Space-Time Adaptive Processing Via Random Matrix Theory for Finite Training Samples
IEEE sensors journal(2023)
摘要
Space-time adaptive processing (STAP) is one of the most effective approaches for clutter suppression in airborne radar systems. However, when the number of training samples is less than two times system degrees of freedom (DoFs), the performance of conventional full-dimension (FD)-STAP degrades severely due to inaccurate clutter-plus-noise covariance matrix (CNCM) estimation. In order to enhance the STAP performance under the situation of insufficient training samples, this article proposes a novel STAP method based on the random matrix theory (RMT). According to the spiked covariance model in RMT, we can obtain the estimate of inverse CNCM by determining its eigenvalues, including the noise-related ones and the clutter-related ones from the eigenvalues of sample covariance matrix (SCM) while keeping its eigenvectors consistent with that of SCM. Specifically, the noise-related eigenvalues are directly determined according to the noise power, while the clutter-related eigenvalues are determined by minimizing the output clutter-plus-noise power in terms of RMT. Ultimately, the optimal RMT-STAP weight is derived according to the classical minimum variance distortionless response (MVDR) criterion. Profiting from the great estimation performance on the inverse CNCM, RMT-STAP is significantly superior to other-related FD STAP methods when the number of training samples is less than two times system DoFs. Numerical and real-data experiments verify the effectiveness and robustness of the proposed RMT-STAP method.
更多查看译文
关键词
Eigenvalues and eigenfunctions,Training,Estimation,Covariance matrices,Clutter,Airborne radar,Sensors,Covariance matrix estimation,finite training samples,random matrix theory (RMT),space-time adaptive processing (STAP)
AI 理解论文
溯源树
样例
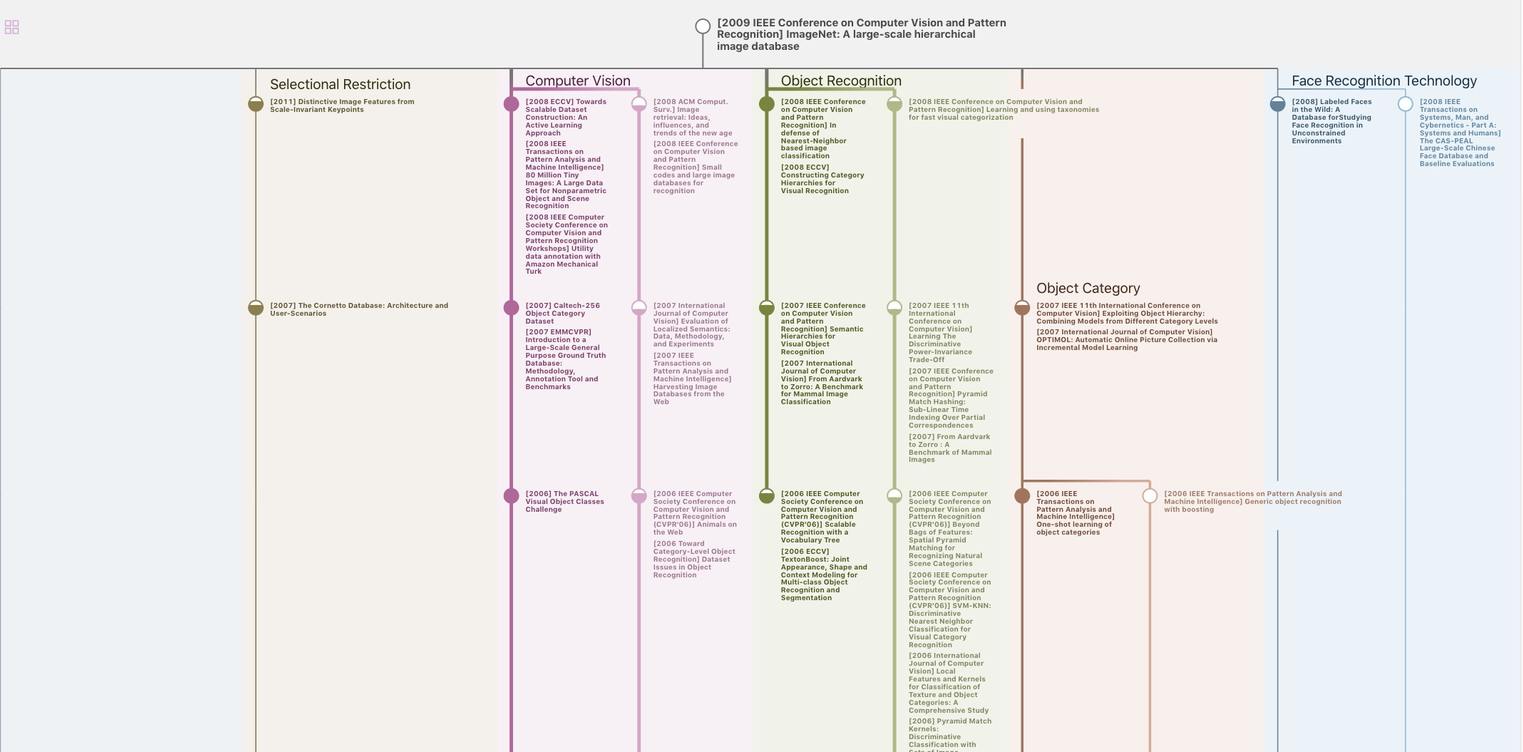
生成溯源树,研究论文发展脉络
Chat Paper
正在生成论文摘要