Towards Interpretable Probabilistic Classification Models for Knowledge Graphs
2022 16th International Conference on Signal-Image Technology & Internet-Based Systems (SITIS)(2022)
摘要
Tackling the problem of learning probabilistic classifiers that can be used the context of knowledge graphs, we describe an inductive approach based on learning networks of Bernoulli variables. Namely, we consider the application of multivariate Bernoulli models, a simple one and a two-levels mixture model. In addition, we also consider a hierarchical model combining the multivariate Bernoulli model with a restricted Boltzmann machine as the first level. We show how such models can be converted into probabilistic rule bases ensuring more understandability. A preliminary empirical evaluation is presented to test the effectiveness of these models on a number of classification problems with different knowledge graphs.
更多查看译文
关键词
knowledge graph,semantic web,probabilistic model,rule base,classification,interpretability,XAI
AI 理解论文
溯源树
样例
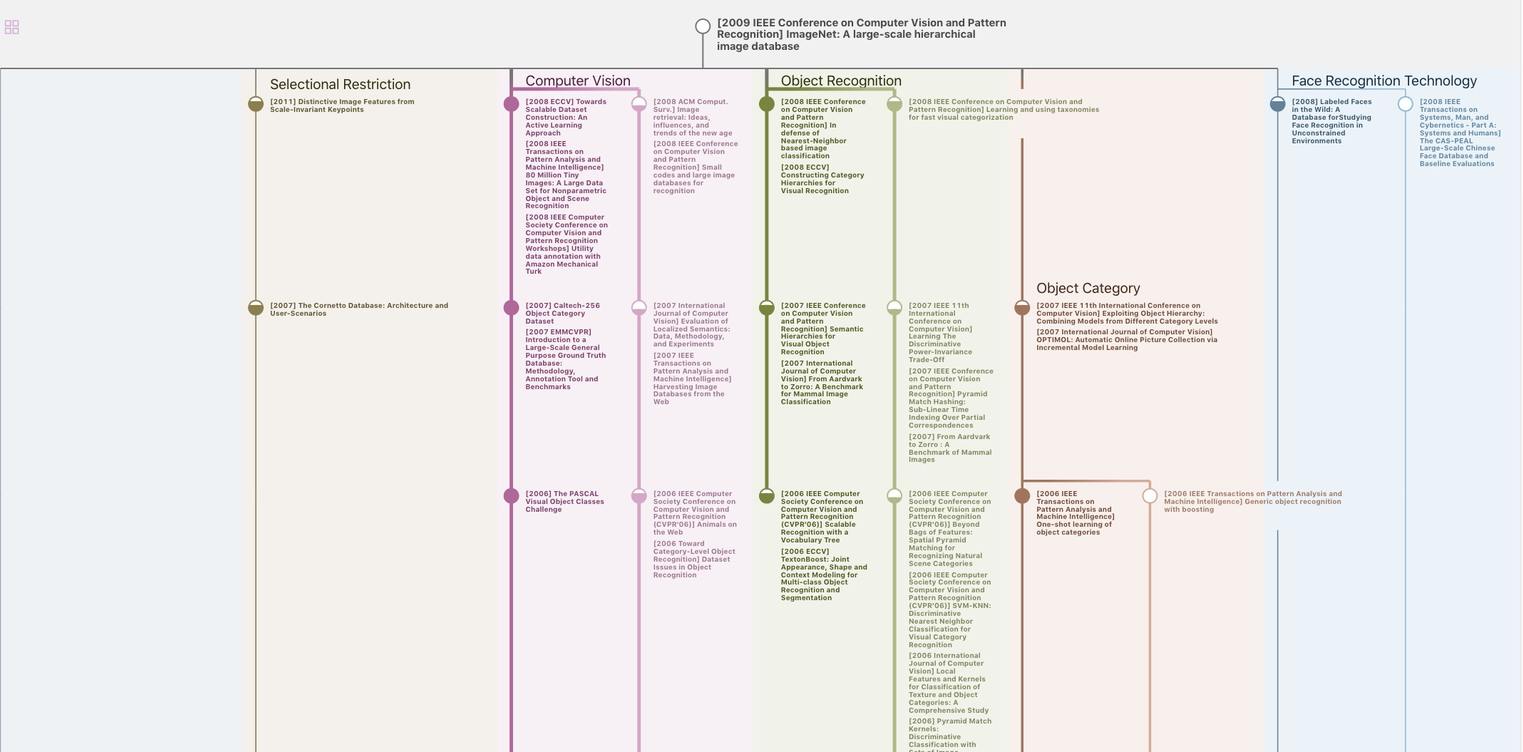
生成溯源树,研究论文发展脉络
Chat Paper
正在生成论文摘要