Does Artificial Intelligence Dream of Elevated Natriuretic Peptide in Chest Radiography?
CIRCULATION(2023)
摘要
BACKGROUND Heart failure pandemic is a medical issue, and its diagnosis can be challenging.
OBJECTIVES To assess the hypothesis that the artificial intelligence (AI) model for predicting elevated brain natriuretic peptide (BNP) levels from chest X-ray images has sufficient performance and could improve the diagnostic performance of human.
METHODS Patients who underwent chest radiography and BNP testing on the same day were enrolled in this study. The chest X-ray images were labeled based on plasma BNP levels >= 200 pg/mL. We trained models that predicted the label, and the final ensemble model was constructed. Humans were trained and tested to predict labels, followed by the same test, referring to the prediction of the AI model. Diagnostic performance was assessed.
RESULTS Among the 1607 patients, 10103 chest X-ray images and BNP values were collected. We assembled 31 models as the final model with an accuracy of 0.855, precision of 0.873, recall of 0.827, receiver-operating-characteristics area-under-curve (AUC) of 0.929, and precision-recall AUC of 0.934. The accuracy of the testing by 35 individuals significantly improved from 0.708±0.049 to 0.829±0.069 (P < 0.001) with AI assistance. The accuracy of the experts was higher than that of non-experts (0.692±0.042 vs. 0.728±0.051, P = 0.030); however, with the AI assistance, the accuracy of the non-experts was rather higher than that of the experts (0.851±0.074 vs. 0.803±0.054, P = 0.033).
CONCLUSIONS The AI model can predict elevated BNP levels from chest X-ray images and could improve the diagnostic performance of humans.
Condensed Abstract We developed the AI model to predict elevated natriuretic peptide levels from chest radiography and evaluated its performance. We tested the performance of humans and examined whether their performance could be improved by referring the AI predictions. The AI model achieved an accuracy of 0.855 and a receiver-operating-characteristics area-under-curve of 0.929. The accuracy of human testing was significantly improved from 0.708±0.049 to 0.829±0.069 (P < 0.001) with AI assistance. The accuracy of the experts was higher than that of non-experts; however, with the AI assistance, the accuracy of the non-experts was higher than that of the experts.
### Competing Interest Statement
The authors have declared no competing interest.
### Funding Statement
There is no financial support for this study
### Author Declarations
I confirm all relevant ethical guidelines have been followed, and any necessary IRB and/or ethics committee approvals have been obtained.
Yes
The details of the IRB/oversight body that provided approval or exemption for the research described are given below:
IRB of Hiroshima City Asa Hospital gave ethical approval for this work.
I confirm that all necessary patient/participant consent has been obtained and the appropriate institutional forms have been archived, and that any patient/participant/sample identifiers included were not known to anyone (e.g., hospital staff, patients or participants themselves) outside the research group so cannot be used to identify individuals.
Yes
I understand that all clinical trials and any other prospective interventional studies must be registered with an ICMJE-approved registry, such as ClinicalTrials.gov. I confirm that any such study reported in the manuscript has been registered and the trial registration ID is provided (note: if posting a prospective study registered retrospectively, please provide a statement in the trial ID field explaining why the study was not registered in advance).
Yes
I have followed all appropriate research reporting guidelines, such as any relevant EQUATOR Network research reporting checklist(s) and other pertinent material, if applicable.
Yes
The source codes and weights of the models used in this study will be available on GitHub.
[https://github.com/ekagawa007/kagawa\_for\_review][1]
* AI
: Artificial intelligence
AUC
: Area-under curve
BNP
: Brain natriuretic peptide
GRAD-CAM
: Gradient-class activation maps
MAE
: Mean absolute errors
PR
: Precision-recall
ROC
: Receiver-operating-characteristics
SD
: Standard deviation
[1]: https://github.com/ekagawa007/kagawa_for_review
更多查看译文
关键词
Artificial Intelligence,Heart Failure,X-ray,Natriuretic peptide,Deep learning
AI 理解论文
溯源树
样例
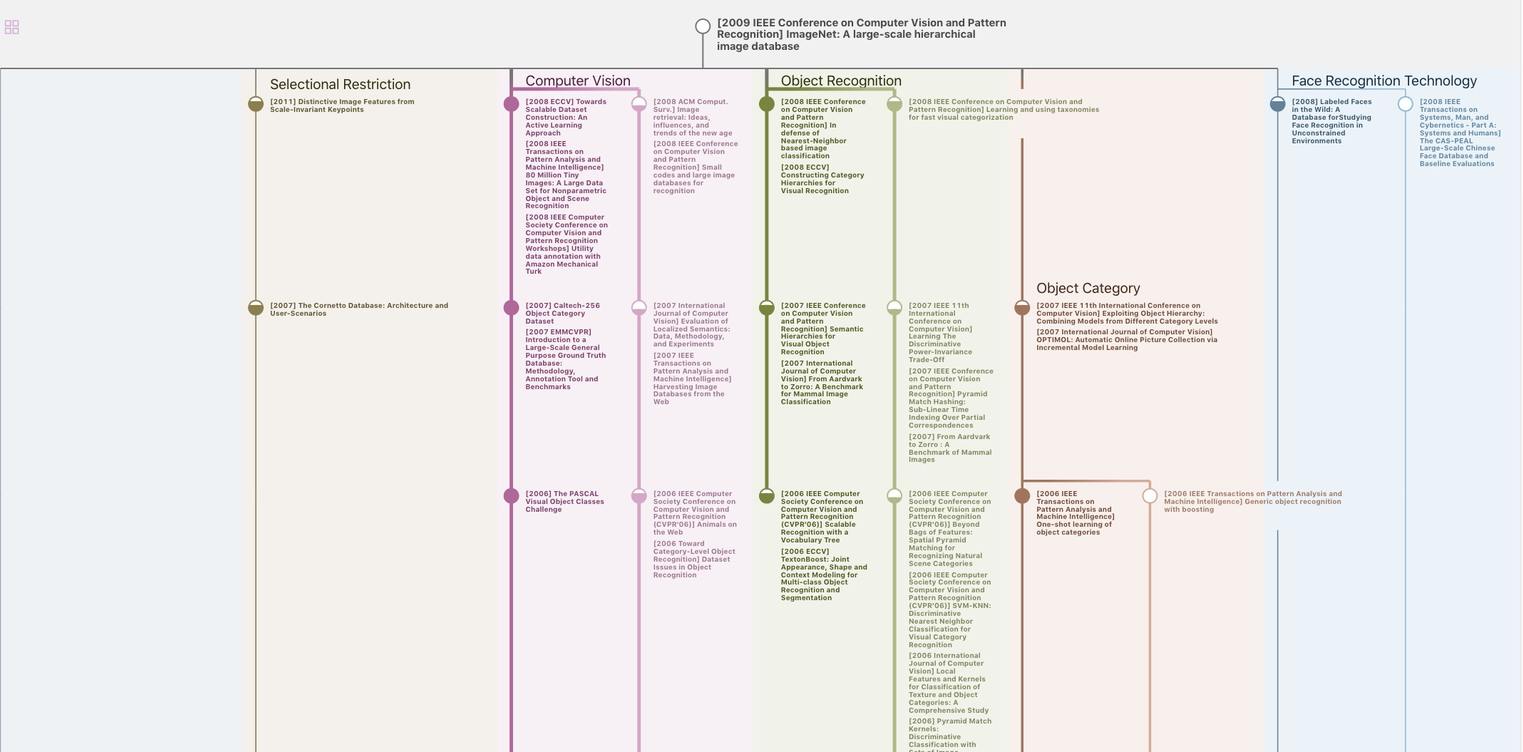
生成溯源树,研究论文发展脉络
Chat Paper
正在生成论文摘要