Quantifying and Explaining Machine Learning Uncertainty in Predictive Process Monitoring: An Operations Research Perspective
CoRR(2023)
摘要
This paper introduces a comprehensive, multi-stage machine learning methodology that effectively integrates information systems and artificial intelligence to enhance decision-making processes within the domain of operations research. The proposed framework adeptly addresses common limitations of existing solutions, such as the neglect of data-driven estimation for vital production parameters, exclusive generation of point forecasts without considering model uncertainty, and lacking explanations regarding the sources of such uncertainty. Our approach employs Quantile Regression Forests for generating interval predictions, alongside both local and global variants of SHapley Additive Explanations for the examined predictive process monitoring problem. The practical applicability of the proposed methodology is substantiated through a real-world production planning case study, emphasizing the potential of prescriptive analytics in refining decision-making procedures. This paper accentuates the imperative of addressing these challenges to fully harness the extensive and rich data resources accessible for well-informed decision-making.
更多查看译文
关键词
machine learning uncertainty,process monitoring,machine learning
AI 理解论文
溯源树
样例
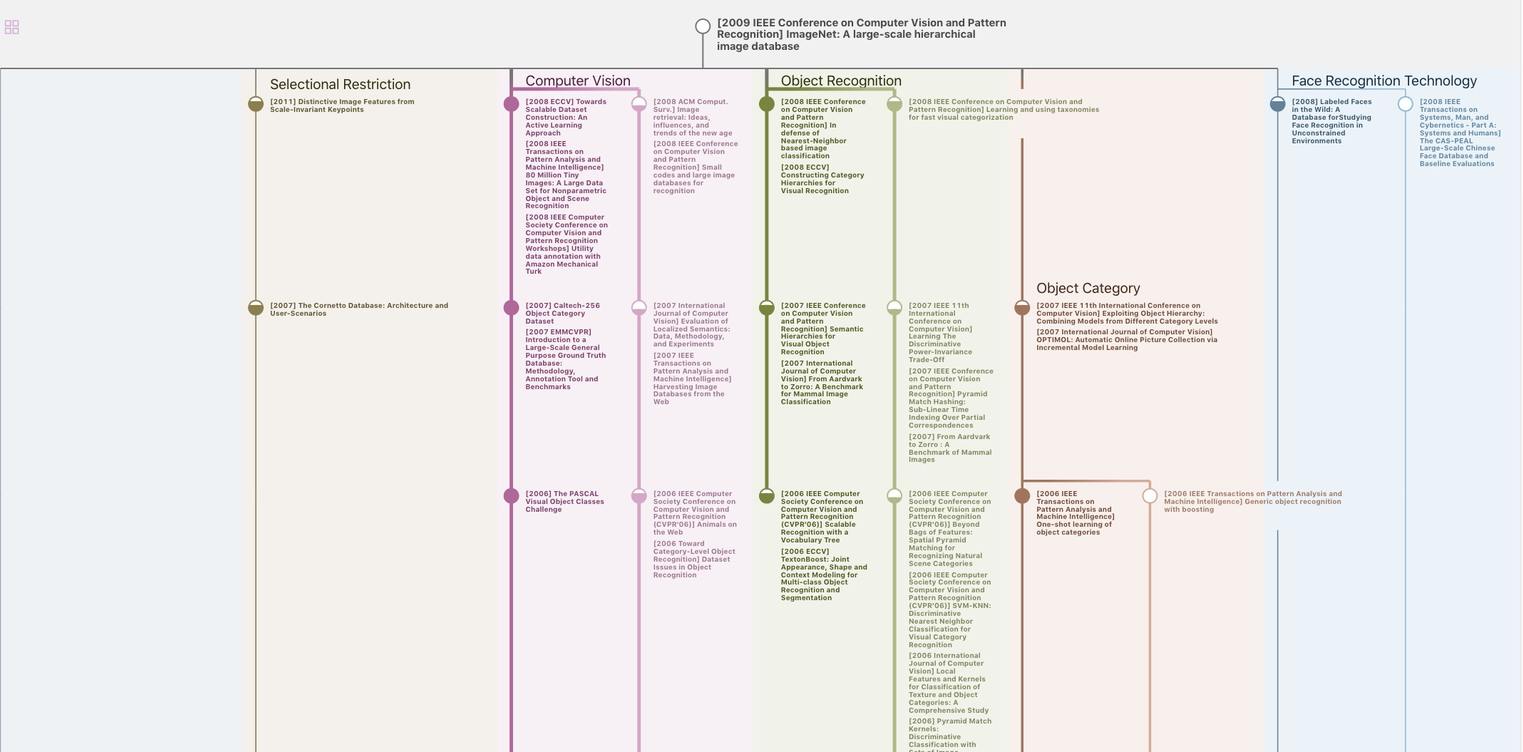
生成溯源树,研究论文发展脉络
Chat Paper
正在生成论文摘要