Generation of Synthetic CT with Deep Learning for Magnetic Resonance Guided Radiotherapy
2022 16TH INTERNATIONAL CONFERENCE ON SIGNAL-IMAGE TECHNOLOGY & INTERNET-BASED SYSTEMS, SITIS(2022)
摘要
Magnetic Resonance guided Radiotherapy (MRgRT) still requires the acquisition of Computer Tomography (CT) images which provide the electronic density information necessary for Radiotherapy (RT) dose planning. Within this workflow, the CT image is registered to the Magnetic Resonance (MR) image in a process that could introduce systematic errors to the dose estimation. The use of synthetic CT (sCT) images generated from MR images could prevent the need of a CT acquisition and further image registration. In this work we propose the use of U-Net and GAN architectures for the generation of synthetic CT images for abdominal radiotherapy with low-field MRI. A deep learning based bulk density approach was also explored where U-Net and GAN networks were used to generate sCT images comprised of 6 different densities only. Evaluation of this methods was measured in terms of image and dose metrics considering the Mean Error, Mean Absolute Error and Gamma Pass Rate with respect to the original CT image and CT-based dose plans. A high dose affinity was achieved with gamma pass rates of 95% for the 3%/3mm criteria. Whole patient sCT image generation was accomplished in 2 seconds for the U-Net and 2.5 seconds with the GAN architecture. The proposed techniques performed within the clinically acceptable criteria with a fast image generation and high dose similarity.
更多查看译文
关键词
Radiotherapy,MRI,sCT,Deep Learning
AI 理解论文
溯源树
样例
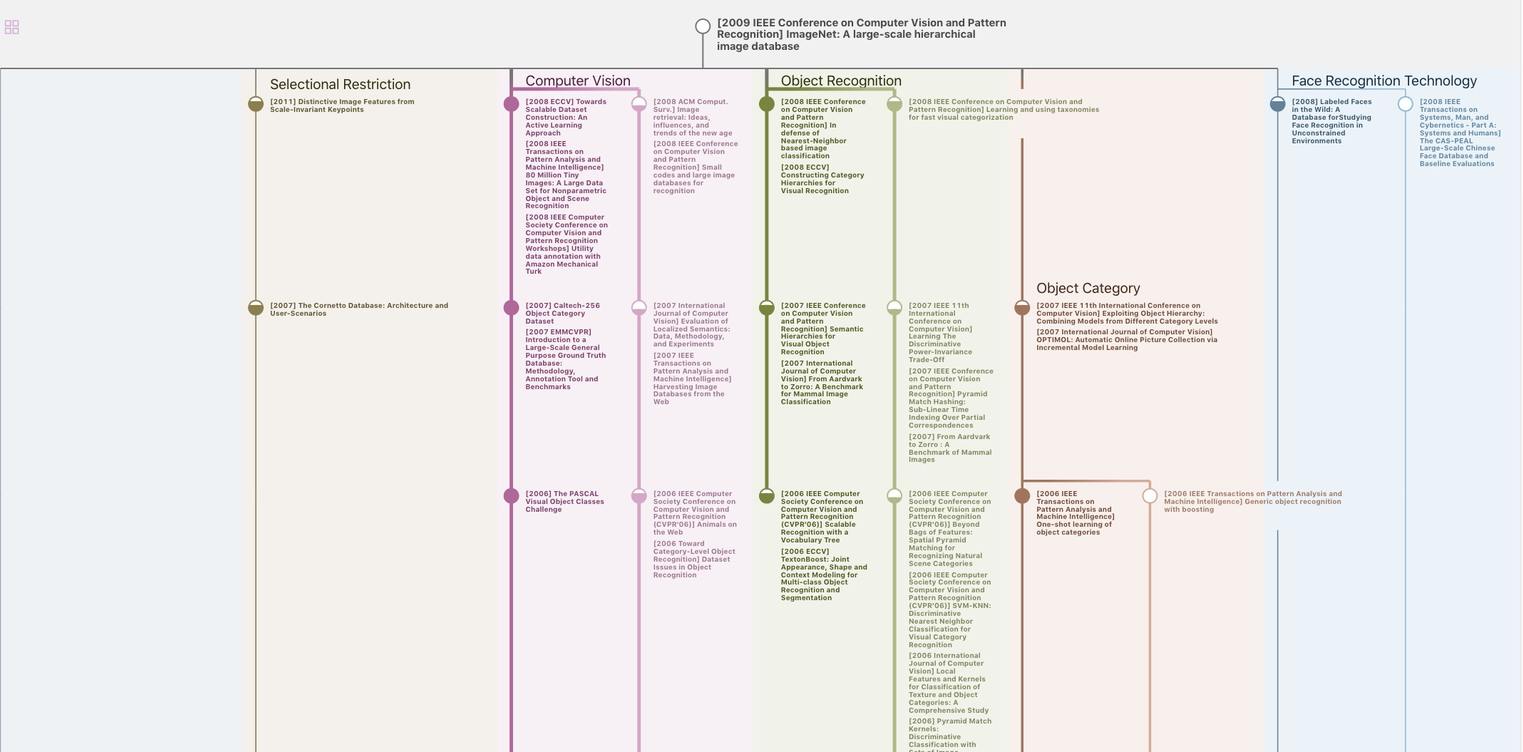
生成溯源树,研究论文发展脉络
Chat Paper
正在生成论文摘要