Auditing ICU Readmission Rates in an Clinical Database: An Analysis of Risk Factors and Clinical Outcomes
CoRR(2023)
摘要
This study presents a machine learning (ML) pipeline for clinical data classification in the context of a 30-day readmission problem, along with a fairness audit on subgroups based on sensitive attributes. A range of ML models are used for classification and the fairness audit is conducted on the model predictions. The fairness audit uncovers disparities in equal opportunity, predictive parity, false positive rate parity, and false negative rate parity criteria on the MIMIC III dataset based on attributes such as gender, ethnicity, language, and insurance group. The results identify disparities in the model's performance across different groups and highlight the need for better fairness and bias mitigation strategies. The study suggests the need for collaborative efforts among researchers, policymakers, and practitioners to address bias and fairness in artificial intelligence (AI) systems.
更多查看译文
关键词
icu readmission rates,clinical outcomes,clinical database
AI 理解论文
溯源树
样例
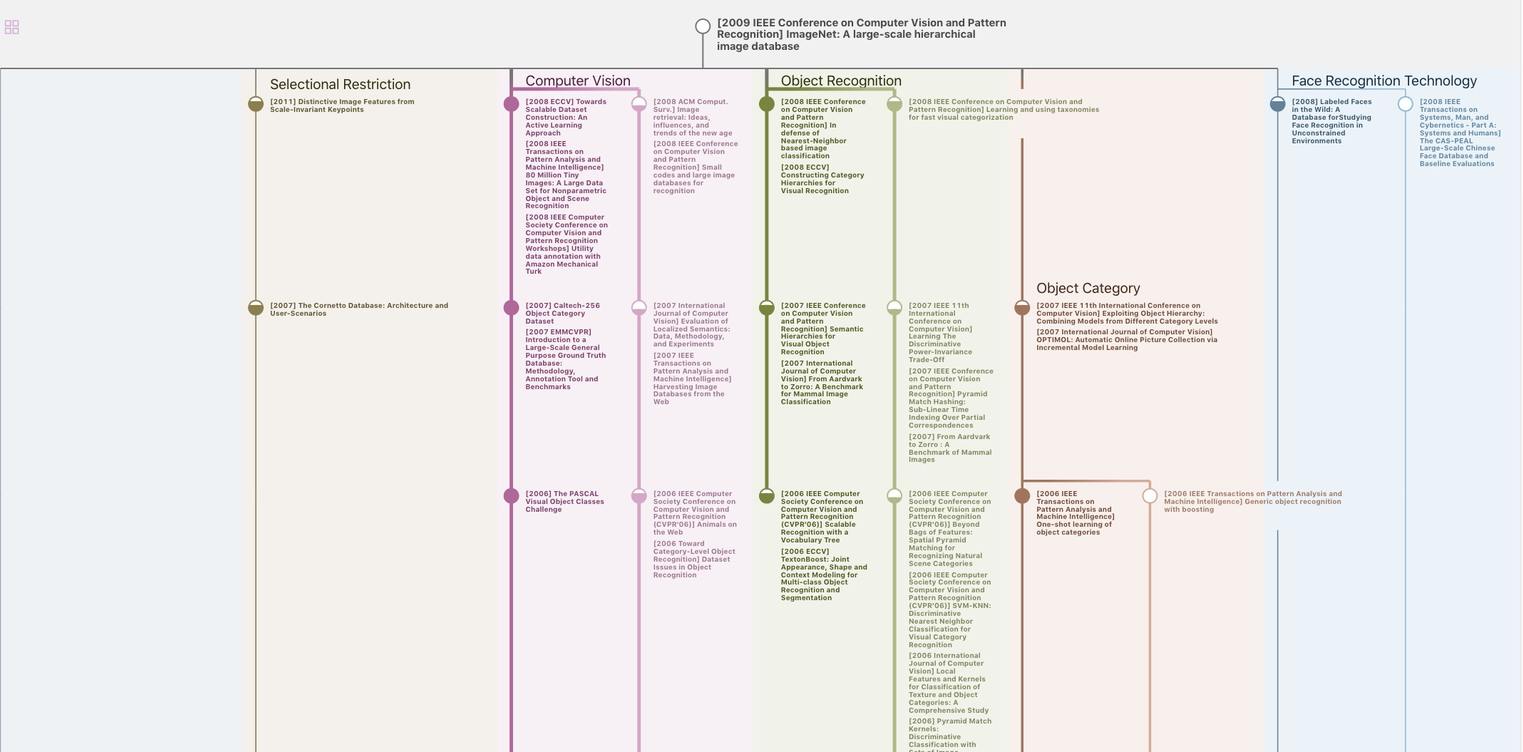
生成溯源树,研究论文发展脉络
Chat Paper
正在生成论文摘要