Using natural language processing to study homelessness longitudinally with electronic health record data subject to irregular observations.
medRxiv (Cold Spring Harbor Laboratory)(2024)
摘要
The Electronic Health Record (EHR) contains information about social determinants of health (SDoH) such as homelessness. Much of this information is contained in clinical notes and can be extracted using natural language processing (NLP). This data can provide valuable information for researchers and policymakers studying long-term housing outcomes for individuals with a history of homelessness. However, studying homelessness longitudinally in the EHR is challenging due to irregular observation times. In this work, we applied an NLP system to extract housing status for a cohort of patients in the US Department of Veterans Affairs (VA) over a three-year period. We then applied inverse intensity weighting to adjust for the irregularity of observations, which was used generalized estimating equations to estimate the probability of unstable housing each day after entering a VA housing assistance program. Our methods generate unique insights into the long-term outcomes of individuals with a history of homelessness and demonstrate the potential for using EHR data for research and policymaking.
更多查看译文
关键词
homelessness,natural language processing,electronic health record data
AI 理解论文
溯源树
样例
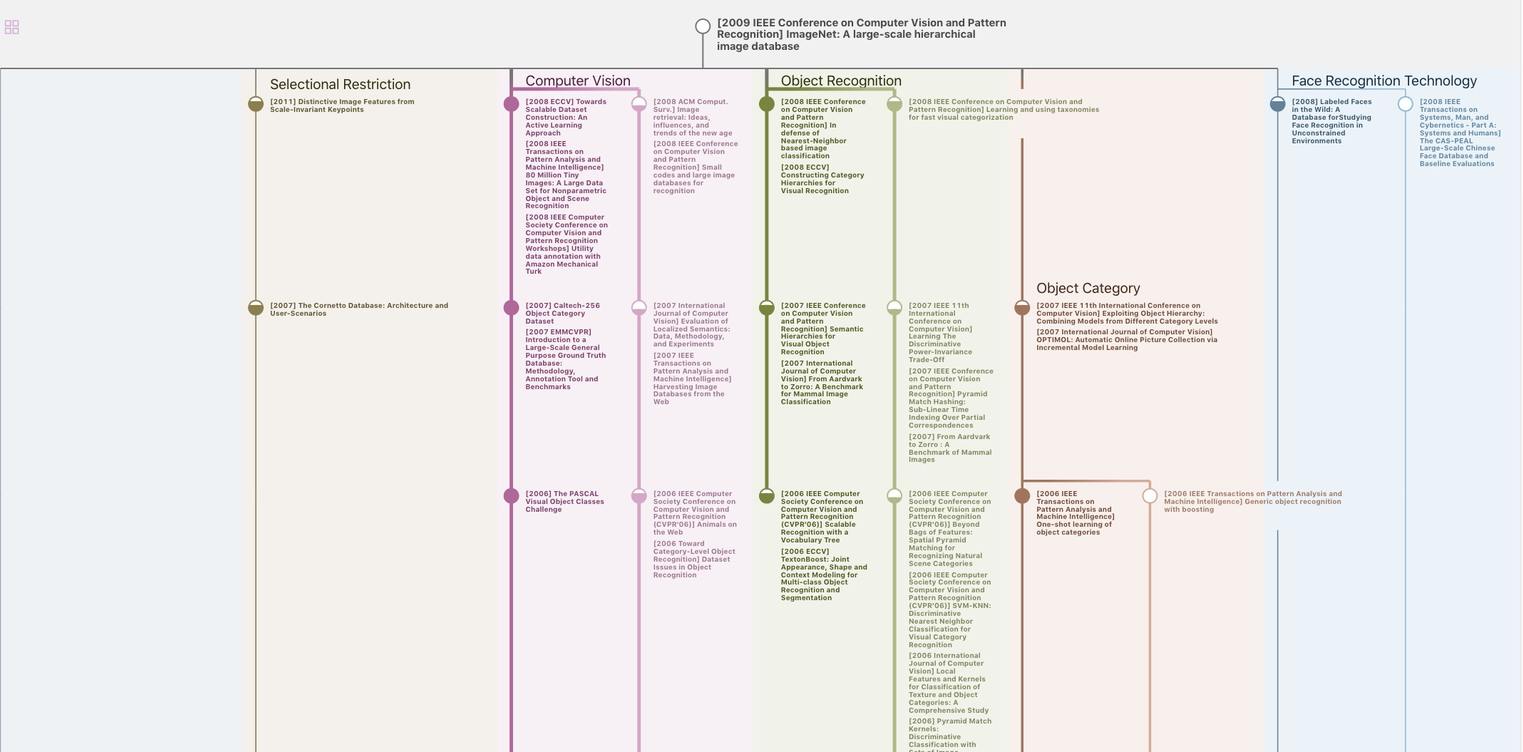
生成溯源树,研究论文发展脉络
Chat Paper
正在生成论文摘要