Efficient and Private Scheduling of Wireless Electric Vehicles Charging Using Reinforcement Learning
IEEE Transactions on Intelligent Transportation Systems(2023)
摘要
Future vehicle-to-grid (V2G) systems require more flexible scheduling to adjust and flatten the peak energy demand. For efficient scheduling and energy trading, the utility provider (UP) needs to keep track of the state of charge (SoC) of vehicle batteries (VBs). However, sharing of SoC of VBs from electric vehicles (EVs) to UP may compromise owner privacy by analyzing the electricity usage in EVs. Therefore, we propose Reinforcement learning (RL)-based demand-side energy management using a rechargeable battery (RB) for enhanced cost-friendly privacy of EVs, efficient scheduling, and accurate billing. With existing Q-Learning-based RL (using
$\epsilon $
-greedy exploration and exploitation), we find that the reward maximization of efficient and private scheduling is often sluggish and incurs convergence issues. Therefore, we develop a genetic algorithm (GA)-based exploration and exploitation, which solves the convergence problems. We develop theoretical analysis and implement numerical results to demonstrate that the proposed GA-based RL framework accelerates convergence and enhances cost-friendly privacy considerably.
更多查看译文
关键词
Electric vehicles (EVs),privacy-cost optimization,reinforcement learning (RL),vehicle to grid (V2G) network
AI 理解论文
溯源树
样例
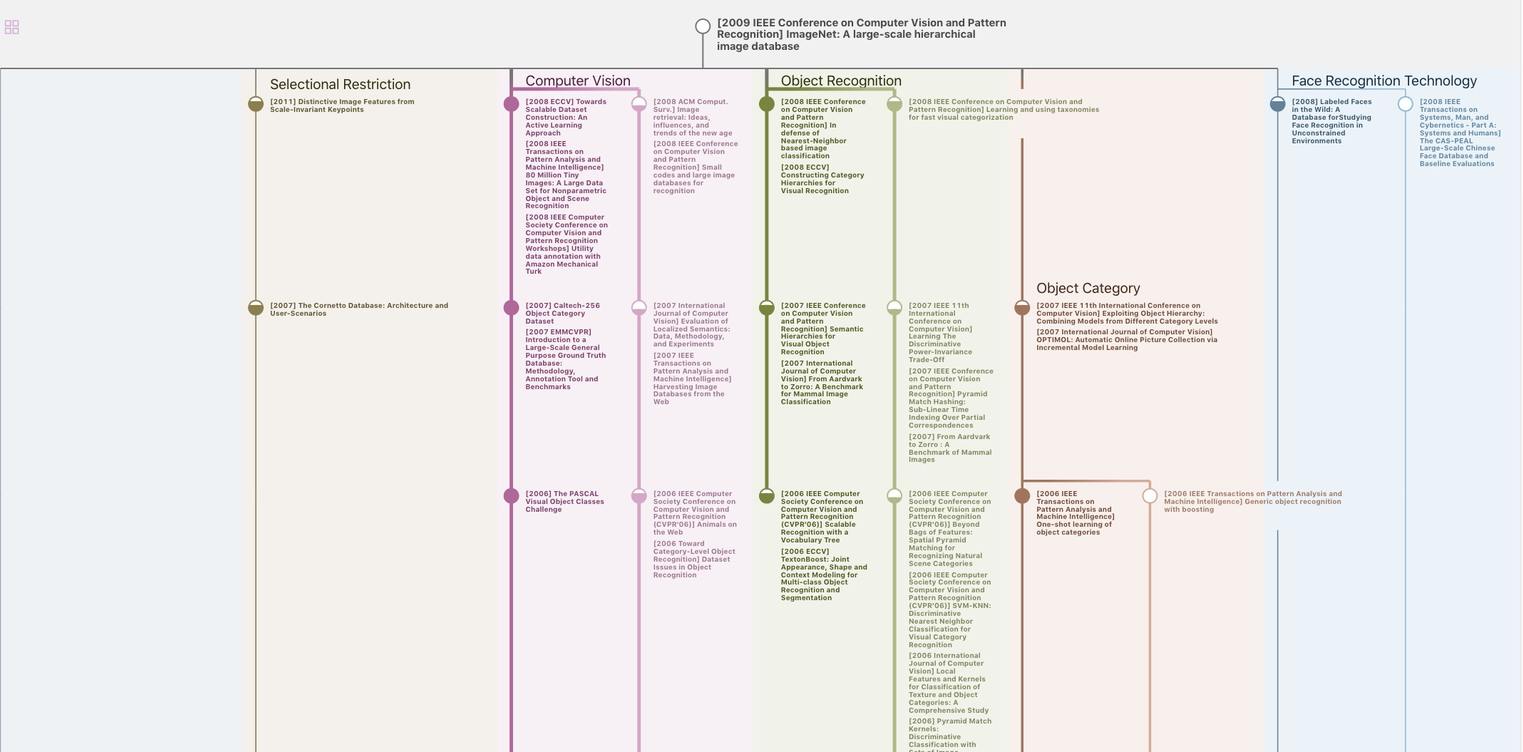
生成溯源树,研究论文发展脉络
Chat Paper
正在生成论文摘要