MPF: A Robust Vehicle Localization Framework Based on Topological Map and Odometry
IEEE Sensors Journal(2023)
摘要
Robust and accurate localization is a mandatory task for autonomous driving. Global positioning method such as global positioning system (GPS) frequently faces signal blocking or city canyon effects in urban area. Dead reckoning sensors such as wheel/visual odometry (VO) or IMU suffer from cumulative drift and require frequent corrections from external sensors. We believe a topological map that is easily available nowadays can be a cheap and effective global sensor for this task. In this article, a flexible topological-map-based probabilistic filtering framework is proposed to tackle the cumulative error problem. Rather than matching the entire trajectory to the map, we prefer extracting sparse anchor points (APs) on the trajectory and producing correcting results for them during filtering. Considering the large uncertainty the trajectory observation contains, our framework is capable of adaptively correcting multiple positions jointly and updating their states only in situations with low uncertainty. This special mechanism explicitly avoids erroneous determination of the position at large uncertain situations such as dense road crossings or roads with small curvature. The experiments carried out on KITTI and our own datasets with both naive single-lane and more advanced multilane topological maps demonstrate that our method can greatly improve the localization accuracy and effectively constrain the cumulative error within a small bound.
更多查看译文
关键词
Anchor points (APs),position correction,probabilistic filtering method,topological road map
AI 理解论文
溯源树
样例
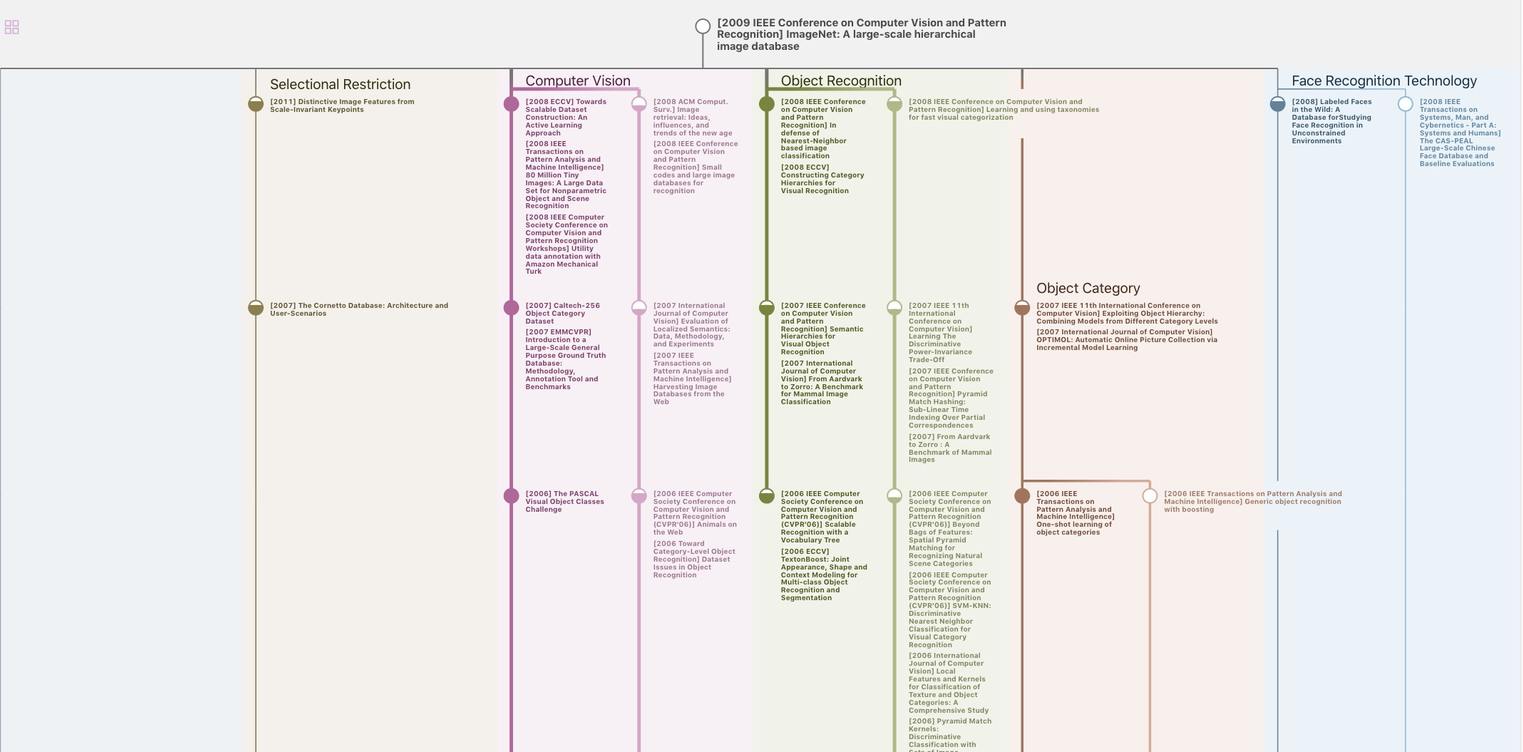
生成溯源树,研究论文发展脉络
Chat Paper
正在生成论文摘要