Plugging the holes in the Swiss Cheese by learning from those who fall through the cracks of our medical system’s multiple lines of defense in Hong Kong: Data-based analytic protocol for identifying the tertiary prevention needs of a population with machine learning pipeline
medrxiv(2022)
摘要
Introduction The objective of tertiary prevention is to reduce re-hospitalization, as re-hospitalization puts patients at unnecessary risk, delays those in the population requiring timely care, and incurs financial burdens on healthcare systems. Nevertheless, it is challenging to stratify the needs of tertiary prevention in a population. Hence, we advance an analytic protocol to identify from an inpatient population the clinical and service-utilization profiles of those re-hospitalized within 28 days.
Methods and analysis The protocol is based on implementing unsupervised and supervised machine learning (ML) in tandem with an inpatient population’s electronic health records. The unsupervised ML will cluster the population into segments of maximized within-segment similarity and between-segment dissimilarity, across the dimensions of clinical diagnoses, acuity, complexity, chronicity, and multimorbidity.
Within each clinically similar segment identified, a 28-day re-hospitalization outcome-supervised decision tree will classify the segment into a series of binarily-split subgroups with profile and service utilization-related features. The order of selected features reflects relative importance to the outcome. Two subgroups originated from a selected feature are statistically different in re-hospitalization outcomes. So, the subgroups lacking selected services while realizing the highest re-hospitalization rates will potentially benefit most from tertiary prevention of the selected services. Thus, they are fit for further assessment and corresponding interventions.
Ethics and dissemination The Survey and Behaviour Research Ethics Committee of the Chinese University of Hong Kong, Hospital Authority Data Collaboration Lab, and a local ethics committee have approved this protocol and its ongoing and forthcoming validations in territory-wide HK through centralized data access and in local clinical management systems, respectively. In addition to disseminating through publications, presentations, and other communications, the protocol is also implementable in different systems as part of the decision support mechanism to inform the venue-based sampling of patients with a high risk of re-hospitalization.
Strength #1 This will be a data-based machine-learning analytic methodology to align population-based cohort study with the conceptual framework of the Swiss Cheese Model for patient safety. The Swiss Cheese Model is a conceptual framework that elevates us from the paradigm of linear causality to conceptualizing safety events as having multiple lines of defense simultaneously broken through. However, the predominant linear model research is incompatible with identifying a medical system’s multiple lines of defense and their potential breakpoints, due to much less prioritizing impacts and mapping interactions on outcomes.
Strength #2 This protocol no long assumes independence among clinical profiles and service utilization-related factors. As a result, the absence and ensembles of post-acute services of the entire population can be handled appropriately.
Strength #3 Subgroups of statistically significant differences in re-hospitalization outcomes will be identified and articulable as a portfolio of clinical profiles and selected services.
Limitations Heterogeneity may still exist after the clustering and classification based on clinical homogeneity. Like all studies based on clinical databases, the heterogeneity can be attributable to exogenous factors absent in clinical information systems, e.g., psychosocial factors, and the narrowly defined tertiary prevention needs of the supervisory outcome.
### Competing Interest Statement
The authors have declared no competing interest.
### Funding Statement
This work was funded by the Strategic Public Policy Research Fund of Policy and Innovation Co-ordinating Office of the Hong Kong Special Administrative Region grant number S2019.A4.015.19S.
### Author Declarations
I confirm all relevant ethical guidelines have been followed, and any necessary IRB and/or ethics committee approvals have been obtained.
Yes
The details of the IRB/oversight body that provided approval or exemption for the research described are given below:
The Survey and Behaviour Research Ethics Committee of the Chinese University of Hong Kong gave ethical approval for this work. The Research Ethics Committees of the Hospital Authority gave ethical approval for this work.
I confirm that all necessary patient/participant consent has been obtained and the appropriate institutional forms have been archived, and that any patient/participant/sample identifiers included were not known to anyone (e.g., hospital staff, patients or participants themselves) outside the research group so cannot be used to identify individuals.
Yes
I understand that all clinical trials and any other prospective interventional studies must be registered with an ICMJE-approved registry, such as ClinicalTrials.gov. I confirm that any such study reported in the manuscript has been registered and the trial registration ID is provided (note: if posting a prospective study registered retrospectively, please provide a statement in the trial ID field explaining why the study was not registered in advance).
Yes
I have followed all appropriate research reporting guidelines and uploaded the relevant EQUATOR Network research reporting checklist(s) and other pertinent material as supplementary files, if applicable.
Yes
The datasets generated and/or analyzed during the current study are not publicly available due to restrictions being put on data sharing by Hong Kong Personal Data (Privacy) Ordinance (Cap. 486) (PDPO), including, but not exclusive to, PDPO Guidance Note in Cross-border Data Transfer. In addition, the Research Ethics Committees of the Hospital Authority do not allow a third-party transfer of patient data. Nor do the Ethics Committees permit study investigators to make public electronic health records of patients of Hospital Authority.
更多查看译文
AI 理解论文
溯源树
样例
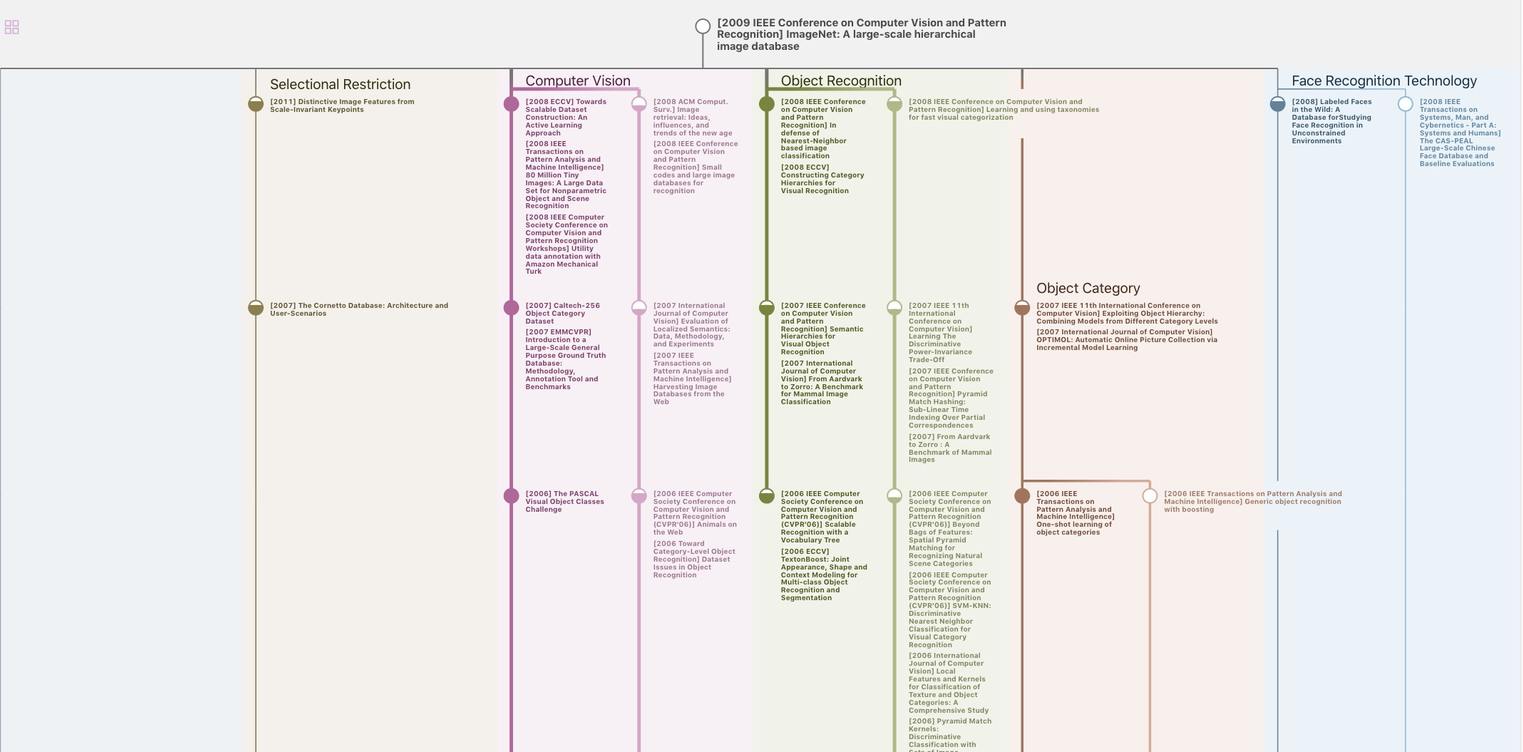
生成溯源树,研究论文发展脉络
Chat Paper
正在生成论文摘要