DDT: Dual-branch Deformable Transformer for Image Denoising
CoRR(2023)
摘要
Transformer is beneficial for image denoising tasks since it can model long-range dependencies to overcome the limitations presented by inductive convolutional biases. However, directly applying the transformer structure to remove noise is challenging because its complexity grows quadratically with the spatial resolution. In this paper, we propose an efficient Dual-branch Deformable Transformer (DDT) denoising network which captures both local and global interactions in parallel. We divide features with a fixed patch size and a fixed number of patches in local and global branches, respectively. In addition, we apply deformable attention operation in both branches, which helps the network focus on more important regions and further reduces computational complexity. We conduct extensive experiments on real-world and synthetic denoising tasks, and the proposed DDT achieves state-of-the-art performance with significantly fewer computational costs.
更多查看译文
关键词
Image denoising, dual-branch, transformer, deformable attention
AI 理解论文
溯源树
样例
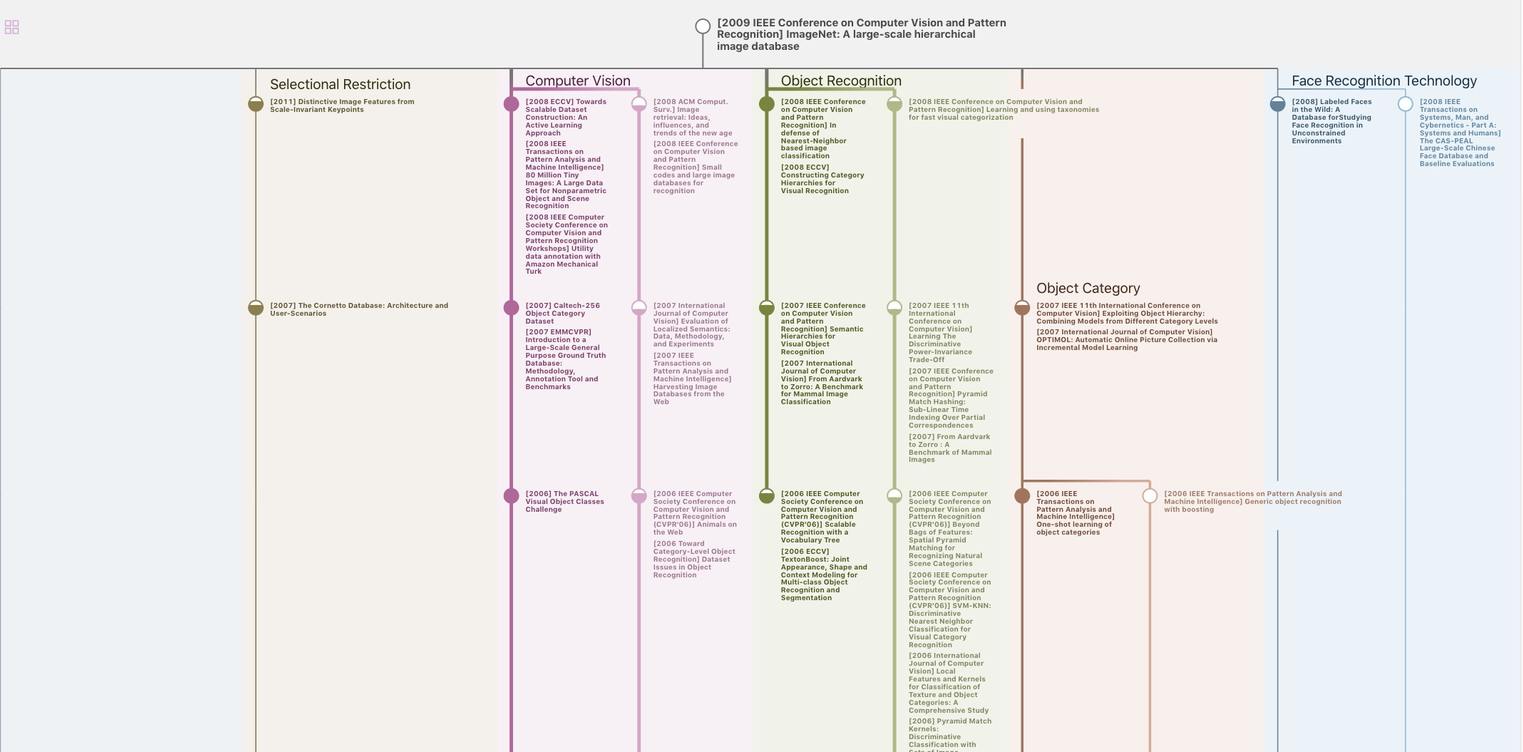
生成溯源树,研究论文发展脉络
Chat Paper
正在生成论文摘要