DETECT: Feature extraction method for disease trajectory modeling
AMIA Joint Summits on Translational Science proceedings. AMIA Joint Summits on Translational Science(2022)
摘要
Modeling with longitudinal electronic health record (EHR) data proves challenging given the high dimensionality, redundancy, and noise captured in EHR. In order to improve precision medicine strategies and identify predictors of disease risk in advance, evaluating meaningful patient disease trajectories is essential. In this study, we develop the algorithm D iseas E T rajectory f E ature extra CT ion ( DETECT) for feature extraction and trajectory generation in high-throughput temporal EHR data. This algorithm can 1) simulate longitudinal individual-level EHR data, specified to user parameters of scale, complexity, and noise and 2) use a convergent relative risk framework to test intermediate codes occurring between a specified index code(s) and outcome code(s) to determine if they are predictive features of the outcome. We benchmarked our method on simulated data and generated real-world disease trajectories using DETECT in a cohort of 145,575 individuals diagnosed with hypertension in Penn Medicine EHR for severe cardiometabolic outcomes.
### Competing Interest Statement
The authors have declared no competing interest.
### Funding Statement
MDR is supported by R01HL141232, R01GM138597, and UL1-TR-001878. AV and DK are supported by NIGMS R01-GM138597. PS is is supported by F31 AG069441-01
### Author Declarations
I confirm all relevant ethical guidelines have been followed, and any necessary IRB and/or ethics committee approvals have been obtained.
Yes
The details of the IRB/oversight body that provided approval or exemption for the research described are given below:
Institutional Review Board of the University of Pennsylvania gave ethical approval for this work through IRB Protocol #829861. The project qualified as exempt.
I confirm that all necessary patient/participant consent has been obtained and the appropriate institutional forms have been archived, and that any patient/participant/sample identifiers included were not known to anyone (e.g., hospital staff, patients or participants themselves) outside the research group so cannot be used to identify individuals.
Yes
I understand that all clinical trials and any other prospective interventional studies must be registered with an ICMJE-approved registry, such as ClinicalTrials.gov. I confirm that any such study reported in the manuscript has been registered and the trial registration ID is provided (note: if posting a prospective study registered retrospectively, please provide a statement in the trial ID field explaining why the study was not registered in advance).
Yes
I have followed all appropriate research reporting guidelines and uploaded the relevant EQUATOR Network research reporting checklist(s) and other pertinent material as supplementary files, if applicable.
Yes
All data produced in the present work are contained in the manuscript.
更多查看译文
关键词
feature extraction method,trajectory,modeling,disease
AI 理解论文
溯源树
样例
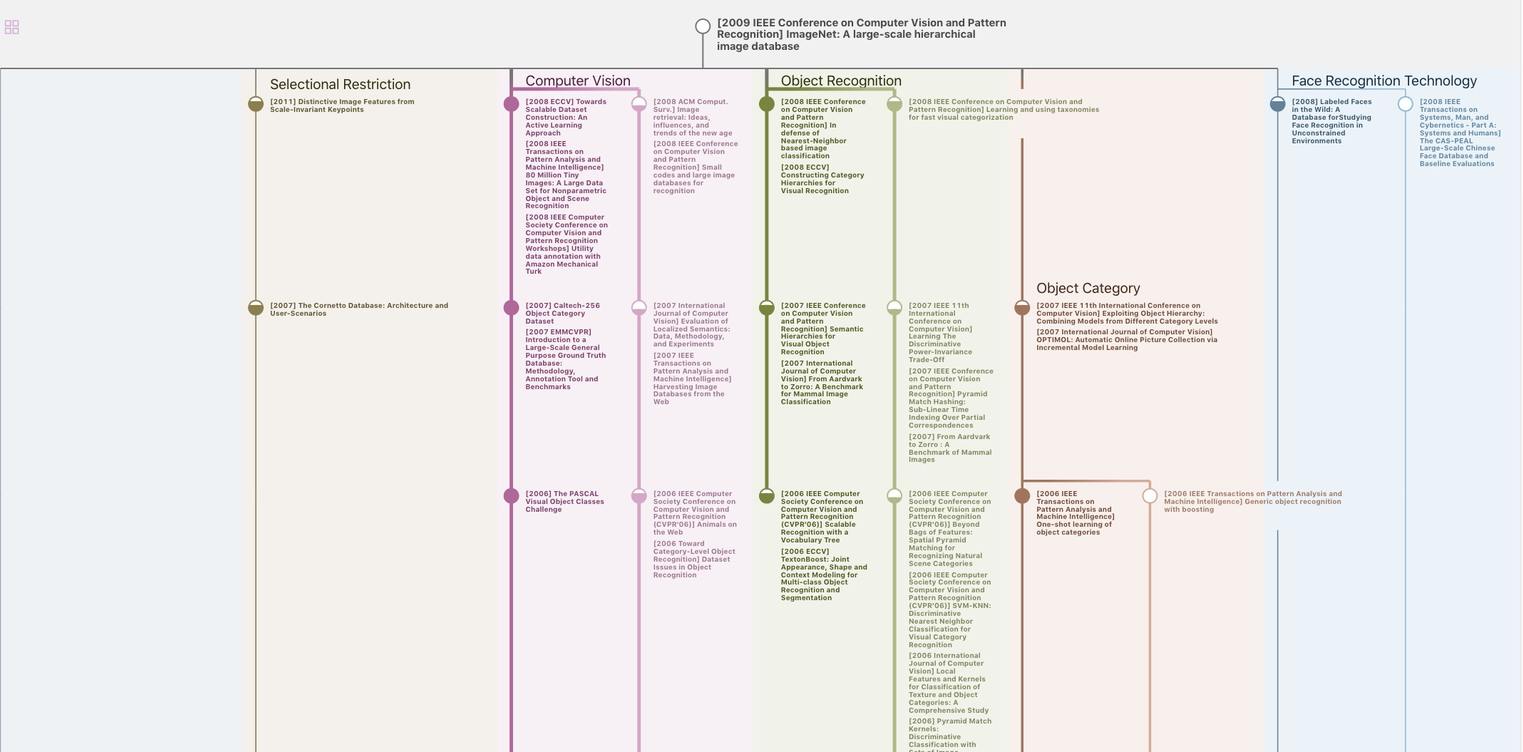
生成溯源树,研究论文发展脉络
Chat Paper
正在生成论文摘要