RESET: Revisiting Trajectory Sets for Conditional Behavior Prediction
CoRR(2023)
摘要
It is desirable to predict the behavior of traffic participants conditioned on different planned trajectories of the autonomous vehicle. This allows the downstream planner to estimate the impact of its decisions. Recent approaches for conditional behavior prediction rely on a regression decoder, meaning that coordinates or polynomial coefficients are regressed. In this work we revisit set-based trajectory prediction, where the probability of each trajectory in a predefined trajectory set is determined by a classification model, and first-time employ it to the task of conditional behavior prediction. We propose RESET, which combines a new metric-driven algorithm for trajectory set generation with a graph-based encoder. For unconditional prediction, RESET achieves comparable performance to a regression-based approach. Due to the nature of set-based approaches, it has the advantageous property of being able to predict a flexible number of trajectories without influencing runtime or complexity. For conditional prediction, RESET achieves reasonable results with late fusion of the planned trajectory, which was not observed for regression-based approaches before. This means that RESET is computationally lightweight to combine with a planner that proposes multiple future plans of the autonomous vehicle, as large parts of the forward pass can be reused.
更多查看译文
关键词
Behavior-Based Systems,Motion and Path Planning,AI-Based Methods
AI 理解论文
溯源树
样例
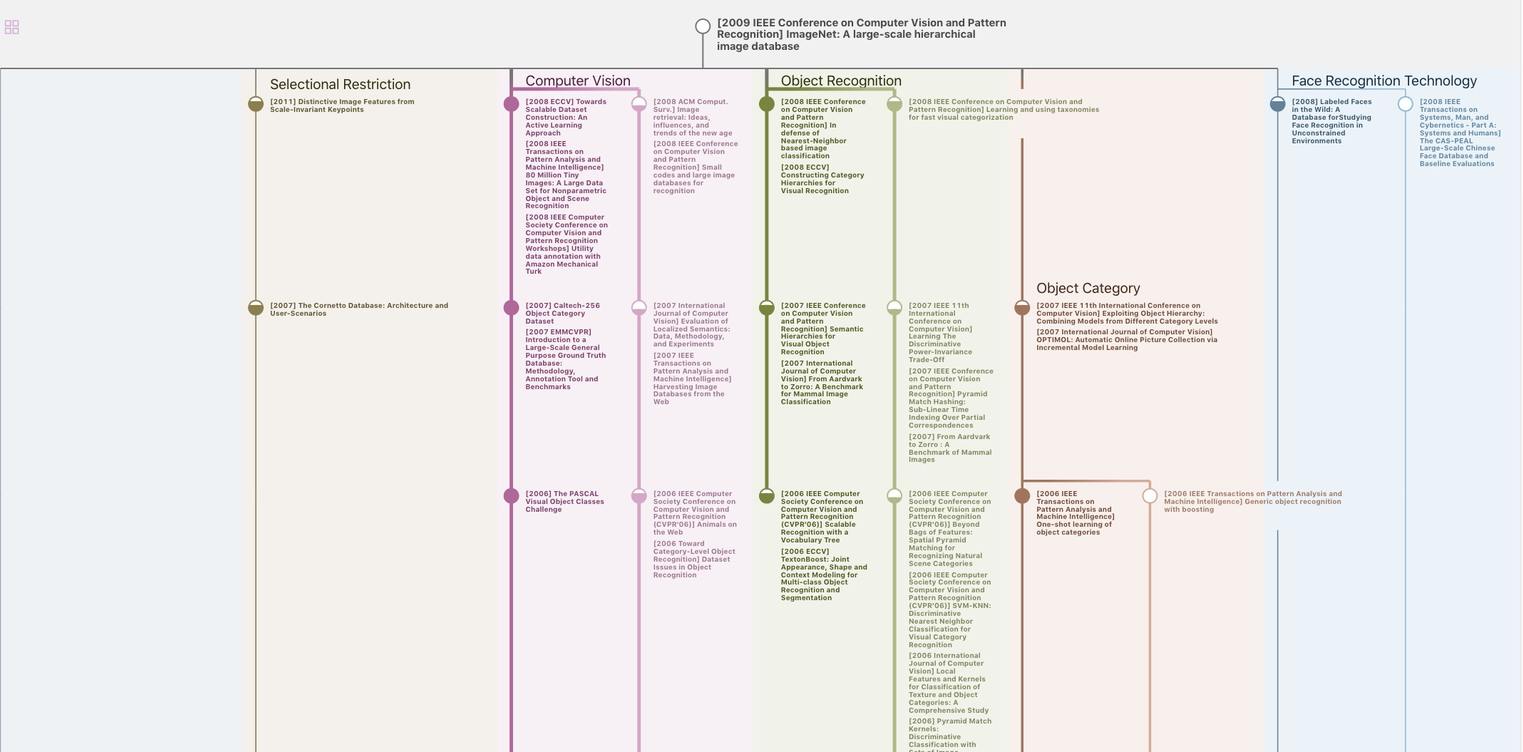
生成溯源树,研究论文发展脉络
Chat Paper
正在生成论文摘要