Multiple imputation of missing data under missing at random: compatible imputation models are not sufficient to avoid bias
Journal of clinical epidemiology(2022)
摘要
Background Epidemiological studies often have missing data. Multiple imputation (MI) is a commonly-used strategy for such studies. MI guidelines for structuring the imputation model have focused on compatibility with the analysis model, but not on the need for the (compatible) imputation model(s) to be correctly specified. Standard (default) MI procedures use simple linear functions. We examine the bias this causes and performance of methods to identify problematic imputation models, providing practical guidance for researchers.
Methods By simulation and real data analysis, we investigated how imputation model mis-specification affected MI performance, comparing results with complete records analysis (CRA). We considered scenarios in which imputation model mis-specification occurred because (i) the analysis model was mis-specified, or (ii) the relationship between exposure and confounder was mis-specified.
Results Mis-specification of the relationship between outcome and exposure, or between exposure and confounder in the imputation model for the exposure, could result in substantial bias in CRA and MI estimates (in addition to any bias in the full-data estimate due to analysis model mis-specification). MI by predictive mean matching could mitigate for model mis-specification. Model mis-specification tests were effective in identifying mis-specified relationships. These could be easily applied in any setting in which CRA was, in principle, valid and data were missing at random (MAR).
Conclusion When using MI methods that assume data are MAR, compatibility between the analysis and imputation models is necessary, but is not sufficient to avoid bias. We propose an easy-to-follow, step-by-step procedure for identifying and correcting mis-specification of imputation models.
### Competing Interest Statement
The authors have declared no competing interest.
### Funding Statement
The results reported herein correspond to specific aims of grant MR/V020641/1 to investigators Kate Tilling and James Carpenter from the UK Medical Research Council. Elinor Curnow, Jon Heron, Rosie Cornish, and Kate Tilling work in the Medical Research Council Integrative Epidemiology Unit at the University of Bristol which is supported by the UK Medical Research Council and the University of Bristol MC\_UU\_00011/3. James Carpenter is also supported by the UK Medical Research Council (grant no MC\_UU\_00004/04).
### Author Declarations
I confirm all relevant ethical guidelines have been followed, and any necessary IRB and/or ethics committee approvals have been obtained.
Yes
The details of the IRB/oversight body that provided approval or exemption for the research described are given below:
Source data were openly available to the public before the initiation of the study. Available at: https://www.ncbi.nlm.nih.gov/pmc/articles/PMC1489946/#S1
I confirm that all necessary patient/participant consent has been obtained and the appropriate institutional forms have been archived, and that any patient/participant/sample identifiers included were not known to anyone (e.g., hospital staff, patients or participants themselves) outside the research group so cannot be used to identify individuals.
Yes
I understand that all clinical trials and any other prospective interventional studies must be registered with an ICMJE-approved registry, such as ClinicalTrials.gov. I confirm that any such study reported in the manuscript has been registered and the trial registration ID is provided (note: if posting a prospective study registered retrospectively, please provide a statement in the trial ID field explaining why the study was not registered in advance).
Yes
I have followed all appropriate research reporting guidelines and uploaded the relevant EQUATOR Network research reporting checklist(s) and other pertinent material as supplementary files, if applicable.
Yes
Stata code to generate and analyse the data as per the simulation study is included in Supplementary Material, Section S11. Stata code to analyse the real data example is included in Supplementary Material, Section S12. The real data are available at: https://www.ncbi.nlm.nih.gov/pmc/articles/PMC1489946/#S1.
更多查看译文
关键词
compatible imputation models,multiple imputation,bias,data,mis-specified
AI 理解论文
溯源树
样例
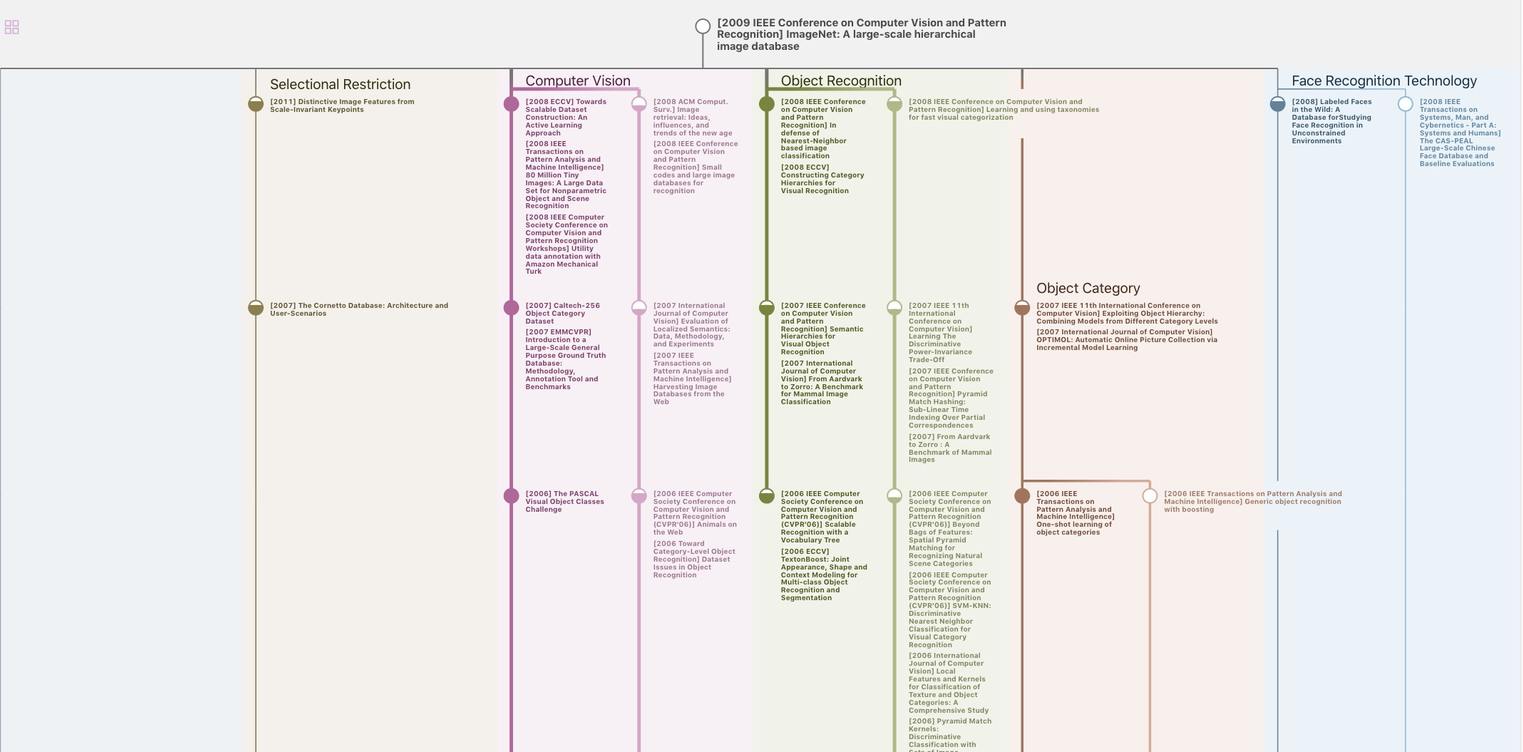
生成溯源树,研究论文发展脉络
Chat Paper
正在生成论文摘要