Comparing supervised machine learning algorithms for the prediction of partial arterial pressure of oxygen during craniotomy
medrxiv(2022)
摘要
Objective To evaluate the feasibility of continuous paO2 prediction in an intraoperative setting among neurosurgical patients with modern machine learning methods.
Materials and Methods Data were extracted from routine clinical care of lung-healthy, neurosurgical patients. We used recursive feature elimination to identify relevant features for the prediction of paO2. Five machine learning algorithms (gradient boosting regressor, k-nearest neighbors regressor, random forest regressor, support vector regression, multi-layer perceptron regressor) and a multivariate linear regression were then tuned and fitted to the selected features. A performance matrix consisting of Spearman’s ρ, mean absolute percentage error (MAPE) and root mean squared error (RMSE) was finally computed based on the test set and used to compare and rank each algorithm.
Results We analyzed 4,180 patients with 12,497 observations. A total of 20 features were selected from analysis of the training dataset comprising 836 patients with 9,992 observations. The best algorithm, random forest, was able to predict paO2 values with ρ=0.90, MAPE=10.4%, and RMSE=30.9mmHg, closely followed by gradient boosting and multi-layer perceptron. Support vector regression, k-nearest neighbors regressor and the linear regression did not achieve the performance metrics.
Discussion We successfully applied and compared several machine learning algorithms to estimate continuous paO2 values in neurosurgical patients. The random forest regressor performed best over all three categories of the performance matrix.
Conclusion PaO2 can be predicted by perioperative routine data in neurosurgical patients.
### Competing Interest Statement
The authors have declared no competing interest.
### Funding Statement
This study did not receive any funding.
### Author Declarations
I confirm all relevant ethical guidelines have been followed, and any necessary IRB and/or ethics committee approvals have been obtained.
Yes
The details of the IRB/oversight body that provided approval or exemption for the research described are given below:
The study was conducted as a single center retrospective cohort study. The University of Munichs institutional review board approved our protocol (submission 19-539) prior to data access. Due to the retrospective design and anonymization, written consent was not necessary.
I confirm that all necessary patient/participant consent has been obtained and the appropriate institutional forms have been archived, and that any patient/participant/sample identifiers included were not known to anyone (e.g., hospital staff, patients or participants themselves) outside the research group so cannot be used to identify individuals.
Yes
I understand that all clinical trials and any other prospective interventional studies must be registered with an ICMJE-approved registry, such as ClinicalTrials.gov. I confirm that any such study reported in the manuscript has been registered and the trial registration ID is provided (note: if posting a prospective study registered retrospectively, please provide a statement in the trial ID field explaining why the study was not registered in advance).
Yes
I have followed all appropriate research reporting guidelines and uploaded the relevant EQUATOR Network research reporting checklist(s) and other pertinent material as supplementary files, if applicable.
Yes
All data produced in the present study are available upon reasonable request to the authors.
* ABBREVIATIONS
:
ABG
: Arterial Blood Gas
CI
: Confidence Interval
diffMSE
: MSE Difference
GBR
: Gradient Boosting Regressor
IQR
: Interquartile Range
KNN Regressor
: K-nearest Neighbors Regressor
MLR
: Multivariate Linear Regression
MAPE
: Mean Absolute Percentage Error
MLP Regressor
: Multi-layer Perceptron Regressor
MLR
: Multivariate Linear Regression
MSE
: Mean Squared Error
n
: Number of Observations (multiple per surgery)
N
: Number of Surgeries
neg.
: Negative
paO2
: Partial Arterial Pressure of Oxygen
pAO2
: Alveolar Oxygen Partial Pressure
Q1/Q3
: First/Third Quartile
RFR
: Random Forest Regressor
RMSE
: Root Mean Squared Error
SVR
: Support Vector Regression
更多查看译文
关键词
craniotomy,partial arterial pressure,supervised machine,machine learning
AI 理解论文
溯源树
样例
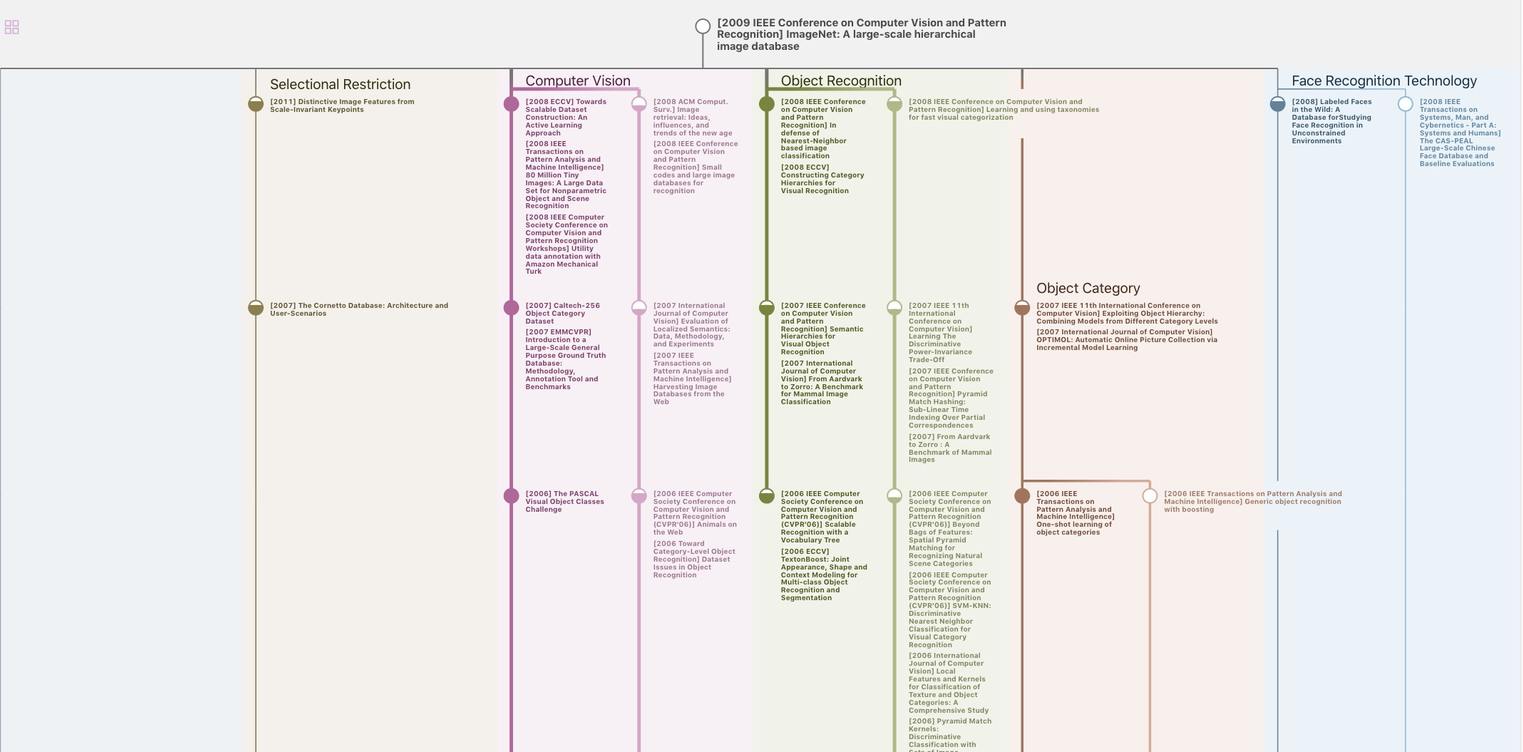
生成溯源树,研究论文发展脉络
Chat Paper
正在生成论文摘要