An Unsupervised Snow Segmentation Approach Based on Dual-Polarized Scattering Mechanism and Deep Neural Network
IEEE Transactions on Geoscience and Remote Sensing(2023)
摘要
Distribution of snow and its melting is a critical factor affecting local weather, avalanche and flood forecasting, livelihood of people residing, and hydropower production. Most of the existing dry and wet snow identification methods were based on expensive quad-pol synthetic aperture radar (SAR) with finite generalizability, while dual-pol SAR with larger coverage, longer time series, and open availability has more advantages. In this study, an unsupervised algorithm for dry and wet snow discrimination, neighborhood-based sparse autoencoder (NSAE)-weighted fuzzy C-means clustering (WFCM), is proposed based on a variety of polarimetric features derived from the
$H$
–
$\alpha $
decomposition in the dual-pol mode using the C-band Sentinel-1 SAR data. NSAE-WFCM constructs a deep training network using the pixel NSAE to optimize polarimetric parameters and inputs reconstructed features with different weights into feature-WFCM to distinguish dry and wet snow for each underlying surface. Ground observation was carried out during the snow melting period of March 2021 in Altay, China, to validate the dual-pol NSAE-WFCM method with an overall accuracy and a Kappa coefficient of 88.8% and 0.68, respectively. The results show that NSAE-WFCM’s accuracy is similar to that of the quad-pol SAR-based dry and wet snow result (90.0%) and significantly better than that of previously published approaches extended to dual-pol SAR, such as support vector machine (SVM) (76.7%), H–
$\alpha $
-Wishart (65.5%), total power-based method (51.7%), and wet snow-based method (43.1%). Therefore, the NSAE-WFCM algorithm improves the ability to classify wet and dry snow based on dual-pol polarimetric features, overcomes the high dependence of existing methods on quad-pol SAR data, and reduces manual interpretation by using unsupervised clustering.
更多查看译文
关键词
Dry and wet snow,feature-weighted fuzzy {C-means} (WFCM),polarimetric decomposition,sparse autoencoder (SAE)
AI 理解论文
溯源树
样例
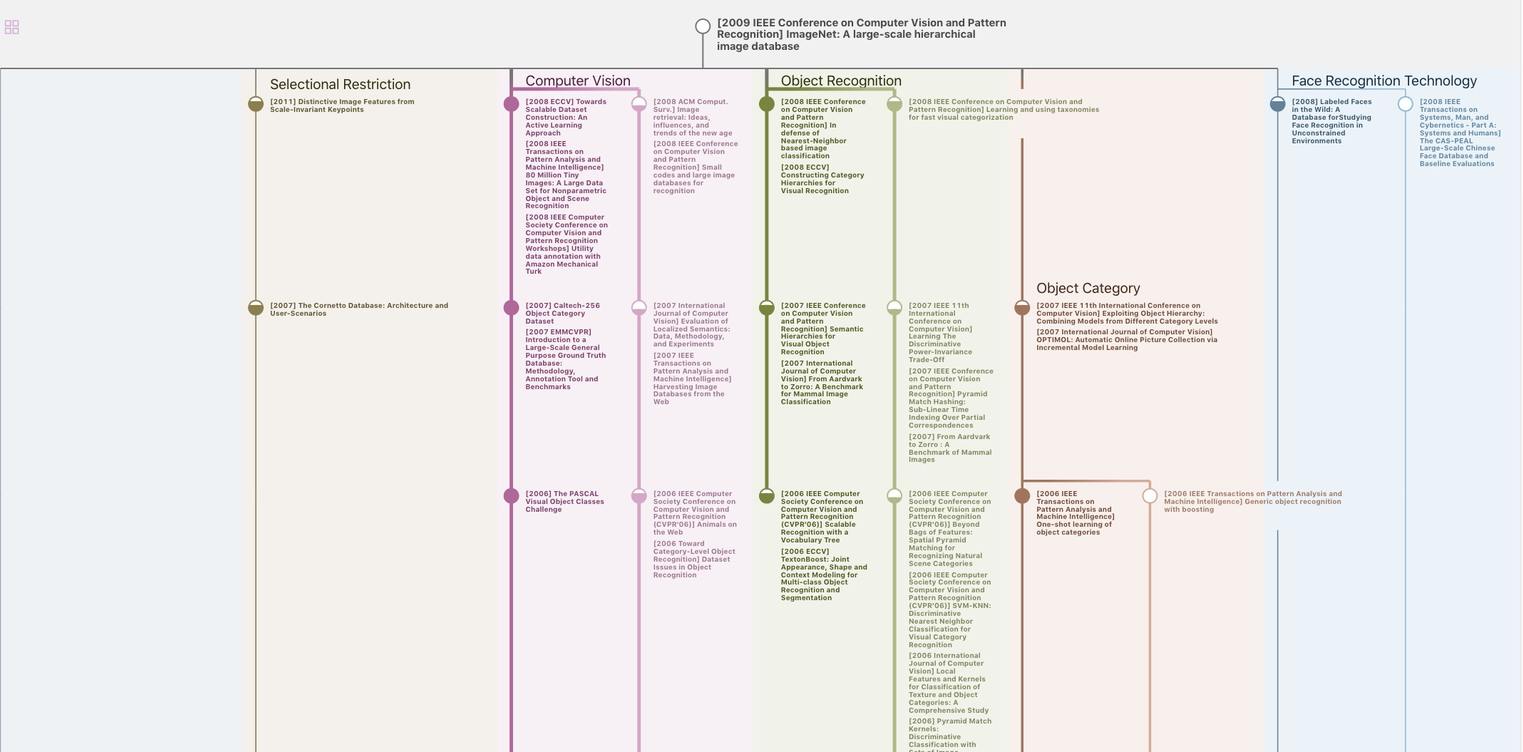
生成溯源树,研究论文发展脉络
Chat Paper
正在生成论文摘要