Graph over-parameterization: Why the graph helps the training of deep graph convolutional network
Neurocomputing(2023)
摘要
Recent studies show that gradient descent can train a deep neural network (DNN) to achieve small train-ing and test errors when the DNN is sufficiently wide. This result applies to various over-parameterized neural network models including fully-connected neural networks and convolutional neural networks. However, existing theory does not apply to graph convolutional networks (GCNs), as GCNs is built according to the topological structures of the data. It has been empirically observed that GCNs can out-perform vanilla neural networks when the underlying graph captures geometric information of the data. However, there is few theoretical justification of such observation. In this paper, we establish theoretical guarantees of the high-probability convergence of gradient descent for training over-parameterized GCNs. Specifically, we introduce a novel measurement of the relation between the graph and the data, called the "graph disparity coefficient", and show that the convergence of GCN is faster when the graph disparity coefficient is smaller. Our analysis provides novel insights into how the graph convolution oper-ation in a GCN helps training, and provides useful guidance for GCN training in practice.(c) 2023 Elsevier B.V. All rights reserved.
更多查看译文
关键词
Graph convolutional neural network,Over -parameterization
AI 理解论文
溯源树
样例
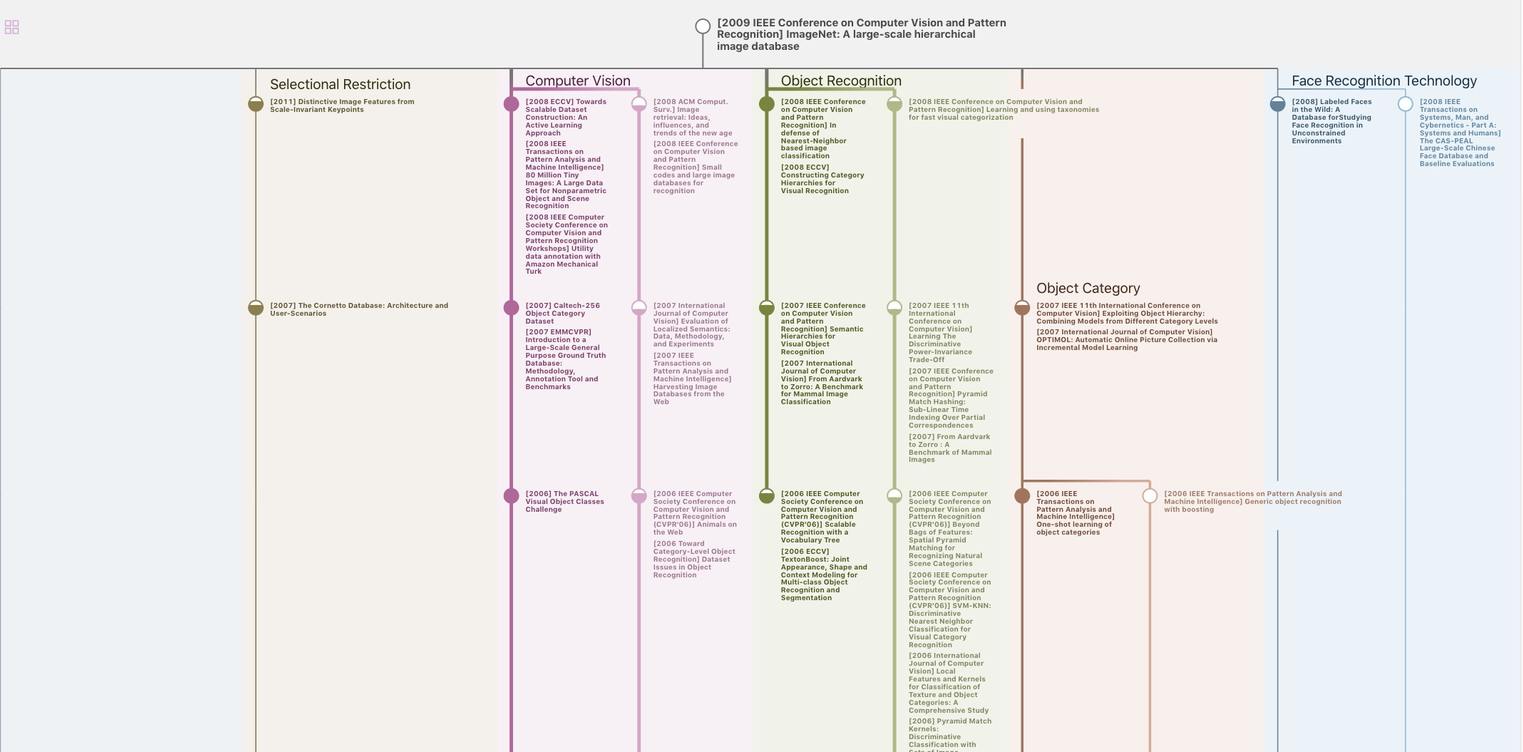
生成溯源树,研究论文发展脉络
Chat Paper
正在生成论文摘要