Improving Particle Thompson Sampling through Regenerative Particles
2023 57th Annual Conference on Information Sciences and Systems (CISS)(2023)
摘要
This paper proposes regenerative particle Thompson sampling (RPTS) as an improvement of particle Thompson sampling (PTS) for solving general stochastic bandit problems. PTS approximates Thompson sampling by replacing the continuous posterior distribution with a discrete distribution supported at a set of weighted static particles. PTS is flexible but may suffer from poor performance due to the tendency of the probability mass to concentrate on a small number of particles. RPTS exploits the particle weight dynamics of PTS and uses non-static particles: it deletes a particle if its probability mass gets sufficiently small and regenerates new particles in the vicinity of the surviving particles. Empirical evidence shows uniform improvement across a set of representative bandit problems without increasing the number of particles.
更多查看译文
关键词
stochastic bandit,Thompson sampling,particles
AI 理解论文
溯源树
样例
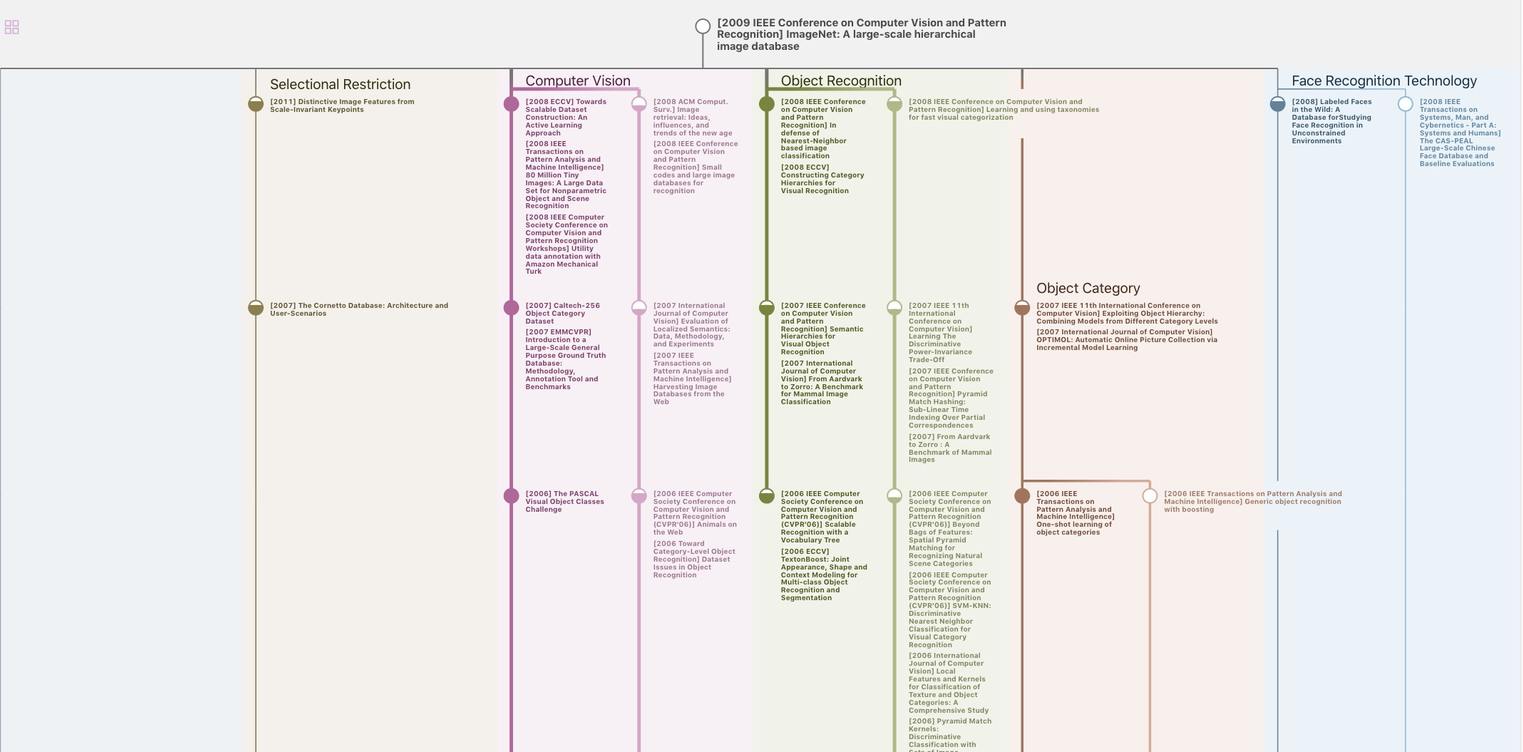
生成溯源树,研究论文发展脉络
Chat Paper
正在生成论文摘要