Nonconvex low-rank tensor approximation with graph and consistent regularizations for multi-view subspace learning
Neural Networks(2023)
摘要
Multi-view clustering is widely used to improve clustering performance. Recently, the subspace clustering tensor learning method based on Markov chain is a crucial branch of multi-view clustering. Tensor learning is commonly used to apply tensor low-rank approximation to represent the relationships between data samples. However, most of the current tensor learning methods have the following shortcomings: the information of the local graph is not taken into account, the relationships between different views are not shown, and the existing tensor low-rank representation takes a biased tensor rank function for estimation. Therefore, a nonconvex low-rank tensor approximation with graph and consistent regularizations (NLRTGC) model is proposed for multi-view subspace learning. NLRTGC retains the local manifold information through graph regularization, and adopts a consistent regularization between multi-views to keep the diagonal block structure of representation matrices. Furthermore, a nonnegative nonconvex low-rank tensor kernel function is used to replace the existing classical tensor nuclear norm via tensor-singular value decomposition (t-SVD), so as to reduce the deviation from rank. Then, an alternating direction method of multipliers (ADMM) which makes the objective function monotonically non-increasing is proposed to solve NLRTGC. Finally, the effectiveness and superiority of the NLRTGC are shown through abundant comparative experiments with various state-of-the-art algorithms on noisy datasets and real world datasets.
更多查看译文
关键词
Multi-view clustering,Subspace clustering,Spectral clustering,Nonconvex low-rank tensor approximation
AI 理解论文
溯源树
样例
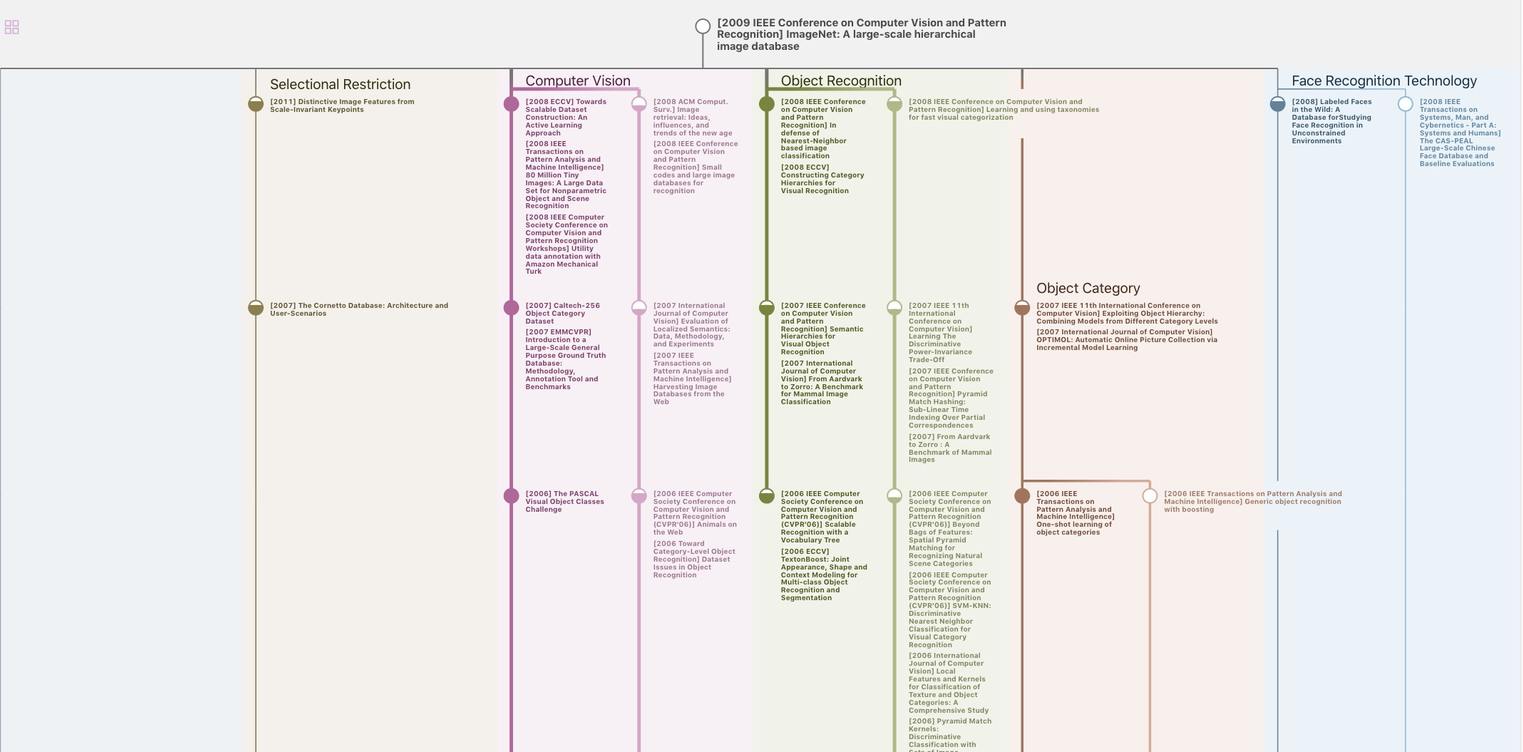
生成溯源树,研究论文发展脉络
Chat Paper
正在生成论文摘要