Scalable Undersized Dataset RF Classification: Using convolutional multistage training
IEEE Antennas and Propagation Magazine(2023)
摘要
Unlike areas such as computer vision and speech recognition, where convolutional and recurrent neural network (NN)-based approaches have proven effective due to the nature of the respective areas of application, deep learning (DL) still lacks a general approach suitable for the unique nature and challenges of radiofrequency (RF) systems such as radar, signals intelligence, electronic warfare, and communications. Existing approaches face problems in robustness, consistency, efficiency, repeatability, and scalability. Some of the main challenges in radiofrequency (RF) sensing such as radar target identification are the difficulty and cost of obtaining data. Hundreds to thousands of samples per class are typically used when training for classifying signals into 2–12 classes with reported accuracy ranging from 87% to 99%, where accuracy generally decreases with more classes added. In this article, we present a new DL approach based on multistage training (MST) and demonstrate it on RF sensing signal classification. We consistently achieve more than 99% accuracy for up to 17 diverse classes using only 11 samples per class for training, a substantial improvement over standard DL approaches.
更多查看译文
关键词
Feature extraction, Training, Radio frequency, Convolution, Radar, Artificial neural networks, Standards
AI 理解论文
溯源树
样例
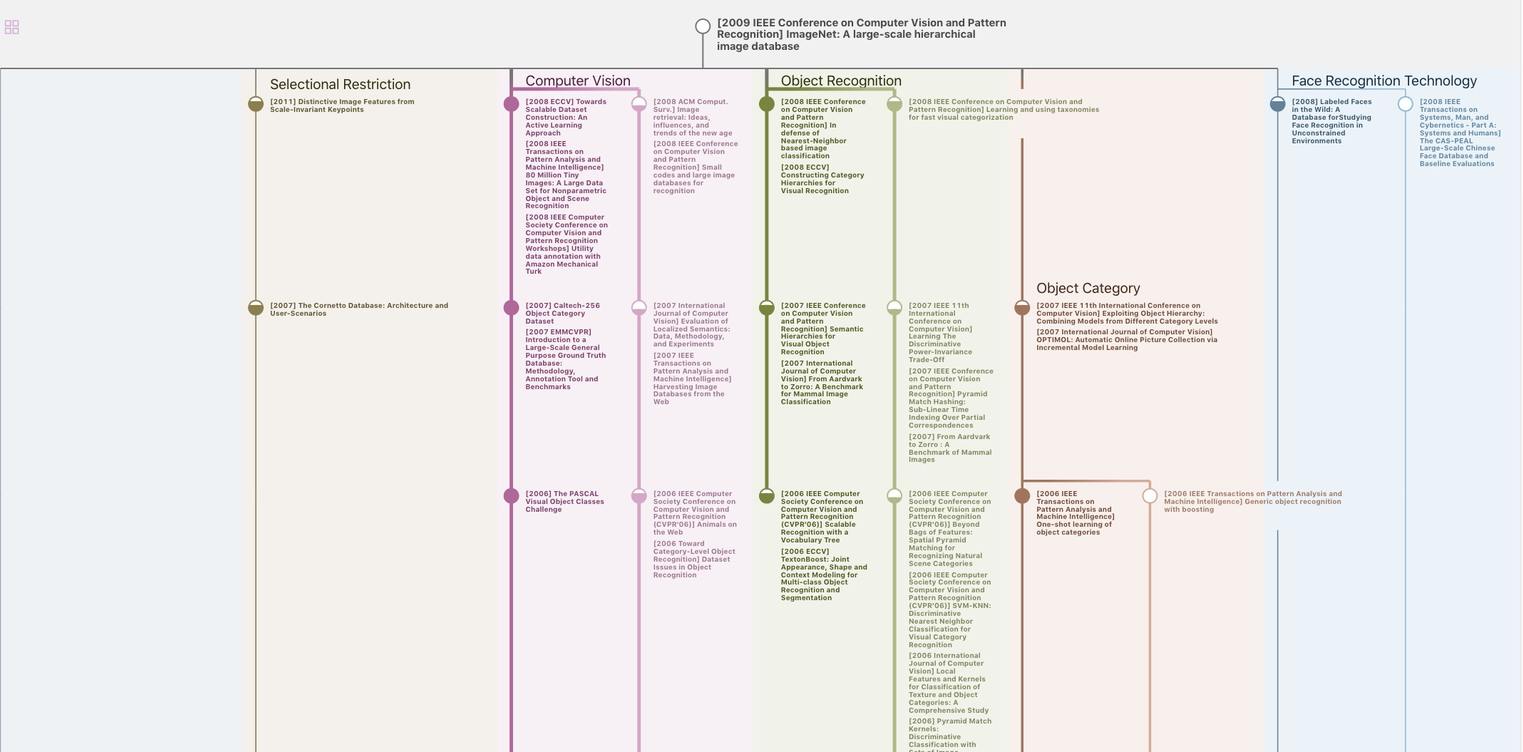
生成溯源树,研究论文发展脉络
Chat Paper
正在生成论文摘要