EEG-Based Multioutput Classification of Sleep Stage and Apnea Using Deep Learning
2023 11th International Winter Conference on Brain-Computer Interface (BCI)(2023)
摘要
Sleep is closely related to physical and mental health and quality of life, and accurately evaluating sleep quality remains a major research topic in related fields. Conventional methods of sleep quality evaluation involve the use of polysomnography (PSG), which continuously records physiological changes during sleep. With the recorded data, sleep quality, and sleep stage or sleep-related disorders can be diagnosed via manual inspection by trained experts. However, the practice is time-consuming, labor-intensive and yields high inter-and intra-rater variability. To overcome such disadvantages, machine learning or deep learning-based automated techniques have been recently proposed. Most of the existing methods, however, can only classify sleep stage or the presence of apnea, not both. This study proposes a novel method that allows simultaneous classification of both sleep stage and apnea, through multioutput classification using a single deep learning model to comprehensively evaluate sleep quality. PSG recordings from a total of 98 subjects from the ISRUC-Sleep dataset subgroup 1 were analyzed in this study, and the proposed model was trained to classify sleep stage and apnea using three EEG channels. The proposed model showed an accuracy and a micro average f1 score of 0.99 for the classification of apnea, and an accuracy and a micro average f1 score of 0.69 for the classification of the sleep stages. The proposed model provides a new paradigm in sleep deep learning research by enabling comprehensive evaluation of sleep quality, hence could help sleep experts to make clinical decisions.
更多查看译文
关键词
Multioutput classification,sleep stage,apnea,deep learning,EEG
AI 理解论文
溯源树
样例
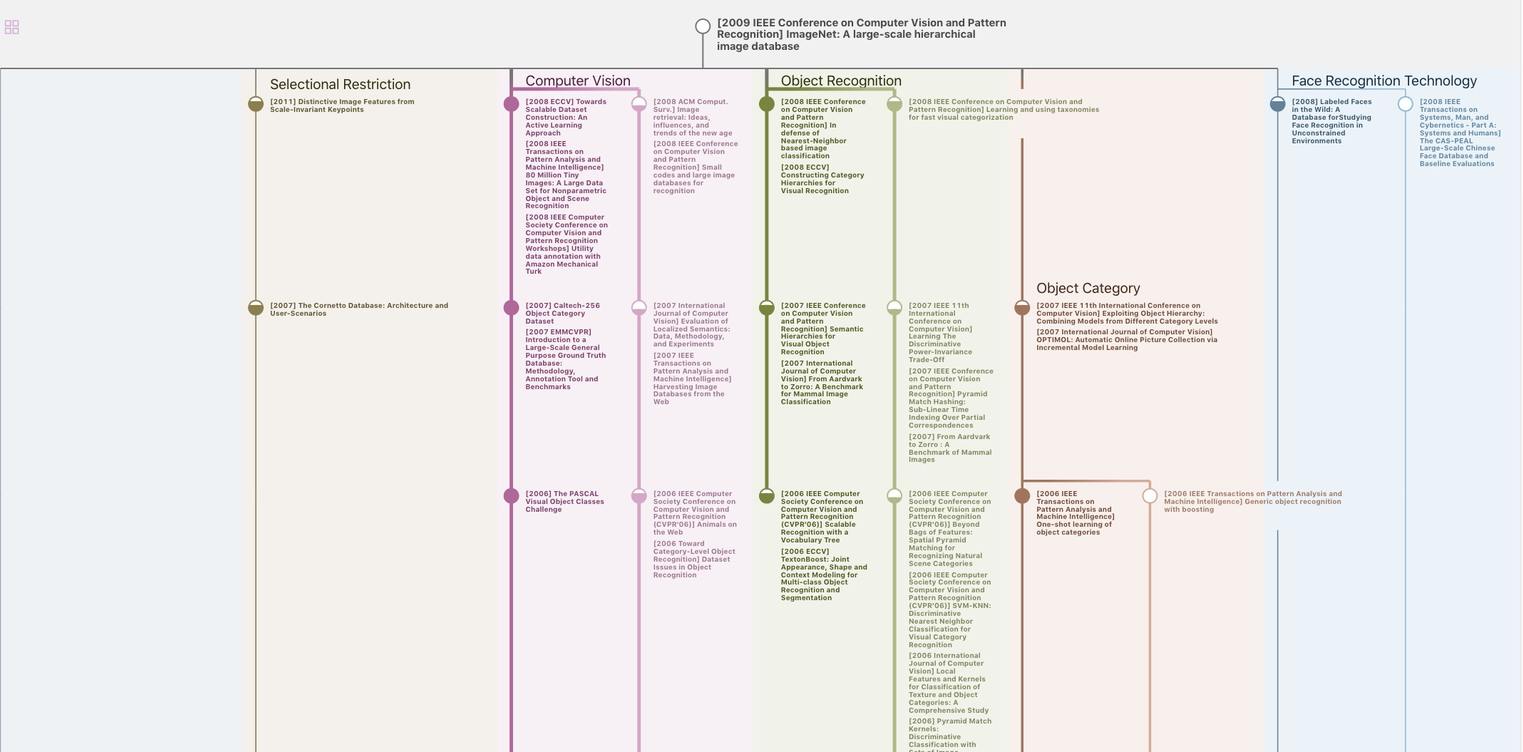
生成溯源树,研究论文发展脉络
Chat Paper
正在生成论文摘要