A fast algorithm to approximate the spectral density of locally tree-like networks with assortativity
Journal of Complex Networks(2023)
摘要
Graphs have become crucial for representing and examining biological, social and technological interactions. In this context, the graph spectrum is an exciting feature to be studied because it encodes the structural and dynamic characteristics of the graph. Hence, it becomes essential to efficiently compute the graph's spectral distribution (eigenvalue's density function). Recently, some authors proposed degree-based methods to obtain the spectral density of locally tree-like networks in linear time. The bottleneck of their approach is that they assumed that the graph's assortativity is zero. However, most real-world networks, such as social and biological networks, present assortativity. Consequently, their spectral density approximations may be inaccurate. Here, we propose a method that considers assortativity. Our algorithm's time and space complexities are
$\mathscr{O}(d_{\max}^{2})$
, where
$d_{\max}$
is the largest degree of the graph. Finally, we show our method's efficacy in simulated and empirical networks.
更多查看译文
关键词
random networks,spectral density,assortativity,locally tree-like networks
AI 理解论文
溯源树
样例
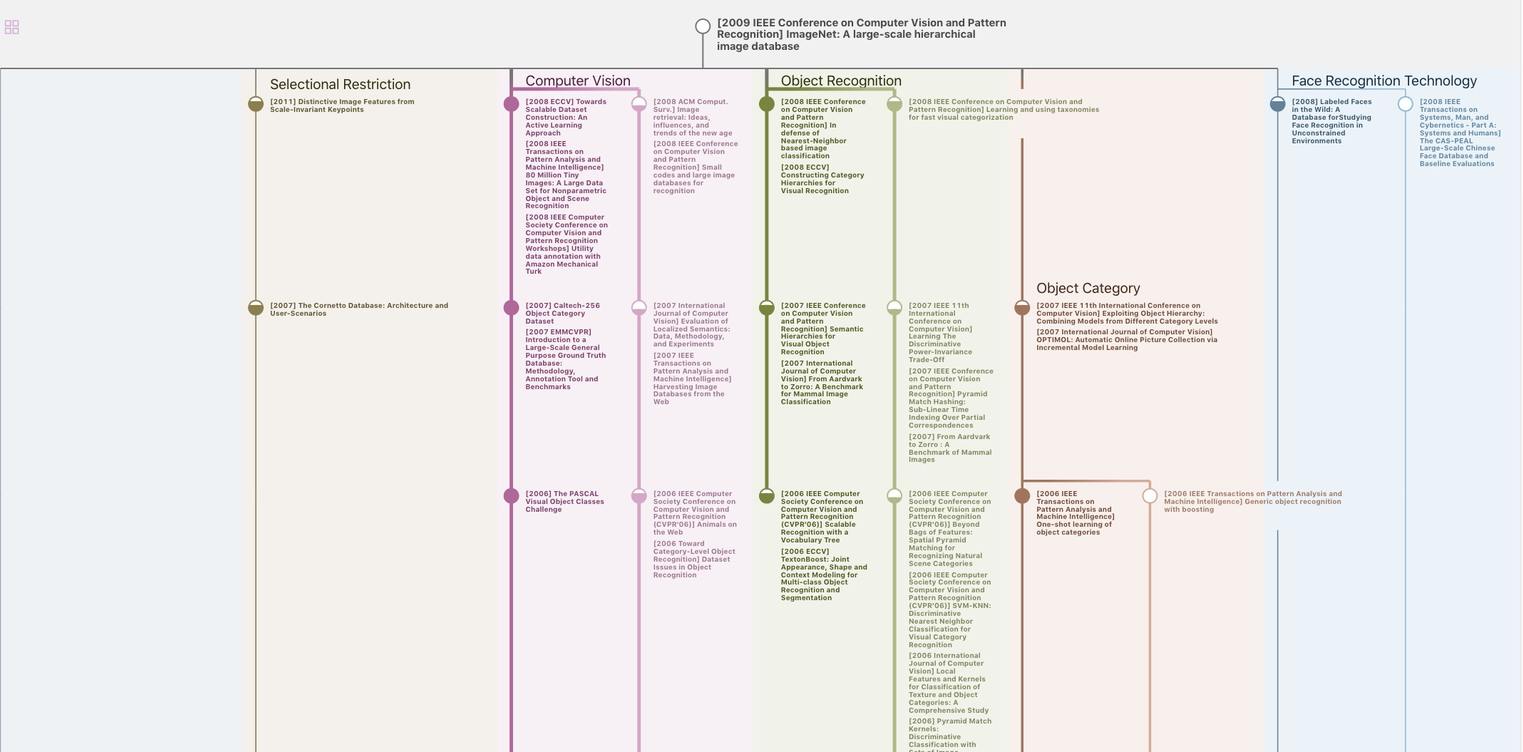
生成溯源树,研究论文发展脉络
Chat Paper
正在生成论文摘要