Early Detection of Crop Types by Integrating Sentinel-1A and Sentinel-2 Imagery based on the CNN Model
crossref(2023)
摘要
Early-season crop-type data are required for a variety of agricultural monitoring and decision-making applications. The early season in this study referred to the prophase and middle of a growth season. Early-season detection of crop types remains challenging because of limited discriminative features, especially for landscapes that are characterized by complex cropping patterns. In fact, different remote-sensing satellites can increase the frequency of data acquisition, which can provide more information in the early season. Moreover, optical and radar sensors have different degrees of sensitivity to crop parameters. Therefore, the integration and application of two types of multitemporal data are of great significance to improve the accuracy and timeliness of crop type detection.In deep learning (DL), convolutional neural network (CNN) and recurrent neural network (RNN) models have great potential for temporal feature extraction. Compared with RNNs, CNNs usually have fewer parameters and are more conducive to determining early-season detection dates of different crop types, which requires a lot of training because of the need to model on different dates. Nevertheless, revisit dates and temporal intervals of different satellites are usually different, resulting in different data acquisition time series; these data cannot be directly used as input for the same convolutional layer. To address this challenge, a Dual-1DCNN was bulit based on the CNN model in this study. Moreover, an incremental training method was used to attain the network on each data acquisition date and obtained the best detection date for each crop type in the early season. A case study for Hengshui City in China was conducted using time series of Sentinel-1A (S1A) and Sentinel-2 (S2). To verify this method, classical methods support vector machine (SVM) and random forest (RF) were implemented. The results demonstrated the following: (1) the Dual-1DCNN extracted discriminative features from S1A and S2 time series at the early season by producing the highest overall accuracy (OA: 87.23%); (2) for summer maize, cotton, and common yam rhizome, the Dual-1DCNN achieved F1 values of 92.39%, 87.71%, and 84.38%, respectively, at the early season (moreover, the early seasons were almost 40, 70, and 80 days before the end of the growth seasons, respectively). These findings suggested that the Dual-1DCNN is promising for the accurate and timely detection of crop types.
更多查看译文
AI 理解论文
溯源树
样例
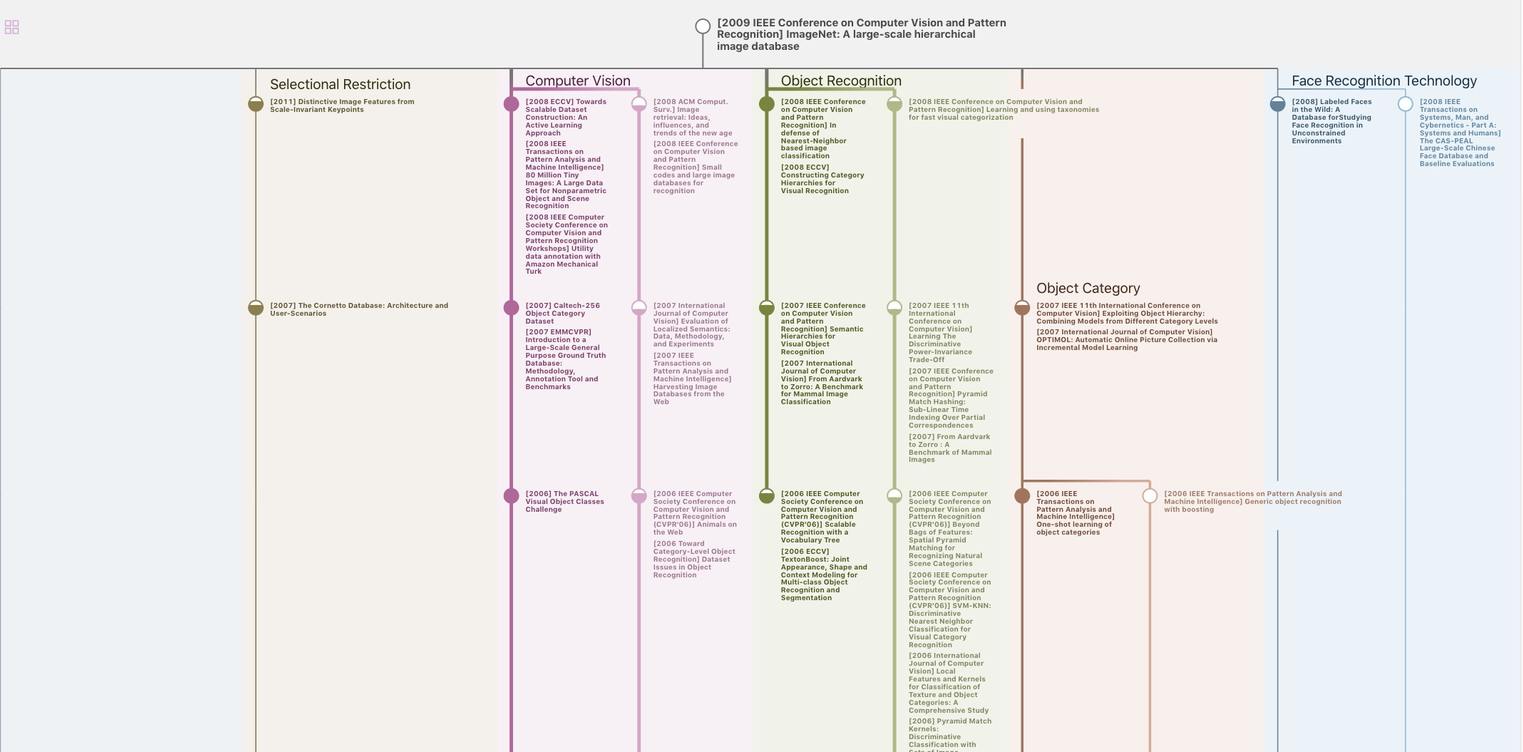
生成溯源树,研究论文发展脉络
Chat Paper
正在生成论文摘要