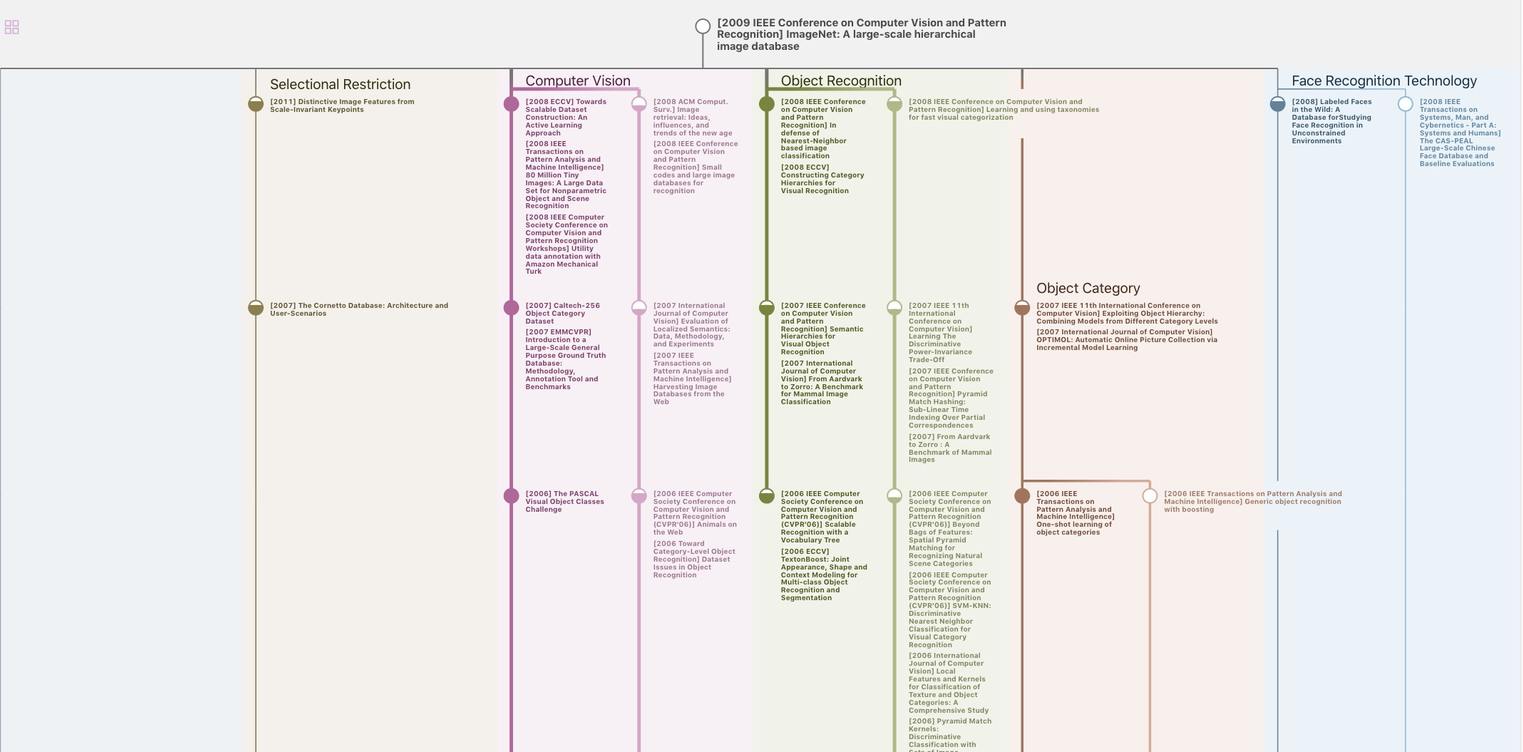
生成溯源树,研究论文发展脉络
Chat Paper
正在生成论文摘要