Byte-Pair Encoding for Classifying Routine Clinical Electroencephalograms in Adults Over the Lifespan
IEEE Journal of Biomedical and Health Informatics(2023)
摘要
Routine clinical EEG is a standard test used for the neurological evaluation of patients. A trained specialist interprets EEG recordings and classifies them into clinical categories. Given time demands and high inter-reader variability, there is an opportunity to facilitate the evaluation process by providing decision support tools that can classify EEG recordings automatically. Classifying clinical EEG is associated with several challenges: classification models are expected to be interpretable; EEGs vary in duration and EEGs are recorded by multiple technicians operating various devices. Our study aimed to test and validate a framework for EEG classification which satisfies these requirements by transforming EEG into unstructured text. We considered a highly heterogeneous and extensive sample of routine clinical EEGs (n = 5785), with a wide range of participants aged between 15 and 99 years. EEG scans were recorded at a public hospital, according to 10/20 electrode positioning with 20 electrodes. The proposed framework was based on symbolizing EEG signals and adapting a previously proposed method from natural language processing (NLP) to break symbols into words. Specifically, we symbolized the multichannel EEG time series and applied a byte-pair encoding (BPE) algorithm to extract a dictionary of the most frequent patterns (tokens) reflecting the variability of EEG waveforms. To demonstrate the performance of our framework, we used newly-reconstructed EEG features to predict patients' biological age with a Random Forest regression model. This age prediction model achieved a mean absolute error of 15.7 years. We also correlated tokens' occurrence frequencies with age. The highest correlations between the frequencies of tokens and age were observed at frontal and occipital EEG channels. Our findings demonstrated the feasibility of applying an NLP-based approach to classifying routine clinical EEG. Notably, the proposed algorithm could be instrumental in classifying clinical EEG with minimal preprocessing and identifying clinically-relevant short events, such as epileptic spikes.
更多查看译文
关键词
Byte-pair encoding,machine learning,EEG classification,clinical electroencephalography,age prediction,natural language processing,multivariate time series
AI 理解论文
溯源树
样例
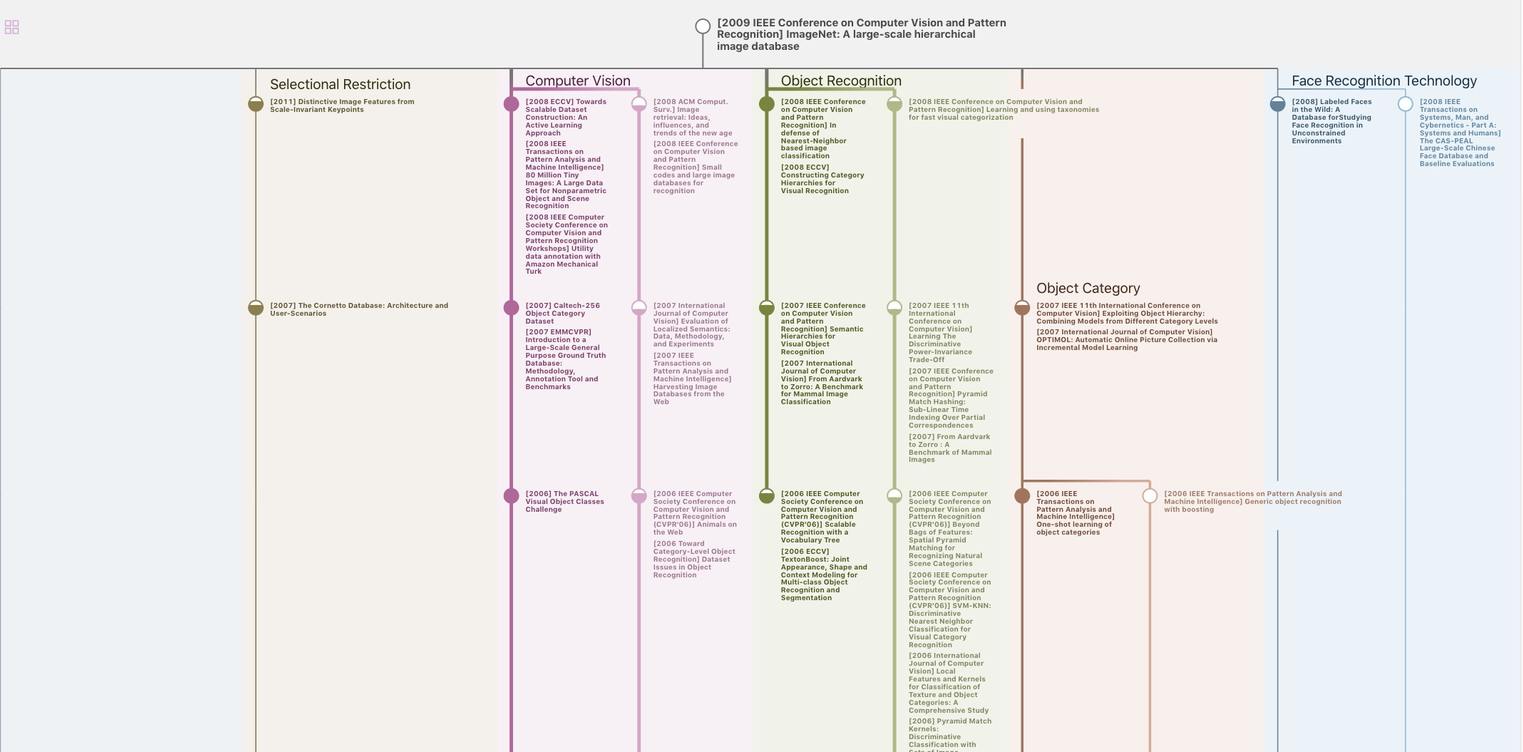
生成溯源树,研究论文发展脉络
Chat Paper
正在生成论文摘要