Long-term fatigue estimation on offshore wind turbines interface loads through loss function physics-guided learning of neural networks
Renewable Energy(2023)
摘要
Offshore wind turbines are exposed during their serviceable lifetime to a wide range of loads from aero-, hydro- and structural dynamics. This complex loading scenario will have an impact on the lifetime of the asset, with fatigue remaining the key structural design driver for the substructure, e.g. the monopile. The ability to monitor the progression of fatigue life of these assets has recently become an operational concern. To achieve a monitoring alternative to strain gauges (cost-prohibitive farm-wide installation), supervisory control and data acquisition (SCADA) systems, often coupled with acceleration measurements, have been used. Existing work focused primarily on ten-minute fatigue load estimation. However, fatigue accumulates over time and the ability to accurately monitor this accumulation of fatigue over a longer time-window is paramount. In this contribution we investigate a novel approach using nine months of real-world SCADA and acceleration ten-minute statistics as inputs of a neural network model for long-term DEM estimation. This is further enhanced by including physical information relative to the problem at hand into the neural network model, in a so-called physics-informed machine learning approach. Specifically, we employ a custom loss function – the Minkowski logarithmic error – which prioritizes conservativeness (over-prediction of fatigue rates) and to embed the damage accumulation into the machine learning model.
更多查看译文
关键词
Offshore wind,Structural health monitoring,Physics-informed machine learning
AI 理解论文
溯源树
样例
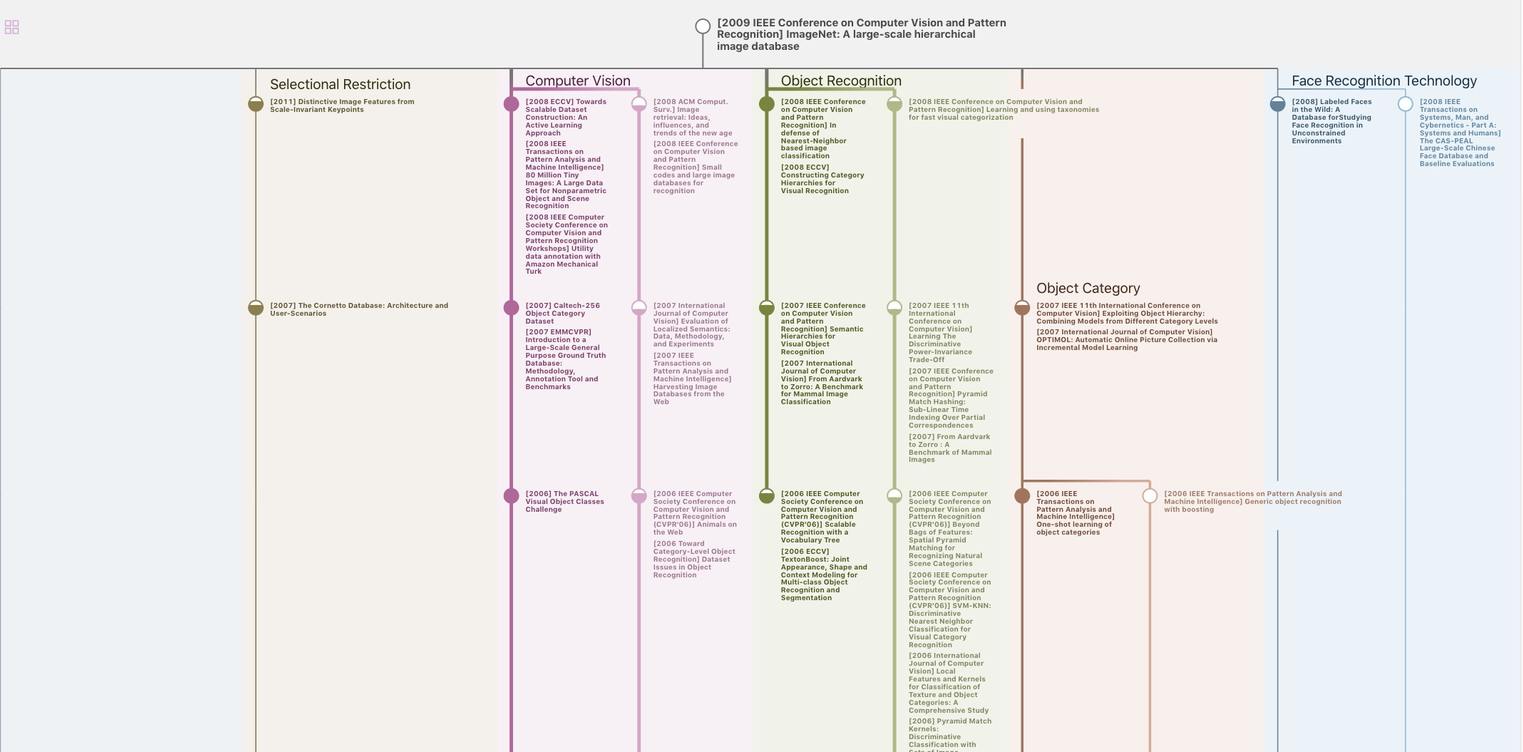
生成溯源树,研究论文发展脉络
Chat Paper
正在生成论文摘要