Edge-assisted Federated Learning in Vehicular Networks
2022 18th International Conference on Mobility, Sensing and Networking (MSN)(2022)
摘要
Given the plethora of sensors with which vehicles are equipped, today's automated vehicles already generate large amounts of data, and this is expected to increase in the case of autonomous vehicles, to enable data-driven solutions for vehicle control, safety and comfort, as well as to effectively implement convenience applications. It is expected that a crucial role in processing such data will be played by machine learning models, which, however, require substantial computing and energy resources for their training. In this paper, we address the use of cooperative learning solutions to train a Neural Network (NN) model while keeping data local to each vehicle involved in the training process. In particular, we focus on Federated Learning (FL) and explore how this cooperative learning scheme can be applied in an urban scenario where several cars, supported by a server located at the edge of the network, collaborate to train a NN model. To this end, we consider an LSTM model for trajectory prediction - a task that is an essential component of many safety and convenience vehicular applications, and investigate the performance of FL as the number of vehicles contributing to the learning process, and the data set they own, vary. To do so, we leverage realistic mobility traces of a large city and the FLOWER FL platform.
更多查看译文
关键词
Connected vehicles,Federated Learning,Edge computing,Performance evaluation
AI 理解论文
溯源树
样例
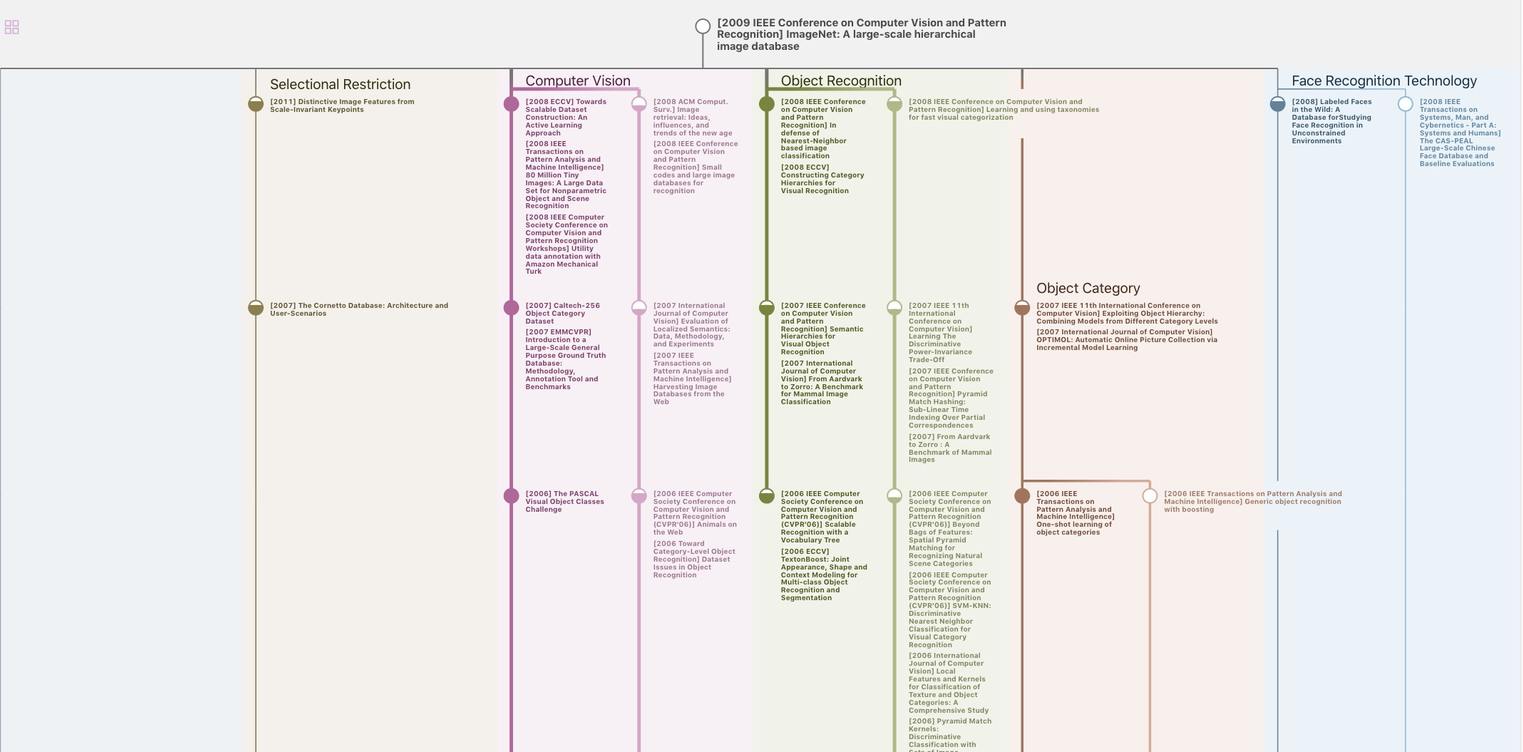
生成溯源树,研究论文发展脉络
Chat Paper
正在生成论文摘要