Tomographic reconstruction from sparse-view and limited-angle data using a generative adversarial network
2022 16th International Conference on Signal-Image Technology & Internet-Based Systems (SITIS)(2022)
摘要
Limited-angle and sparse-view computed tomography have been widely used to shorten the acquisition time in medical imaging and to offer the possibility of scanning large objects. However, this is a severely ill-posed inverse problem due to missing data. In these scenarios, the well-known filtered back-projection reconstruction technique exhibits severe artifacts and degradation. Recently, deep learning methods have demonstrated impressive performance in computer vision (denoising, classification, etc.) but it frequently fails to solve both limited-angle and sparse-view reconstruction. Inspired by the high performance of GAN-based image-to-image translation methods, we investigate a patchGAN as a solution to the reconstruction problem mapping data (Radon space) into the image domain. The generator is made of a V-net where the reconstruction in the sense of a least-squares minimization is carried out at different scales in the encoder path and linked with the decoder path by skip connections. The discriminator uses both information from the image and projection data domains. The proposed method gives promising reconstruction results from data acquired with a limited angular range covering only 110 degrees (instead of 180 degrees), as well as for sparse-view data with 10 degrees of sampling step. Moreover, different reconstruction results show that the method is able to reconstruct images from sparse and limited angular range data at the same time.
更多查看译文
关键词
computed tomography (CT),generative adversarial networks (GAN),image reconstruction,inverse problem,hybrid representations
AI 理解论文
溯源树
样例
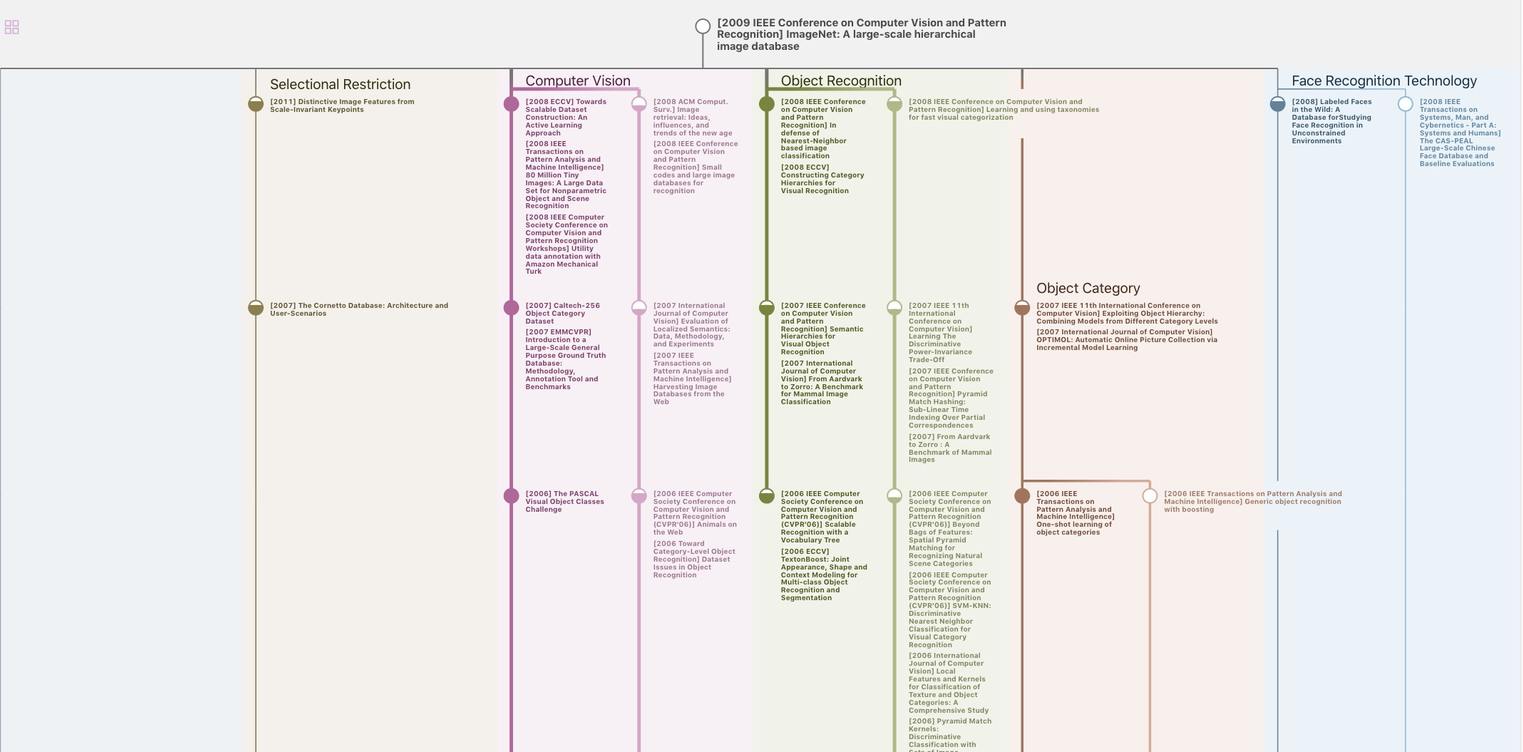
生成溯源树,研究论文发展脉络
Chat Paper
正在生成论文摘要