AI integration improves breast cancer screening in a real-world, retrospective cohort study
medRxiv (Cold Spring Harbor Laboratory)(2022)
摘要
Background Artificial intelligence (AI) readers, derived from applying deep learning models to medical image analysis, hold great promise for improving population breast cancer screening. However, previous evaluations of AI readers for breast cancer screening have mostly been conducted using cancer-enriched cohorts and have lacked assessment of the potential use of AI readers alongside radiologists in multi-reader screening programs. Here, we present a new AI reader for detecting breast cancer from mammograms in a large-scale population screening setting, and a novel analysis of the potential for human-AI reader collaboration in a well-established, high-performing population screening program. We evaluated the performance of our AI reader and AI-integrated screening scenarios using a two-year, real-world, population dataset from Victoria, Australia, a screening program in which two radiologists independently assess each episode and disagreements are arbitrated by a third radiologist.
Methods We used a retrospective full-field digital mammography image and non-image dataset comprising 808,318 episodes, 577,576 clients and 3,404,326 images in the period 2013 to 2019. Screening episodes from 2016, 2017 and 2018 were sequential population cohorts containing 752,609 episodes, 565,087 clients and 3,169,322 images. The dataset was split by screening date into training, development, and testing sets. All episodes from 2017 and 2018 were allocated to the testing set (509,109 episodes; 3,651 screen-detected cancer episodes). Eight distinct AI models were trained on subsets of the training set (which included a validation set) and combined into our ensemble AI reader. Operating points were selected using the development set. We evaluated our AI reader on our testing set and on external datasets previously unseen by our models.
Findings The AI reader outperformed the mean individual radiologist on this large retrospective testing dataset with an area under the receiver operator characteristic curve of 0.92 (95% CI 0.91, 0.92). The AI reader generalised well across screening round, client demographics, device manufacturer and cancer type, and achieved state-of-the-art performance on external datasets compared to recently published AI readers. Our simulations of AI-integrated screening scenarios demonstrated that a reader-replacement human-AI collaborative system could achieve better sensitivity and specificity (82.6%, 96.1%) compared to the current two-reader consensus system (79.9%, 96.0%), with reduced human reading workload and cost. Our band-pass AI-integrated scenario also enabled both higher sensitivity and specificity (80.6%, 96.2%) with larger reductions in human reading workload and cost.
Interpretation This study demonstrated that human-AI collaboration in a population breast cancer screening program has potential to improve accuracy and lower radiologist workload and costs in real world screening programs. The next stage of validation is to undertake prospective studies that can also assess the effects of the AI systems on human performance and behaviour.
### Competing Interest Statement
Peter Brotchie is an employee of annalise.ai.
### Funding Statement
This study was supported by funding from the Australian Government under the Medical Research Future Fund Grant (MRFAI000090) for the Transforming Breast Cancer Screening with Artificial Intelligence (BRAIx) Project and a National Health and Medical Research Council Investigator Grant (GNT1195595). This study was also supported by a Ramaciotti Health Investment Grant and funding from a Royal Australian and New Zealand College of Radiologists Clinical Research Grant and the St Vincent's Hospital Melbourne Research Endowment Fund. The funders had no role in the work or decision to publish.
### Author Declarations
I confirm all relevant ethical guidelines have been followed, and any necessary IRB and/or ethics committee approvals have been obtained.
Yes
The details of the IRB/oversight body that provided approval or exemption for the research described are given below:
Ethics Committee of St Vincents Hospital Melbourne gave ethical approval LNR/18/SVHM/162 for this work
I confirm that all necessary patient/participant consent has been obtained and the appropriate institutional forms have been archived, and that any patient/participant/sample identifiers included were not known to anyone (e.g., hospital staff, patients or participants themselves) outside the research group so cannot be used to identify individuals.
Yes
I understand that all clinical trials and any other prospective interventional studies must be registered with an ICMJE-approved registry, such as ClinicalTrials.gov. I confirm that any such study reported in the manuscript has been registered and the trial registration ID is provided (note: if posting a prospective study registered retrospectively, please provide a statement in the trial ID field explaining why the study was not registered in advance).
Yes
I have followed all appropriate research reporting guidelines and uploaded the relevant EQUATOR Network research reporting checklist(s) and other pertinent material as supplementary files, if applicable.
Yes
The non-transformed image and non-image data that established the ADMANI datasets used in the present study were accessed under license agreement with BreastScreen Victoria. Data availability is subject to agreement with BreastScreen Victoria.
更多查看译文
关键词
ai,screening,breast cancer,cohort study,real-world
AI 理解论文
溯源树
样例
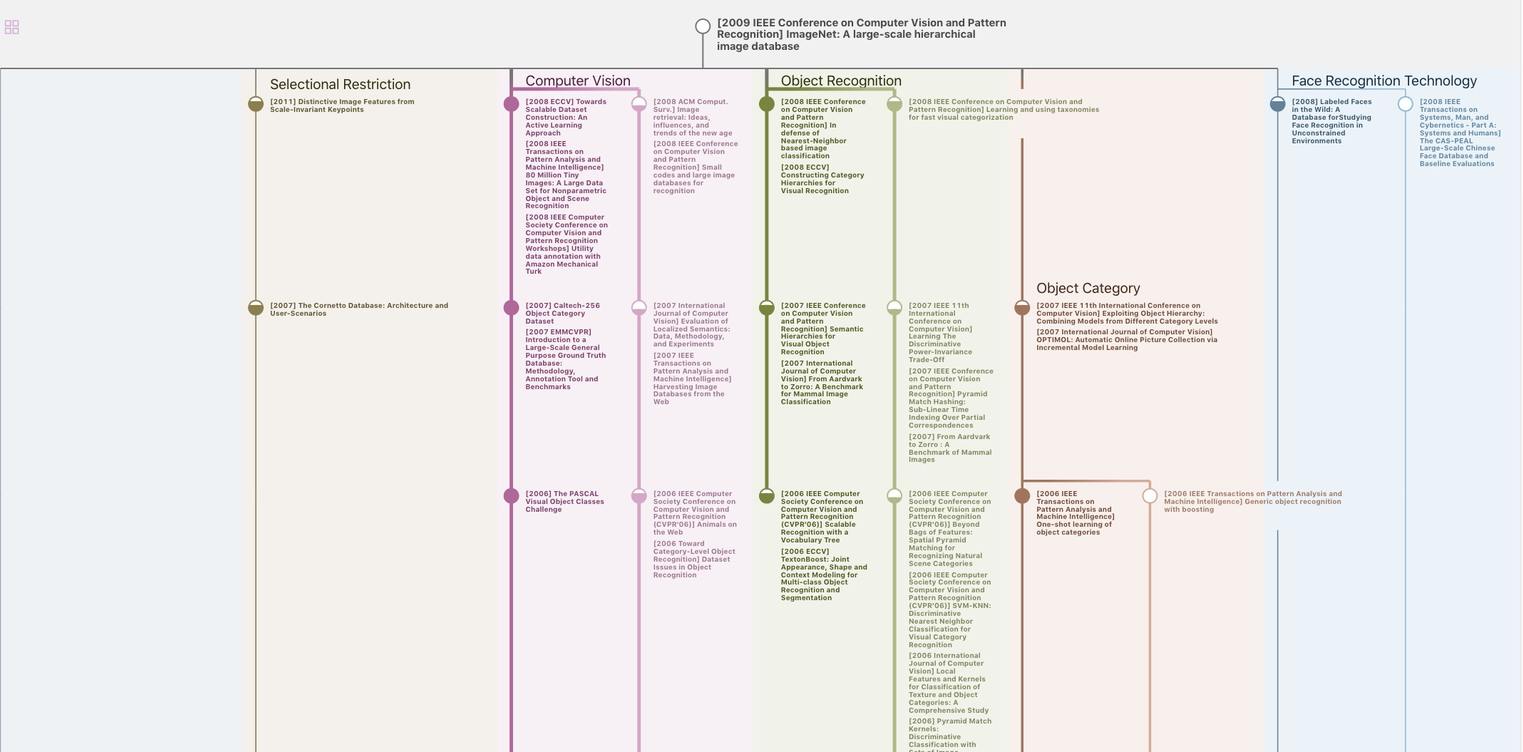
生成溯源树,研究论文发展脉络
Chat Paper
正在生成论文摘要