Off-Policy Reinforcement based on a Safe Model Eco-Driving Education for Fully-Automated, Connected Hybrid Vehicles
2023 Second International Conference on Electronics and Renewable Systems (ICEARS)(2023)
摘要
Eco-driving reduces gas use. Eco-driving improves driving behavior and fuel economy without hardware modifications. Eco-driving strategies exist. Speed control is difficult in many driving conditions. This research develops an eco-driving approach to reduce car emissions in a variety of scenarios. DRL has helped eco-driving reduce fuel usage and travel time. Data-driven reinforcement learning can improve autonomous electric car speed planning. Eco-driving strategy reinforcement learning prototype. Three enhancements are offered. Off-policy learning and physics-based models improve sample efficiency. Second, constraint fulfillment can be achieved without externally rewarding proper behavior during training. Third, deep generative techniques to mimic a safe set ensure the route is possible. The reinforcement learning method can optimize car speed based on highway gradient and vehicle distance. Reinforcement learning outperforms evolutionary algorithms nearly perfectly.
更多查看译文
关键词
Eco-Driving,Electric Vehicle,Deep Reinforcement Learning (DRL),Off Policy-Learning,Fuel Consumption
AI 理解论文
溯源树
样例
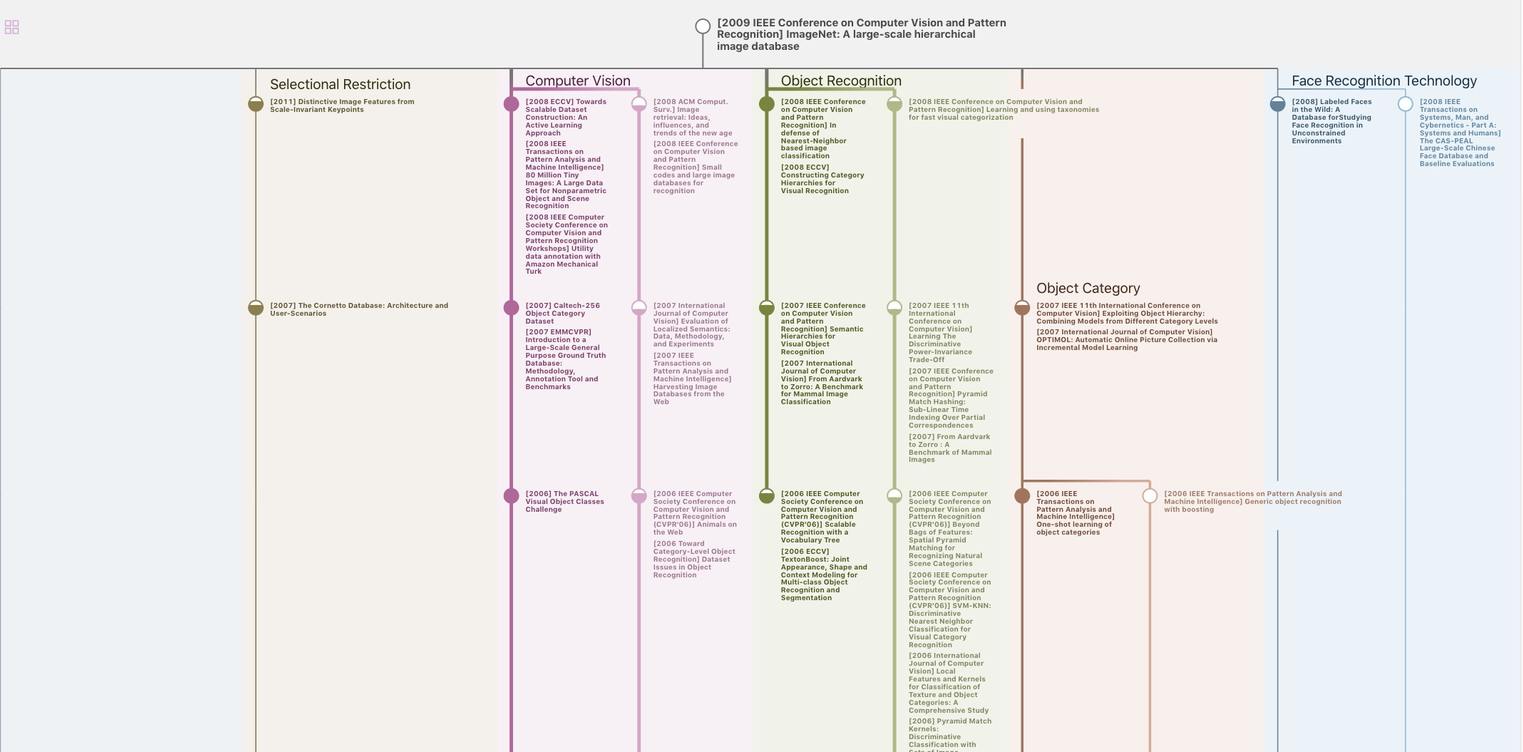
生成溯源树,研究论文发展脉络
Chat Paper
正在生成论文摘要