Distributed Policy Gradient with Heterogeneous Computations for Federated Reinforcement Learning
2023 57th Annual Conference on Information Sciences and Systems (CISS)(2023)
摘要
The rapid advances in federated learning (FL) in the past few years have recently inspired federated reinforcement learning (FRL), where multiple reinforcement learning (RL) agents collaboratively learn a common decision-making policy without exchanging their raw interaction data with their environments. In this paper, we consider a general FRL framework where agents interact with different environments with identical state and action spaces but different rewards and dynamics. Motivated by the fact that agents often have heterogeneous computation capabilities, we propose a Federated Heterogeneous Policy Gradient (FedHPG) algorithm for FRL, where agents can use different numbers of data trajectories (i.e., batch sizes) and different numbers of local computation iterations for their respective PG algorithms. We characterize performance bounds for the learning accuracy of FedHPG, which shows that it achieves a learning accuracy ∊ with sample complexity of
$O$
(1/∊
2
), which matches the performance of existing RL algorithms. The results also show the impacts of local iteration numbers and batch sizes for iteration on the learning accuracy. We also extend FedHPG to heterogeneous policy gradient variance reduction (FedHPGVR) algorithm based on the variance reduction method, and analyze the convergence of this algorithm. The theoretical results are verified empirically for benchmark RL tasks.
更多查看译文
AI 理解论文
溯源树
样例
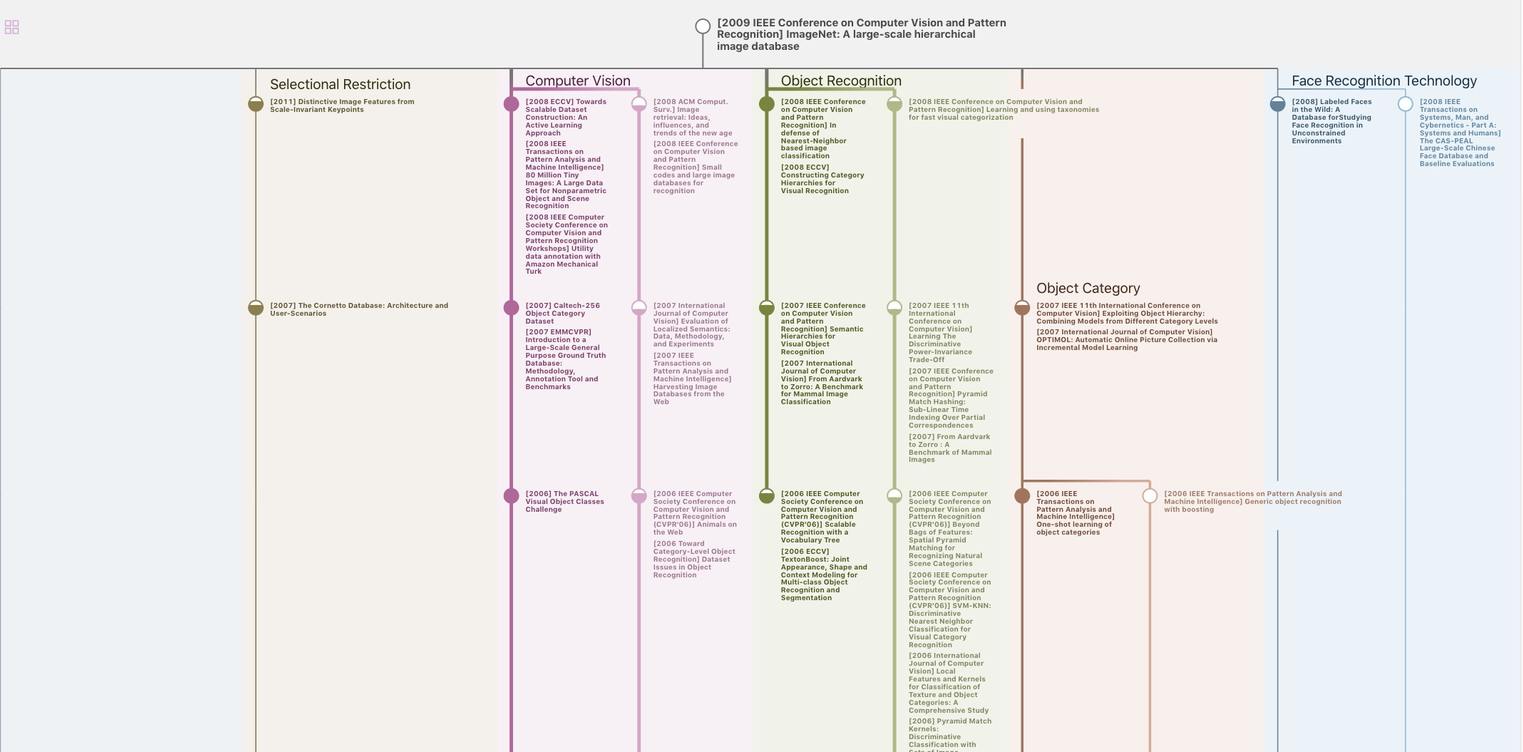
生成溯源树,研究论文发展脉络
Chat Paper
正在生成论文摘要