3D Shape Estimation from RGB Data Using 2.5D Features and Deep Learning
2023 4th International Conference on Advancements in Computational Sciences (ICACS)(2023)
摘要
Creation of 3D models from a single RGB image is challenging problem in image processing these days, as the technology is in its early development stage. However, the demands for 3D technology and 3D reconstruction have been rapidly increasing nowadays. The traditional approach of computer graphics is to create a geometric model in 3D and try to reproduce it onto a 2D image with rendering. The major aim of the study is to create 3D models from 2D RGB image using machine learning techniques to be less computationally complex as compared to any deep learning algorithm. The proposed model has been based on three different modules such as: 2.5D features extraction, mesh generation, and 3D boundary detection. The ShapeNet dataset has been used for comparison. The testing results has shown an accuracy of 90.77 % in the plane class, 85.72% in the chair class, and 72.14% in the automobile class. The proposed model could be applicable to problems where reconstruction of 3D models is required such as: variations in geometric scale, mix of textured, uniformly colored, and reflective surfaces.
更多查看译文
关键词
Machine learning,Data mining,neural network,Big data analytics,3D bounding box
AI 理解论文
溯源树
样例
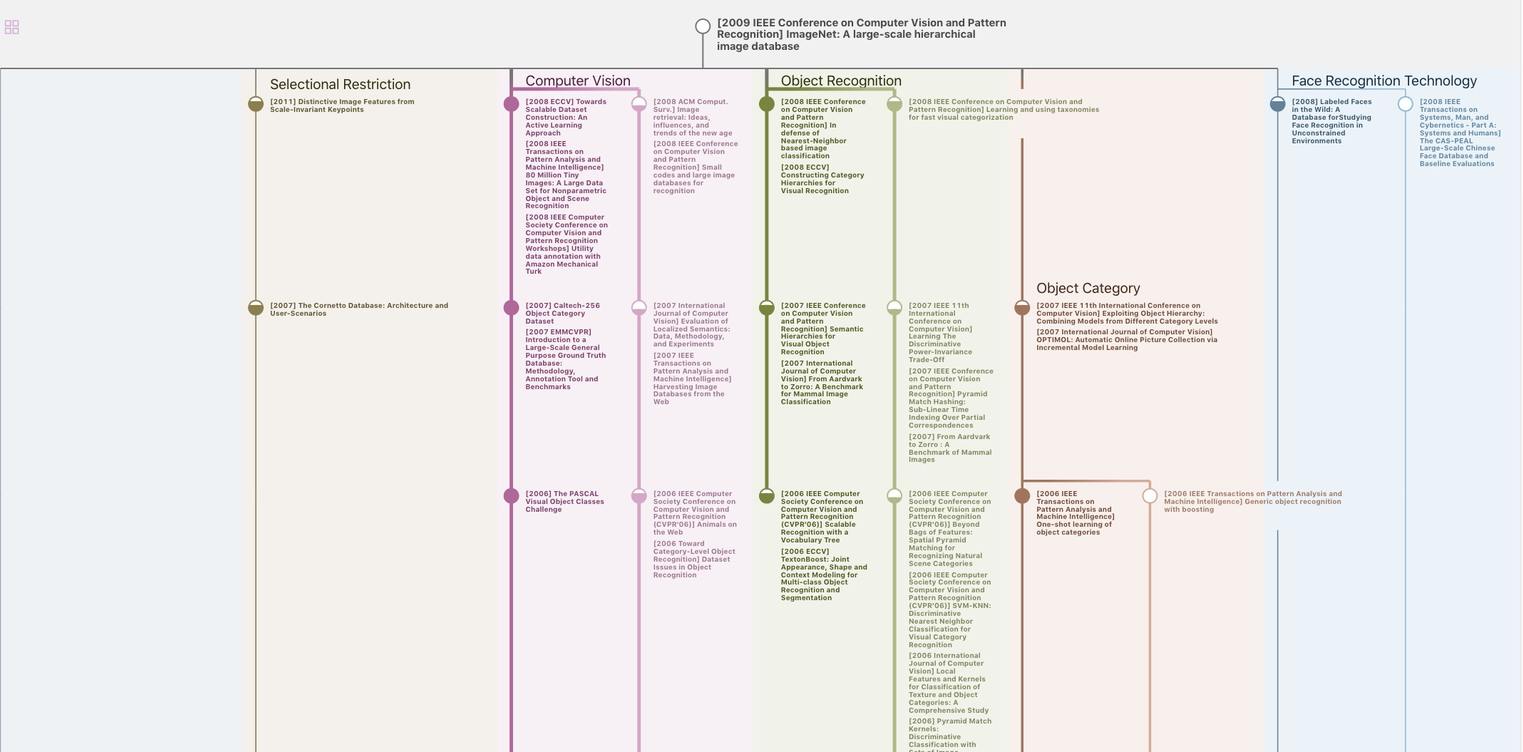
生成溯源树,研究论文发展脉络
Chat Paper
正在生成论文摘要