AMU: Using mRNA Embedding in Self-Attention Network to Predict Melanoma Immune Checkpoint Inhibitor Response
medrxiv(2022)
摘要
Background To precisely predict drug response and avoid unnecessary treatment have been urgent needs to be resolved in the age of melanoma immunotherapy. Deep learning model is a powerful instrument to predict drug response. Simultaneously extracting the function and expression data characteristics of mRNA may help to improve the prediction performance of the model.
Methods We designed a deep learning model named AMU with self-attention structure which were fed with the mRNA expression values for predicting melanoma immune checkpoint inhibitor clinical responses.
Results Comparing with SVM, Random Forest, AdaBoost, XGBoost and the classic convolutional network, AMU showed the preferred performance with the AUC of 0.941 and mAP of 0.960 in validation dataset and AUC of 0.672, mAP of 0.800 in testing dataset, respectively. In model interpretation work, TNF-TNFRSF1A pathway were indicated as a key pathway to influence melanoma immunotherapy responses. Further, gene features extracted from embedding layer and calculated by t-SNE algorithm, showed a local similarity with Functional Protein Association Network (STRING, ), AMU could predict gene functions and interactions simultaneously.
Conclusions Deep learning model built with self-attention structure has strong power to process mRNA expression data and gene vector representation is a promising work in biomedical field.
What is already known on this topic The types of biomarkers for immunotherapy are very complex and transcriptomics biomarker research is one part of it, but currently it is lack of generally acknowledged results with practical value. Combining deep learning models with transcriptomics biomarker markers can help us to predict drug sensitivity. However, the powerful capabilities of deep learning models have not been fully exploited and utilized.
What this study adds The expression of 160 genes could well predict the efficacy of immunotherapy, even if the tissue samples were after drug administration, and through model training, we could also extract the interactions and connections between genes. The deep learning model could not only do prediction, but were also promising in performing gene vector representation learning.
How this study might affect research, practice or policy Our research is not only to provide a model with high predictive value, but also to extract gene interaction relations during model training, which is very enlightening for gene vector representation learning. The research of gene vector representation learning can promote the prediction accuracy of deep learning models in various biomedical fields because it can become the common upstream of many biomedical tasks.
### Competing Interest Statement
The authors have declared no competing interest.
### Funding Statement
This study did not receive any funding
### Author Declarations
I confirm all relevant ethical guidelines have been followed, and any necessary IRB and/or ethics committee approvals have been obtained.
Yes
The details of the IRB/oversight body that provided approval or exemption for the research described are given below:
GSE78220, GSE91061, GSE165278 from GEO Datasets PMID:31792460
I confirm that all necessary patient/participant consent has been obtained and the appropriate institutional forms have been archived, and that any patient/participant/sample identifiers included were not known to anyone (e.g., hospital staff, patients or participants themselves) outside the research group so cannot be used to identify individuals.
Yes
I understand that all clinical trials and any other prospective interventional studies must be registered with an ICMJE-approved registry, such as ClinicalTrials.gov. I confirm that any such study reported in the manuscript has been registered and the trial registration ID is provided (note: if posting a prospective study registered retrospectively, please provide a statement in the trial ID field explaining why the study was not registered in advance).
Yes
I have followed all appropriate research reporting guidelines and uploaded the relevant EQUATOR Network research reporting checklist(s) and other pertinent material as supplementary files, if applicable.
Yes
All data produced in the present study are available upon reasonable request to the authors All data produced in the present work are contained in the manuscript All data produced are available online at
* AI
: Artificial Intelligence
AUC
: The Area Under the Curve
CV
: Computer Vision
CNN
: Convolutional Neural Network
DL
: Deep Learning
GNN
: Graph Neural Network
GO
: Gene Ontology
ICI
: Immune Checkpoint Inhibitor
mAP
: mean Average Precision
NLP
: Natural Language Processing
ORR
: Objective Response Rate
PR
: Precision-Recall
ROC
: receiver operating characteristic curve
SVM
: Support Vector Machine
SHAP
: SHapley Additive Explanations
TMB
: Tumor Mutation Burden
XGBoost
: eXtreme Gradient Boosting
更多查看译文
关键词
mrna,melanoma,immune,self-attention
AI 理解论文
溯源树
样例
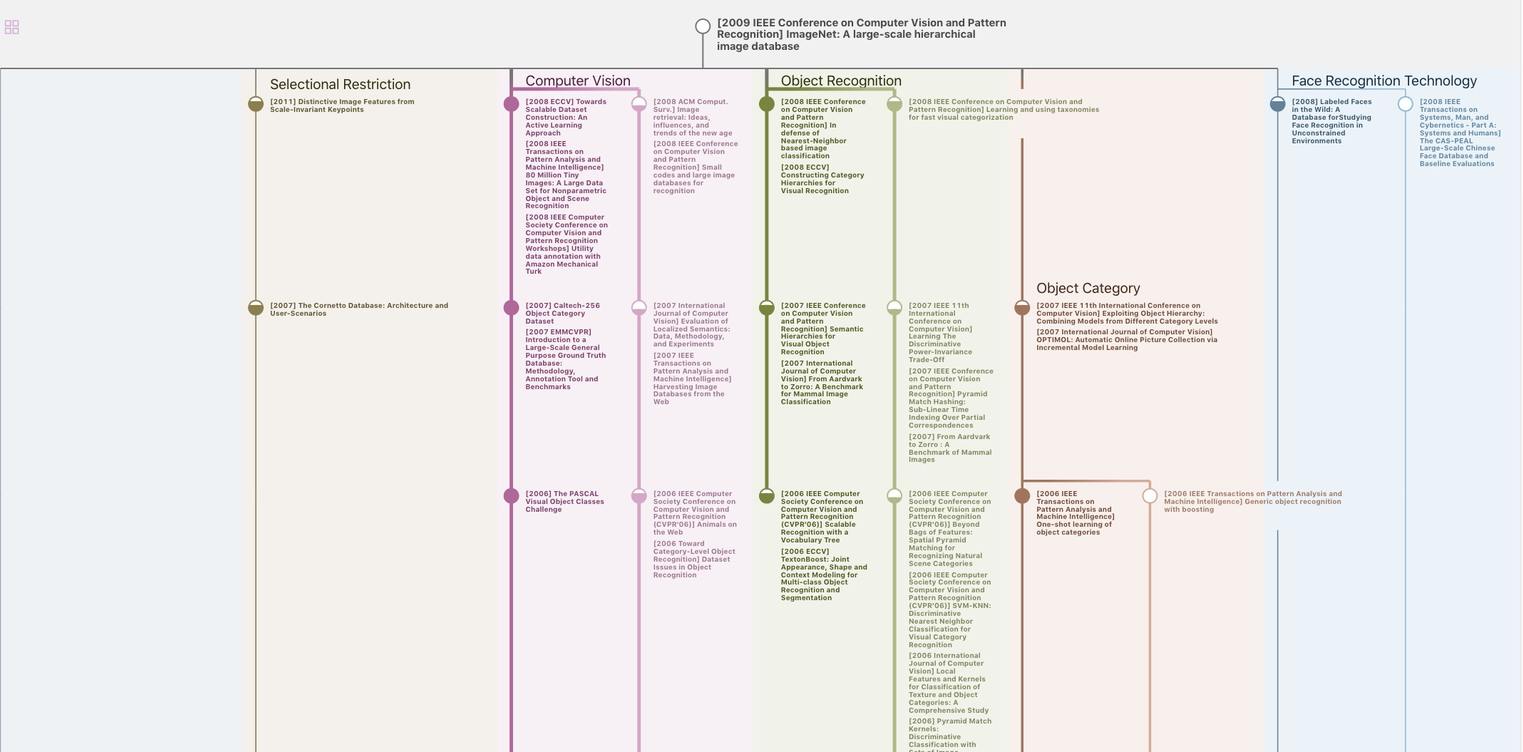
生成溯源树,研究论文发展脉络
Chat Paper
正在生成论文摘要