Real-time forecasting of COVID-19-related hospital strain in France using a non-Markovian mechanistic model
medrxiv(2023)
摘要
Background The COVID-19 pandemic emphasised the importance of access to reliable real-time forecasts for key epidemiological indicators. Given the strong heterogeneity between regions, providing forecasts at the local level is essential for health professionals.
Methods We developed a SARS-CoV-2 transmission model in France, COVIDici, that performs parameter estimation using up-to-date vaccination coverage and hospital data to provide forecasts up to a four-week horizon based on the current epidemic trend. We present the model, its associated online tool and perform a retrospective evaluation of the forecasts provided from January to December 2021 by comparing to three standard statistical forecasting methods (auto-regression, exponential smoothing, and ARIMA) at the national and regional levels.
Results COVIDici allowed simultaneous real-time visualisation of several indicators of the COVID-19 epidemic at the sub-national level. For anticipating risk of critical care unit overload, it performed worse compared to the baseline methods for forecasts under the three-week horizon, but had better point forecasts at the longest horizons (e.g. four weeks) for 8 of the 13 regions considered depending on the metric.
Conclusions Effective communication between modelers and clinicians is essential for utilising forecasts for health care planning. Online visualisation tools and consideration of how metrics can be affected by distortion from non-pharmaceutical government interventions facilitate this dialogue.
What is already known on this topic
What this study adds
How this study might affect research, practice or policy
### Competing Interest Statement
The authors have declared no competing interest.
### Funding Statement
This study was funded by the PaSSES grant under the MODCOV19 platform (INSMI and CRNS).
### Author Declarations
I confirm all relevant ethical guidelines have been followed, and any necessary IRB and/or ethics committee approvals have been obtained.
Yes
The details of the IRB/oversight body that provided approval or exemption for the research described are given below:
The study used ONLY openly available human data that were originally located at Santé Publique France.
I confirm that all necessary patient/participant consent has been obtained and the appropriate institutional forms have been archived, and that any patient/participant/sample identifiers included were not known to anyone (e.g., hospital staff, patients or participants themselves) outside the research group so cannot be used to identify individuals.
Yes
I understand that all clinical trials and any other prospective interventional studies must be registered with an ICMJE-approved registry, such as ClinicalTrials.gov. I confirm that any such study reported in the manuscript has been registered and the trial registration ID is provided (note: if posting a prospective study registered retrospectively, please provide a statement in the trial ID field explaining why the study was not registered in advance).
Yes
I have followed all appropriate research reporting guidelines and uploaded the relevant EQUATOR Network research reporting checklist(s) and other pertinent material as supplementary files, if applicable.
Yes
The model in this manuscript is based on publicly available data provided by Santé Publique France. The scripts and data used to perform the analysis and generate this manuscript are available on GitLab () and archived in Zenodo (doi:10.5281/zenodo.7641133). The companion web dashboard is hosted by France Bioinformatique ( ).
更多查看译文
AI 理解论文
溯源树
样例
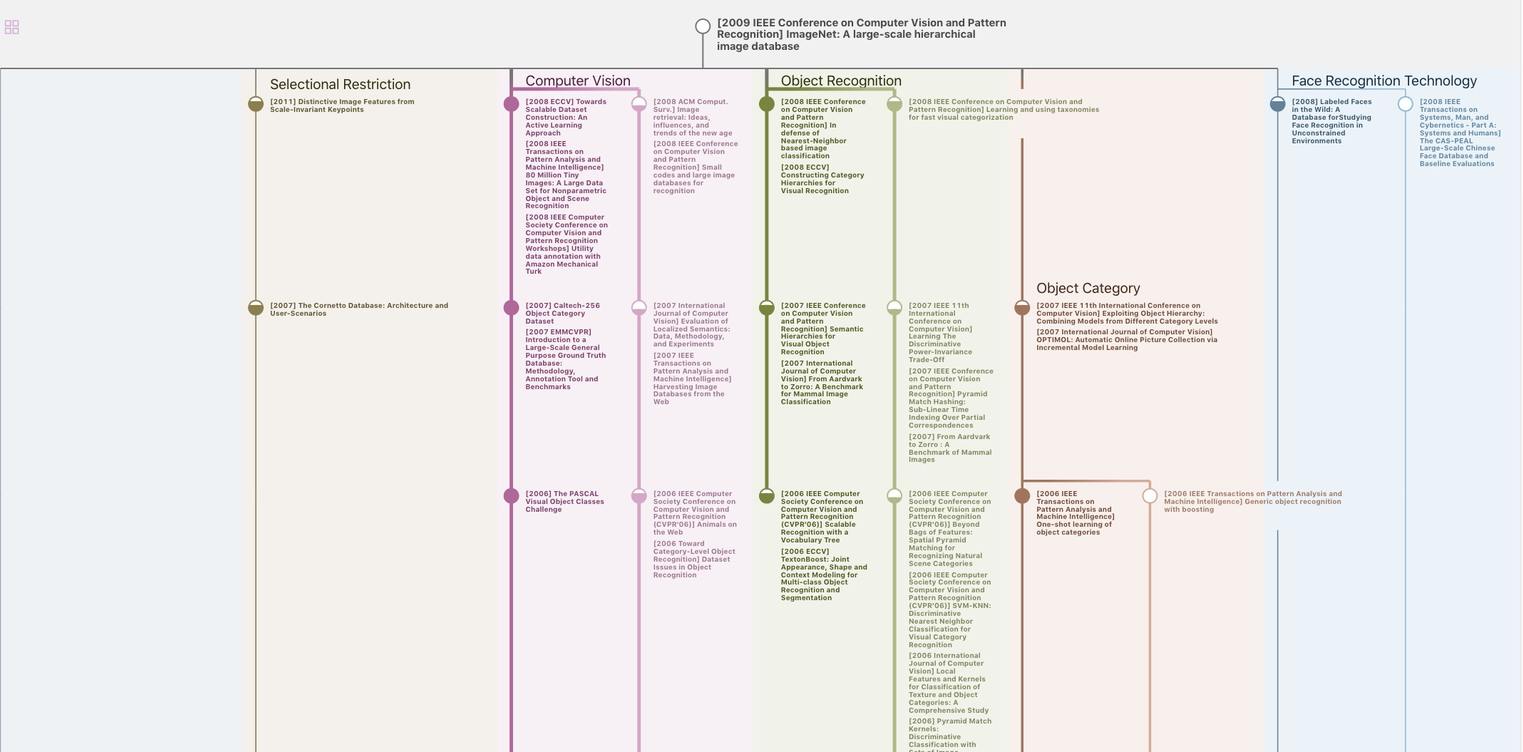
生成溯源树,研究论文发展脉络
Chat Paper
正在生成论文摘要