DGA Based Ensemble learning and Random Forest Models for Condition Assessment of Transformers
2022 International Conference on Smart Generation Computing, Communication and Networking (SMART GENCON)(2022)
摘要
One of the most common and significant components in the energy sector is power transformers. In a transformer, we use oil which has four applications as electric insulation medium, cooling objective, diagnostic goal, and defense against chemical attack. Transformer's dissolved gas analysis (D.G.A.) is the most extensively used technique that achieves the diagnostic goal of identifying early insulation issues. However, the DGA fault interpretation using processing methods has significant obstacles due to the minimal data availability, considerable vagueness in DGA interpretation, applicability, and model performance. It is necessary to comprehend the impact of data preparation and databalancing on the DGA database as a potential issue to upgrade the current model to the industry. This work uses vast, extremely diverse DGA data samples of in-service transformers from several sites in Himachal Pradesh, India, to create a practical methodology for fault classification. In this condition interpretation work, we analyze various parameters of DGA outputs and assess the state of the oil. A total of 421 DGA test databases were collected, and we trained the various algorithmic models in machine learning to predict the condition of oil. The best model's hyper-parameters are further tweaked for the most accurate fault categorization.
更多查看译文
关键词
Transformer,Machine Learning (ML),Diagnostic Test (DT),Dissolved gas analysis (DGA),etc
AI 理解论文
溯源树
样例
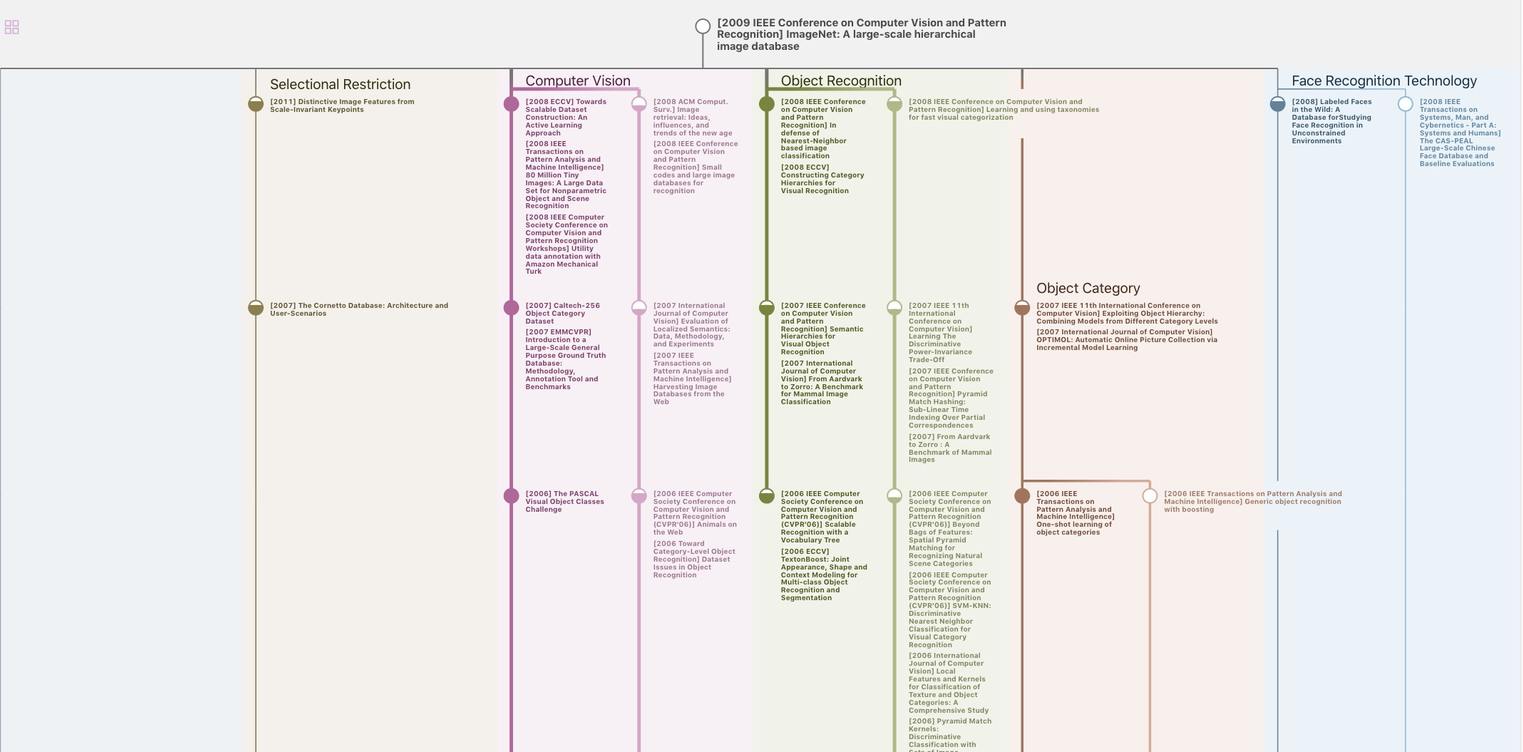
生成溯源树,研究论文发展脉络
Chat Paper
正在生成论文摘要