Generative Editing via Convolutional Obscuring (GECO): A Generative Adversarial Network for MRI de-artifacting
NEUROLOGY(2023)
摘要
Magnetic resonance imaging (MRI) is the dominant diagnostic technique to non-invasively image the brain, and deep learning has proven a powerful tool for analyzing these images. However, machine learning models trained on such MRI data have empirically shown an ability to detect complex and invisible artifacts, such as which type of machine a scan was taken from to a high degree of accuracy. Such artifacts are potentially invisible to the human eye, but can be identified by machine learning systems, leading them to focus on irrelevant features rather than scientifically and/or medically useful ones. For example, machine learning systems can often “shortcut” past the actual features researchers would like to detect and utilize separate spurious correlations to make predictions. Several such undesired features have been reported to interfere with cross-institutional medical imaging deep learning research, and more are likely to be identified as time goes on. Here, we develop a method capable of removing these spurious correlations in an unsupervised manner, leveraging generative techniques to produce images which maintain image quality while learning how to remove technical artifacts. Generative Adversarial Networks are a class of deep learning architectures which have shown impressive efficacy in image generation and editing tasks, and our work builds upon this success. Here, we propose Generative Editing via Convolutional Obscuring (GECO), a Generative Adverserial Network for MRI deartifacting. GECO is based on a CycleGAN, a GAN architecture designed for image-to-image translation that is transforming an input image into a new image with one or more desirable properties. By formulating the CycleGAN loss as a two-player game with a regularization term and incentivizing the generator to erase spurious correlations the original image quality can be better preserved. Beginning with classifiers trained on original images to identify images based on artifacts of interest, GECO reduced the classifiers’ ability to detect these spurious correlations from 97% down to a difference which is nearly equal to a classifier making purely random guesses. We also observe over 98% structural similarity between the original and deartifacted images, indicating the preservation of the vast majority of non-spurious information contained in the original images. In addition to solving the known problem of avoiding artifacts from scanner type, this method opens the door to potentially removing many other types of spurious correlations from medical images and other data modalities across many fields.
Software availability [1][1].
### Competing Interest Statement
Michael Zeineh reports receiving funding from GE. All other authors report no conflict of interest.
### Funding Statement
This study was funded by the NIH National Institute of Biomedical Imaging and Bioengineering, and the NIH National Cancer Institute.
### Author Declarations
I confirm all relevant ethical guidelines have been followed, and any necessary IRB and/or ethics committee approvals have been obtained.
Yes
I confirm that all necessary patient/participant consent has been obtained and the appropriate institutional forms have been archived, and that any patient/participant/sample identifiers included were not known to anyone (e.g., hospital staff, patients or participants themselves) outside the research group so cannot be used to identify individuals.
Yes
I understand that all clinical trials and any other prospective interventional studies must be registered with an ICMJE-approved registry, such as ClinicalTrials.gov. I confirm that any such study reported in the manuscript has been registered and the trial registration ID is provided (note: if posting a prospective study registered retrospectively, please provide a statement in the trial ID field explaining why the study was not registered in advance).
Yes
I have followed all appropriate research reporting guidelines and uploaded the relevant EQUATOR Network research reporting checklist(s) and other pertinent material as supplementary files, if applicable.
Yes
All data used are available online at: K. Clark, B. Vendt, K. Smith, J. Freymann, J. Kirby, P. Koppel, S. Moore. S. Phillips, D. Maffitt, M. Pringle, L. Tarbox, F. Prior, Thecancer imaging archive (tcia): maintaining and operating a public information repository. Journal of digital imaging, 26:1045-1057, 12 2013. K.M. Schmainda and M. Prah. Data from brain-tumor-progression. The Cancer Imaging Archive, 2018. N. Pedano,A.E. Flanders, L. Scarpace, T. Mikkelsen, J.M. Eschbacher, B. Hermes, V. Sisneros, J. Barnholtz,and Q .Ostrom. Radiology data from the cancer genome atlas low grade glioma [tcga-lgg] collection. The Cancer Imaging Archive, 2016. Software created is available at: https://github.com/gevaertlab/GECO
[1]: #fn-3
更多查看译文
AI 理解论文
溯源树
样例
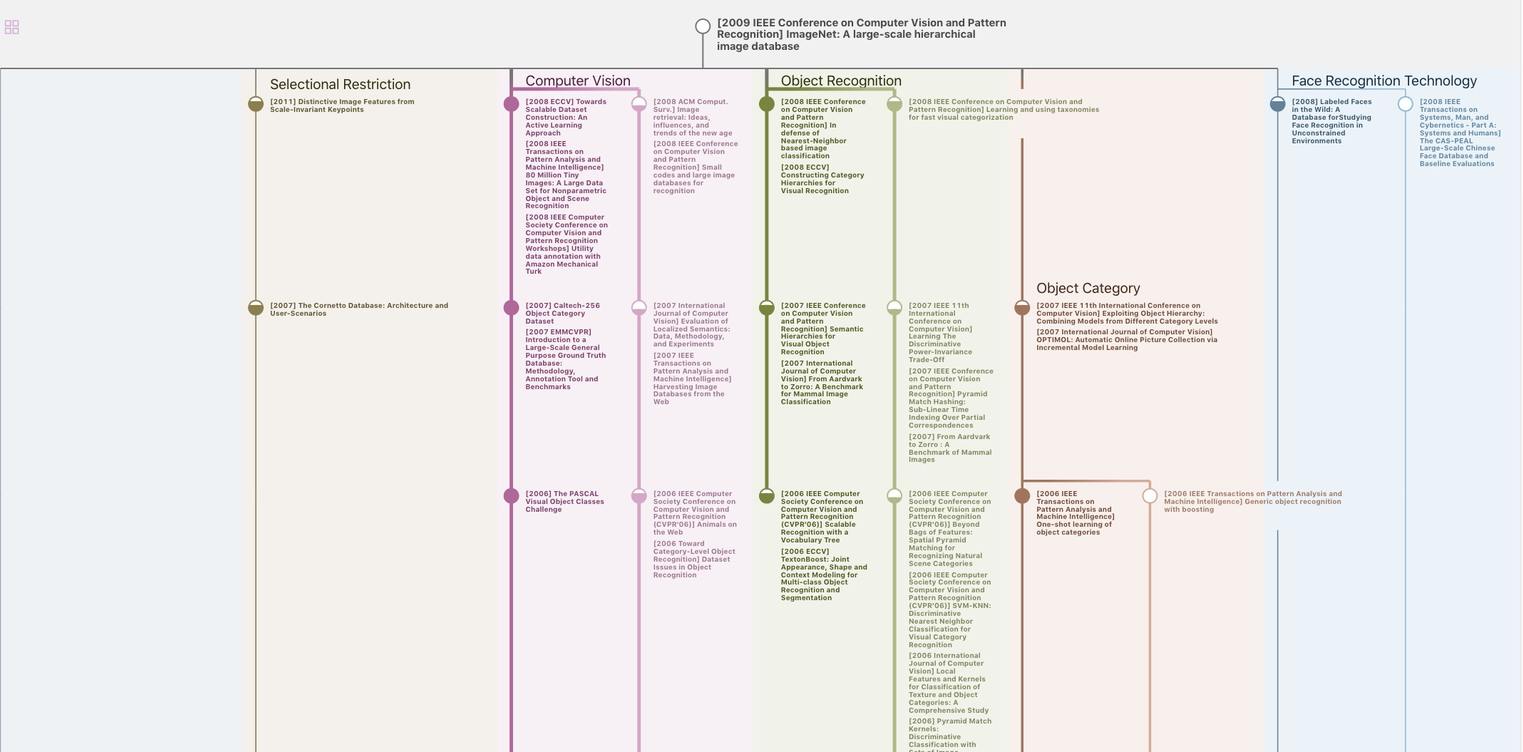
生成溯源树,研究论文发展脉络
Chat Paper
正在生成论文摘要